Realistic Endoscopic Illumination Modeling for NeRF-Based Data Generation
MEDICAL IMAGE COMPUTING AND COMPUTER ASSISTED INTERVENTION, MICCAI 2023, PT IX(2023)
摘要
Expanding training and evaluation data is a major step towards building and deploying reliable localization and 3D reconstruction techniques during colonoscopy screenings. However, training and evaluating pose and depth models in colonoscopy is hard as available datasets are limited in size. This paper proposes a method for generating new pose and depth datasets by fitting NeRFs in already available colonoscopy datasets. Given a set of images, their associated depth maps and pose information, we train a novel light source location-conditioned NeRF to encapsulate the 3D and color information of a colon sequence. Then, we leverage the trained networks to render images from previously unobserved camera poses and simulate different camera systems, effectively expanding the source dataset. Our experiments show that our model is able to generate RGB images and depth maps of a colonoscopy sequence from previously unobserved poses with high accuracy. Code and trained networks can be accessed at https://github.com/surgical-vision/ REIM-NeRF.
更多查看译文
关键词
Surgical Data Science,Surgical AI,Data generation,Neural Rendering,Colonoscopy
AI 理解论文
溯源树
样例
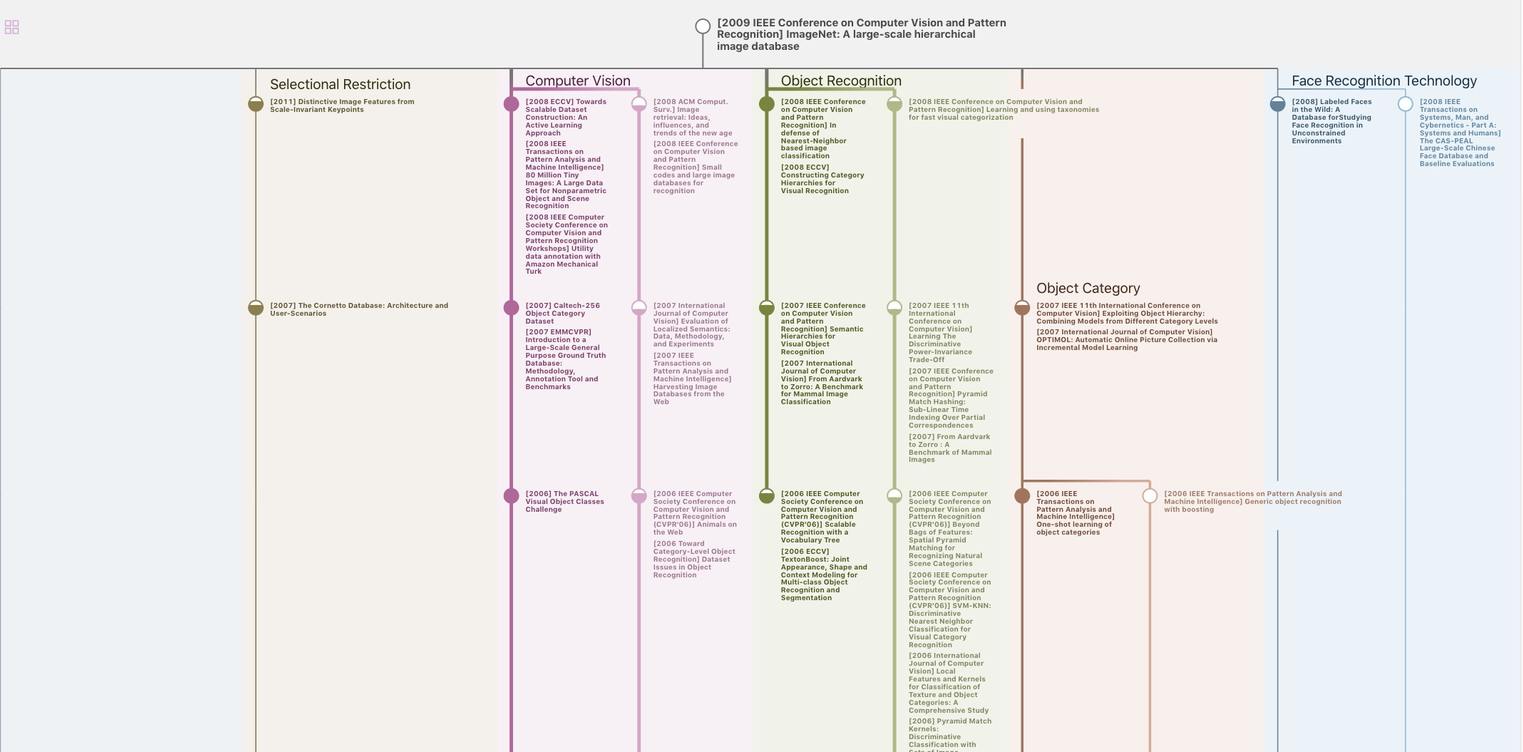
生成溯源树,研究论文发展脉络
Chat Paper
正在生成论文摘要