Building a Bridge: Close the Domain Gap in CT Metal Artifact Reduction
MEDICAL IMAGE COMPUTING AND COMPUTER ASSISTED INTERVENTION, MICCAI 2023, PT X(2023)
摘要
Metal artifacts in computed tomography (CT) degrade the imaging quality, leading to a negative impact on the clinical diagnosis. Empowered by medical big data, many DL-based approaches have been proposed for metal artifact reduction (MAR). In supervised MAR methods, models are usually trained on simulated data and then applied to the clinical data. However, inferior MAR performance on clinical data is usually observed due to the domain gap between simulated and clinical data. Existing unsupervised MAR methods usually use clinical unpaired data for training, which often distort the anatomical structure due to the absence of supervision information. To address these problems, we propose a novel semi-supervised MAR framework. The clean image is employed as the bridge between the synthetic and clinical metal-affected image domains to close the domain gap. We also break the cycle-consistency loss, which is often utilized for domain transformation, since the bijective assumption is too harsh to accurately respond to the facts of real situations. To further improve the MAR performance, we propose a new Artifact Filtering Module (AFM) to eliminate features helpless in recovering clean images. Experiments demonstrate that the performance of the proposed method is competitive with several state-of-the-art unsupervised and semi-supervised MAR methods in both qualitative and quantitative aspects.
更多查看译文
关键词
Computed Tomography,Metal Artifact Reduction,Deep Learning,Domain Gap
AI 理解论文
溯源树
样例
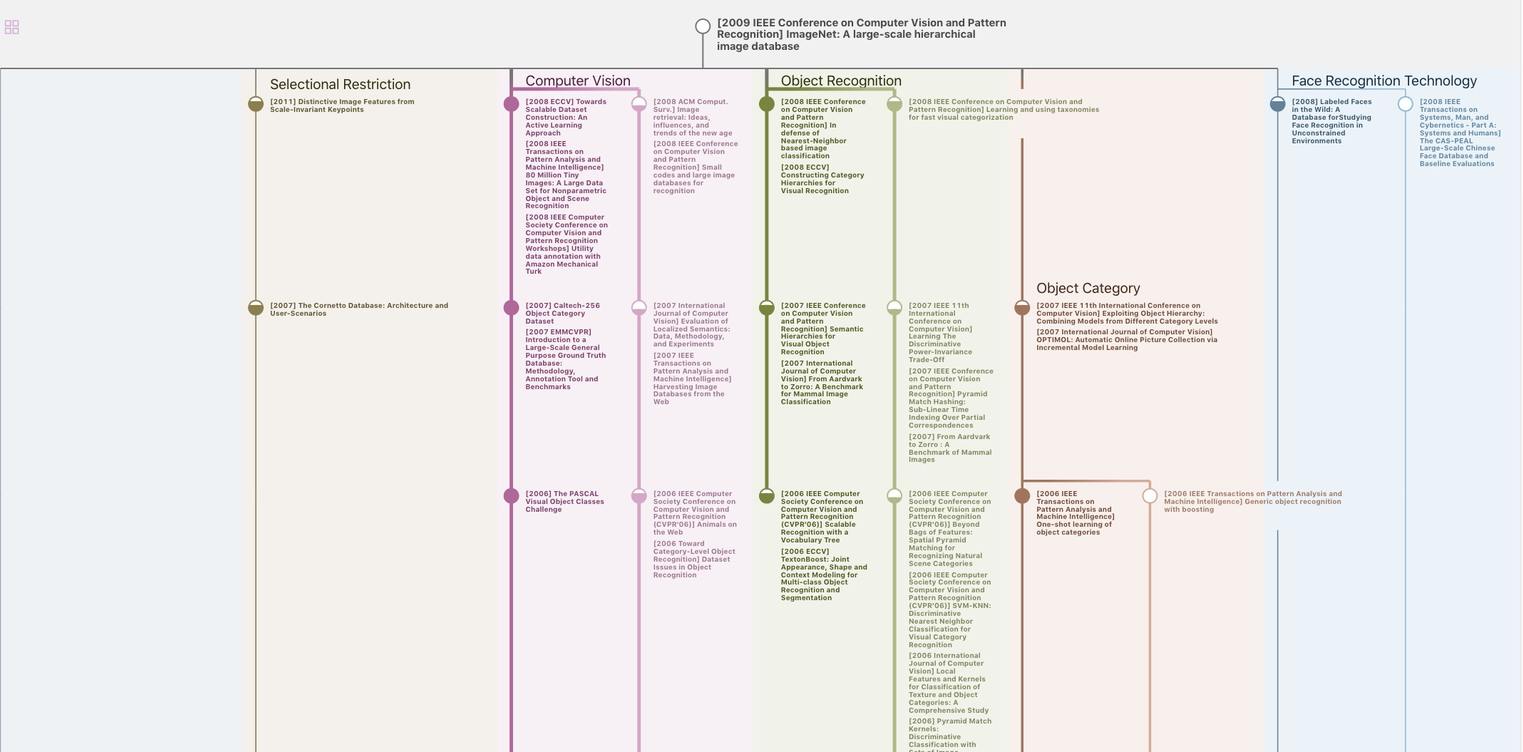
生成溯源树,研究论文发展脉络
Chat Paper
正在生成论文摘要