Taxonomy-Guided Fine-Grained Entity Set Expansion.
SDM(2023)
摘要
Entity set expansion, the task of expanding a small set of similar entities into a much larger set, is a vital step for downstream tasks such as named entity recognition, knowledge base construction and information retrieval. Existing entity set expansion methods were developed by mainly considering entities at coarse-grained levels, which encounter difficulties for entity set expansion at fine-grained levels, due to the subtlety on fine-grained type inference and semantic drifting. In this study, we propose an automated (i.e. without human annotation), fine-grained set expansion framework, FGExpan, which utilizes a taxonomy structure and a pre-trained language model to achieve high performance. To facilitate our testing, a new fine-grained set expansion dataset is also constructed. Experiments on this dataset and those used in previous studies show that FGExpan achieves significantly better performance (MAP up by 0.176) on finegrained types and also the state-of-the-art expansion quality on coarse-grained entity sets.
更多查看译文
关键词
expansion,taxonomy-guided,fine-grained
AI 理解论文
溯源树
样例
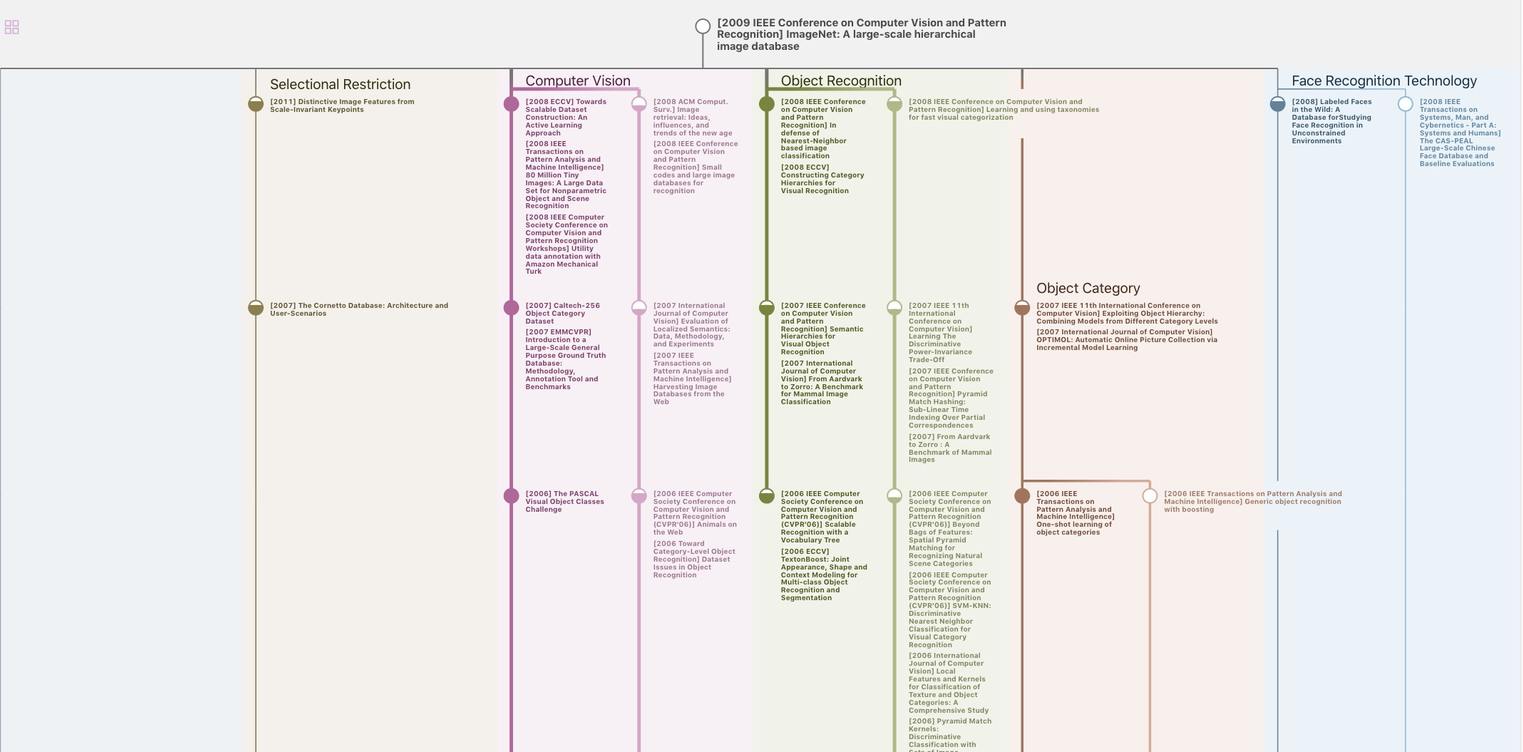
生成溯源树,研究论文发展脉络
Chat Paper
正在生成论文摘要