Meta-Learning with Motif-based Task Augmentation for Few-Shot Molecular Property Prediction.
SDM(2023)
摘要
Predicting molecular properties is known to be a difficult few-shot learning problem in drug discovery due to high failure rates of virtual screening. Meta-learning frameworks have been successfully leveraged to tackle this few-shot molecular property prediction problem. Recent work has shown that the generalization of meta- learning can be further improved by task augmentation techniques. However, it is challenging to directly apply current popular task augmentation strategies to molecular modeling due to its highly discrete nature. To this end, we propose a motif-based task augmentation (MTA) technique to address this challenge. MTA is mainly conducting task augmentations by generating new labeled samples through retrieving highly relevant motifs from a pre-defined motif vocabulary as an external memory. Both support samples and query samples are interpolated with their corresponding retrieved motifs in the latent space in a convex manner parameterized by a specific probability distribution. Augmented query samples are classified by measuring their Euclidean distances to augmented prototype clusters. With our novel MTA approach, the above two overfitting issues can be alleviated through establishing connections between different tasks with augmented motifs. Empirical results for popular benchmark datasets demonstrate that our approach can consistently outperform existing baseline methods and can also provide some interpretable relation information between structural motifs and molecules.Keywordsmeta-learningfew-shot learningmolecular property predictionmotiftask augmentation
更多查看译文
关键词
molecular,task augmentation,meta-learning,motif-based,few-shot
AI 理解论文
溯源树
样例
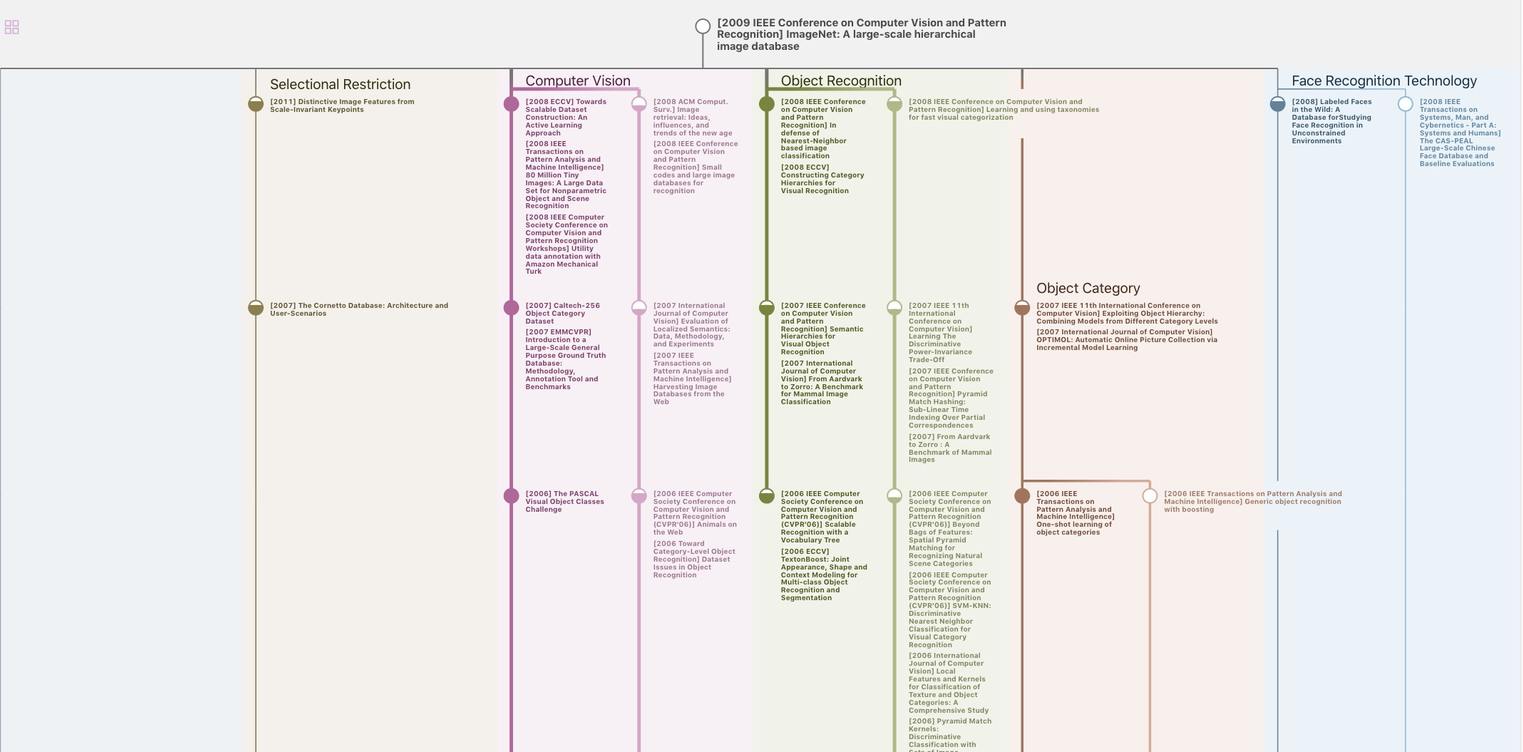
生成溯源树,研究论文发展脉络
Chat Paper
正在生成论文摘要