Beyond The Evidence Lower Bound: Dual Variational Graph Auto-Encoders For Node Clustering.
SDM(2023)
摘要
Variational Graph Auto-Encoders (VGAEs) have achieved promising performance in several applications. Some recent models incorporate the clustering inductive bias by imposing non-Gaussian prior distributions. However, the regularization term is practically insufficient to learn the clustering structures due to the mismatch between the target and the learned distributions. Thus, we formulate a new variational lower bound that incorporates an explicit clustering objective function. The introduction of a clustering objective leads to two problems. First, the latent information destroyed by the clustering process is critical for generating the between-cluster edges. Second, the noisy and sparse input graph does not benefit from the information learned during the clustering process. To address the first problem, we identify a new term overlooked by existing Evidence Lower BOunds (ELBOs). This term accounts for the difference between the variational posterior used for the clustering task and the variational posterior associated with the generation task. Furthermore, we find that the new term increases resistance to posterior collapse. Theoretically, we demonstrate that our lower bound is a tighter approximation of the log-likelihood function. To address the second problem, we propose a graph update algorithm that reduces the over-segmentation and under-segmentation problems. We conduct several experiments to validate the merits of our approach. Our results show that the proposed method considerably improves the clustering quality compared to state-of-the-art VGAE models.
更多查看译文
关键词
graph,auto-encoders
AI 理解论文
溯源树
样例
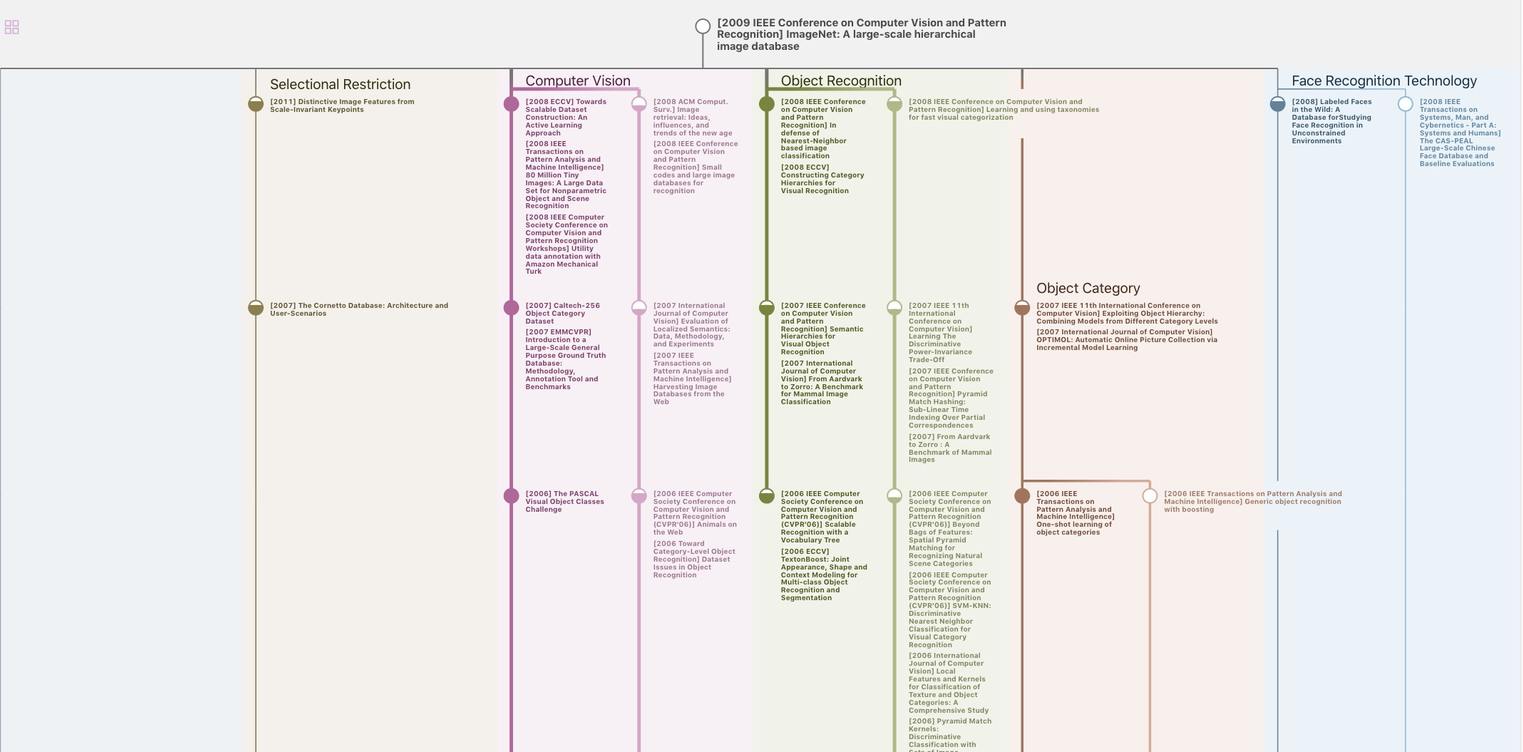
生成溯源树,研究论文发展脉络
Chat Paper
正在生成论文摘要