BM-SMIL: A Breast Cancer Molecular Subtype Prediction Framework from H&E Slides with Self-supervised Pretraining and Multi-instance Learning
COMPUTATIONAL MATHEMATICS MODELING IN CANCER ANALYSIS, CMMCA 2023(2023)
摘要
Breast cancer is the most commonly diagnosed cancer, and accurate molecular subtype prediction plays a crucial role in determining treatment strategies. While immunohistochemistry (IHC) is commonly used for molecular subtype diagnosis, it suffers from cost and labor limitations. The prediction of molecular subtypes using hematoxylin and eosin (H&E) stained slides has gained importance. However, the task is challenged by limited samples, weak annotations, and strong tumor heterogeneity. This paper proposes a scalable framework, BM-SMIL, for molecular subtype prediction of breast cancer based on self-supervised pre-training and multi-instance learning. Firstly, a self-supervised pretraining framework utilizing multi-scale knowledge distillation is introduced to obtain a representative patch encoder. Then, an attention-based instance selection strategy is employed to filter out noise instances. Finally, a Transformer integrated with subtype contrastive loss is proposed for effective aggregation and WSI-level prediction. Experimental results on the dataset from cooperative hospital demonstrate the effectiveness of our proposed framework. The BM-SMIL framework has the potential to enhance molecular subtype prediction performance and can be extended to other pathology image classification tasks.
更多查看译文
关键词
Molecular Subtype,H&E Slides,Self-supervised Pretraining,MIL
AI 理解论文
溯源树
样例
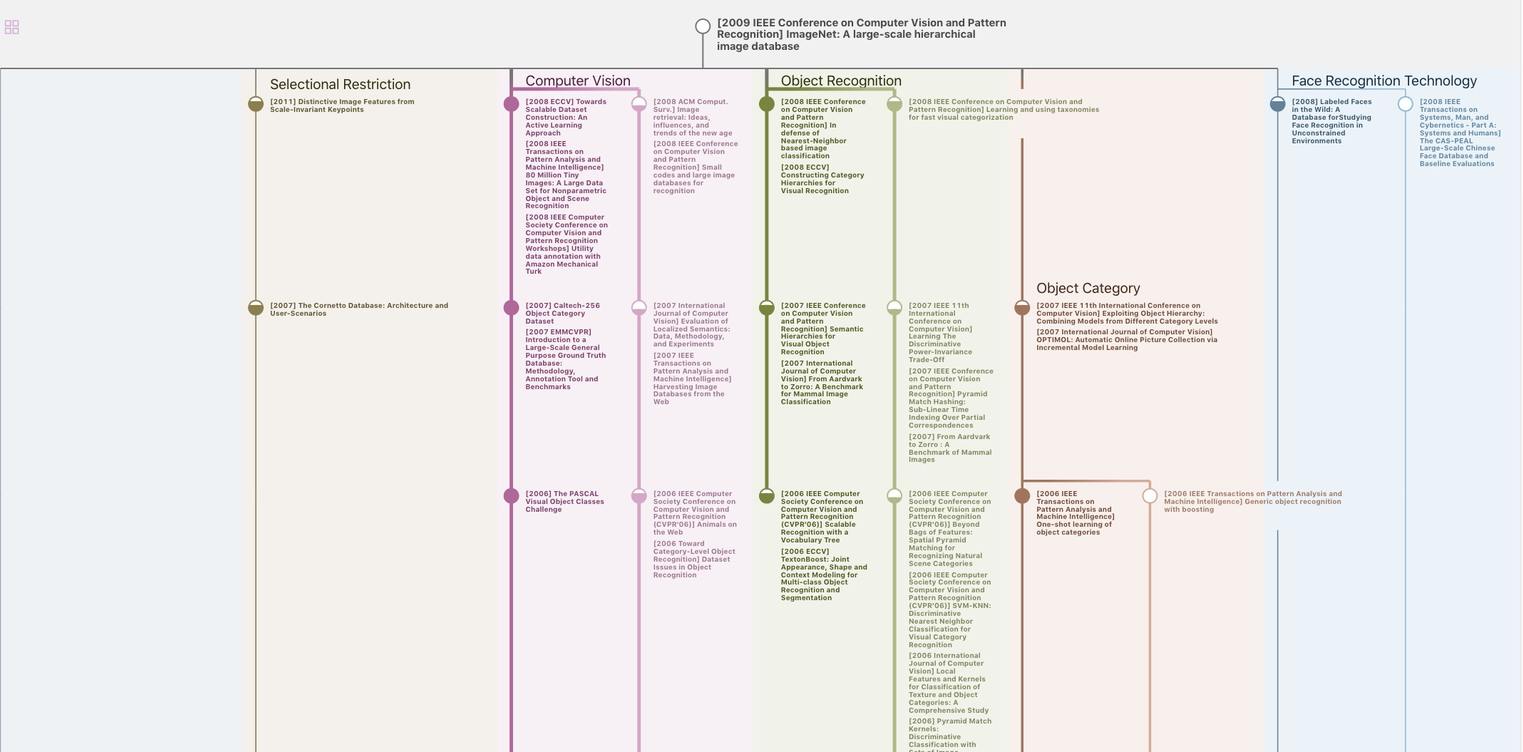
生成溯源树,研究论文发展脉络
Chat Paper
正在生成论文摘要