DiMix: Disentangle-and-Mix Based Domain Generalizable Medical Image Segmentation
MEDICAL IMAGE COMPUTING AND COMPUTER ASSISTED INTERVENTION, MICCAI 2023, PT III(2023)
摘要
The rapid advancements in deep learning have revolutionized multiple domains, yet the significant challenge lies in effectively applying this technology to novel and unfamiliar environments, particularly in specialized and costly fields like medicine. Recent deep learning research has therefore focused on domain generalization, aiming to train models that can perform well on datasets from unseen environments. This paper introduces a novel framework that enhances generalizability by leveraging transformer-based disentanglement learning and style mixing. Our framework identifies features that are invariant across different domains. Through a combination of content-style disentanglement and image synthesis, the proposed method effectively learns to distinguish domain-agnostic features, resulting in improved performance when applied to unseen target domains. To validate the effectiveness of the framework, experiments were conducted on a publicly available Fundus dataset, and comparative analyses were performed against other existing approaches. The results demonstrated the power and efficacy of the proposed framework, showcasing its ability to enhance domain generalization performance.
更多查看译文
关键词
Domain generalization,Medical image segmentation,Disentanglement,Transformers
AI 理解论文
溯源树
样例
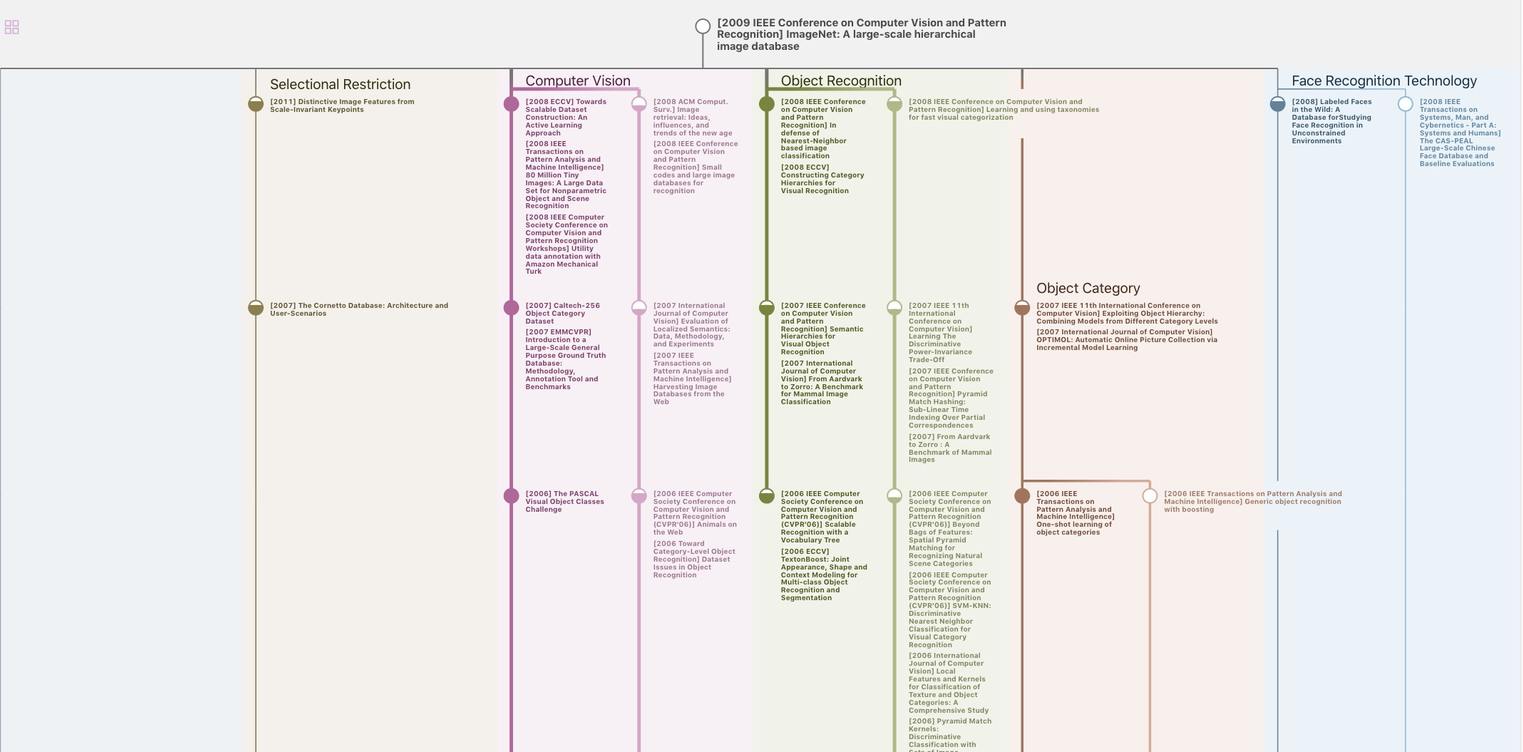
生成溯源树,研究论文发展脉络
Chat Paper
正在生成论文摘要