Learning Robust Classifier for Imbalanced Medical Image Dataset with Noisy Labels by Minimizing Invariant Risk
MEDICAL IMAGE COMPUTING AND COMPUTER ASSISTED INTERVENTION, MICCAI 2023, PT VI(2023)
摘要
In medical image analysis, imbalanced noisy dataset classification poses a long-standing and critical problem since clinical large-scale datasets often attain noisy labels and imbalanced distributions through annotation and collection. Current approaches addressing noisy labels and long-tailed distributions separately may negatively impact real-world practices. Additionally, the factor of class hardness hindering label noise removal remains undiscovered, causing a critical necessity for an approach to enhance the classification performance of noisy imbalanced medical datasets with various class hardness. To address this paradox, we propose a robust classifier that trains on a multi-stage noise removal framework, which jointly rectifies the adverse effects of label noise, imbalanced distribution, and class hardness. The proposed noise removal framework consists of multiple phases. Multi-Environment Risk Minimization (MER) strategy captures data-to-label causal features for noise identification, and the Rescaling Class-aware Gaussian Mixture Modeling (RCGM) learns class-invariant detection mappings for noise removal. Extensive experiments on two imbalanced noisy clinical datasets demonstrate the capability and potential of our method for boosting the performance of medical image classification.
更多查看译文
关键词
Imbalanced Data,Noisy Labels,Medical Image Analysis
AI 理解论文
溯源树
样例
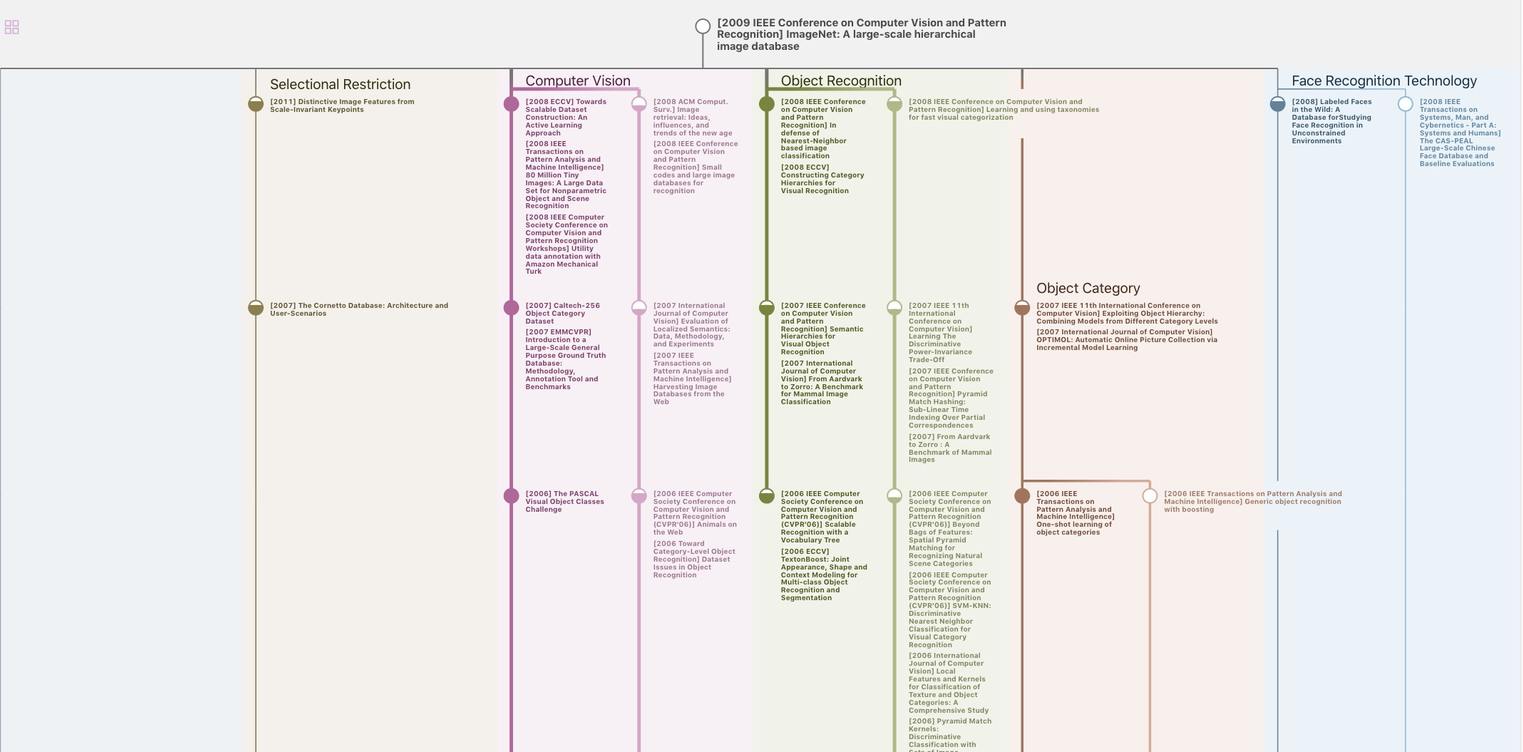
生成溯源树,研究论文发展脉络
Chat Paper
正在生成论文摘要