Self-Supervised Super-Resolution for Anisotropic MR Images with and Without Slice Gap
SIMULATION AND SYNTHESIS IN MEDICAL IMAGING, SASHIMI 2023(2023)
摘要
Magnetic resonance (MR) images are often acquired as multi-slice volumes to reduce scan time and motion artifacts while improving signal-to-noise ratio. These slices often are thicker than their in-plane resolution and sometimes are acquired with gaps between slices. Such thick-slice image volumes (possibly with gaps) can impact the accuracy of volumetric analysis and 3D methods. While many super-resolution (SR) methods have been proposed to address thick slices, few have directly addressed the slice gap scenario. Furthermore, data-driven methods are sensitive to domain shift due to the variability of resolution, contrast in acquisition, pathology, and differences in anatomy. In this work, we propose a self-supervised SR technique to address anisotropic MR images with and without slice gap. We compare against competing methods and validate in both signal recovery and downstream task performance on two open-source datasets and show improvements in all respects. Our code publicly available at https://gitlab.com/iacl/smore.
更多查看译文
关键词
super-resolution,MRI,self-supervised,deep learning
AI 理解论文
溯源树
样例
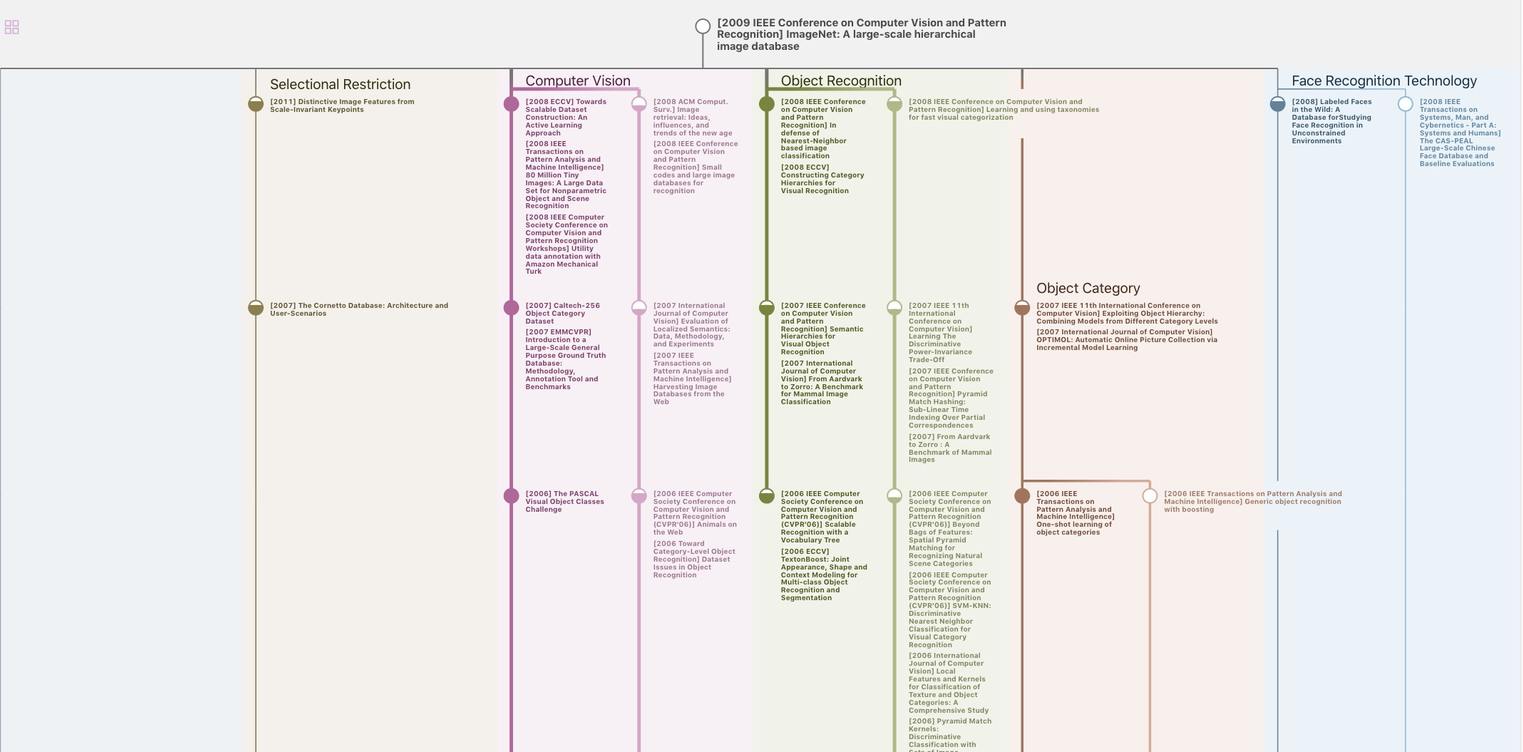
生成溯源树,研究论文发展脉络
Chat Paper
正在生成论文摘要