Deep Survival Analysis in Multiple Sclerosis
PREDICTIVE INTELLIGENCE IN MEDICINE, PRIME 2023(2023)
摘要
Multiple Sclerosis (MS) is the most frequent non-traumatic debilitating neurological disease. It is usually diagnosed based on clinical observations and supporting data from auxiliary procedures. However, its course is extremely unpredictable, and traditional statistical survival models fail to perform reliably on longitudinal data. An efficient and precise prognosis model of patient-specific MS time-to-event distributions is needed to aid in joint decision-making in subsequent treatment and care. In this work, we aim to estimate the survival function to predict MS disability progression based on discrete longitudinal reaction time trajectories and related clinical variables. To this end, we initially preprocess two sets of measurements obtained from the same cohort of patients. One set comprises the patients' reaction trajectories recorded during computerized tests, while the other set involves assessing their disability progression and extracting practical clinical information. Then we propose our deep survival model for discovering the connections between temporal data and the potential risk. The model is optimised over the sum of three losses, including longitudinal loss, survival loss and consistent loss. We evaluate our model against other machine learning methods on the same dataset. The experimental results demonstrate the advantage of our proposed deep learning model and prove that such computerized measurements can genuinely reflect the disease stage of MS patients and provide a second opinion for prognosticating their disability progression.
更多查看译文
关键词
Survival analysis,Multiple sclerosis,Disability progression,temporal
AI 理解论文
溯源树
样例
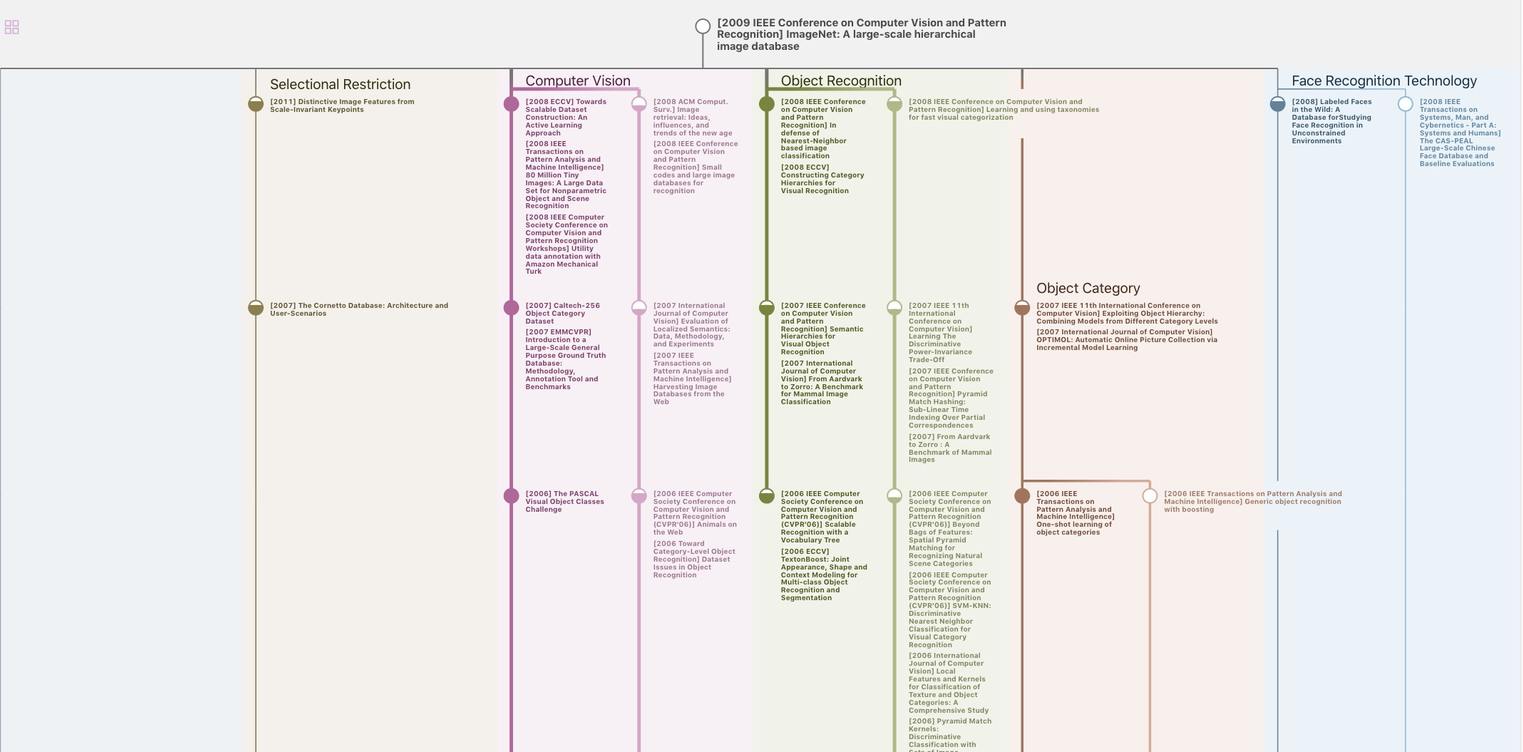
生成溯源树,研究论文发展脉络
Chat Paper
正在生成论文摘要