CADENCE: Community-Aware Detection of Dynamic Network States.
SDM(2023)
摘要
Dynamic interaction data is often aggregated in a sequence of network snapshots before being employed in downstream analysis. The two common ways of defining network snapshots are i) a fixed time interval or ii) fixed number of interactions per snapshot. The choice of aggregation has a significant impact on subsequent analysis, and it is not trivial to select one approach over another for a given dataset. More importantly assuming snapshot regularity is data-agnostic and may be at odds with the underlying interaction dynamics.To address these challenges, we propose a method for community-aware detection of network states (CADENCE) based on the premise of stable interaction time-frames within network communities. We simultaneously detect network communities and partition the global interaction activity into scale-adaptive snapshots where the level of interaction within communities remains stable. We model a temporal network as a node-node-time tensor and use a structured canonical polyadic decomposition with a piece-wise constant temporal factor to iteratively identify communities and their activity levels. We demonstrate that transitions between network snapshots learned by CADENCE constitute network change points of better quality than those predicted by state-of-the-art network change point detectors. Furthermore, the network structure within individual snapshots reflects ground truth communities better than baselines for adaptive tensor granularity. Through a case study on a real-world Reddit dataset, we showcase the interpretability of CADENCE motivated snapshots as periods separated by significant events.
更多查看译文
关键词
dynamic,detection,cadence,community-aware
AI 理解论文
溯源树
样例
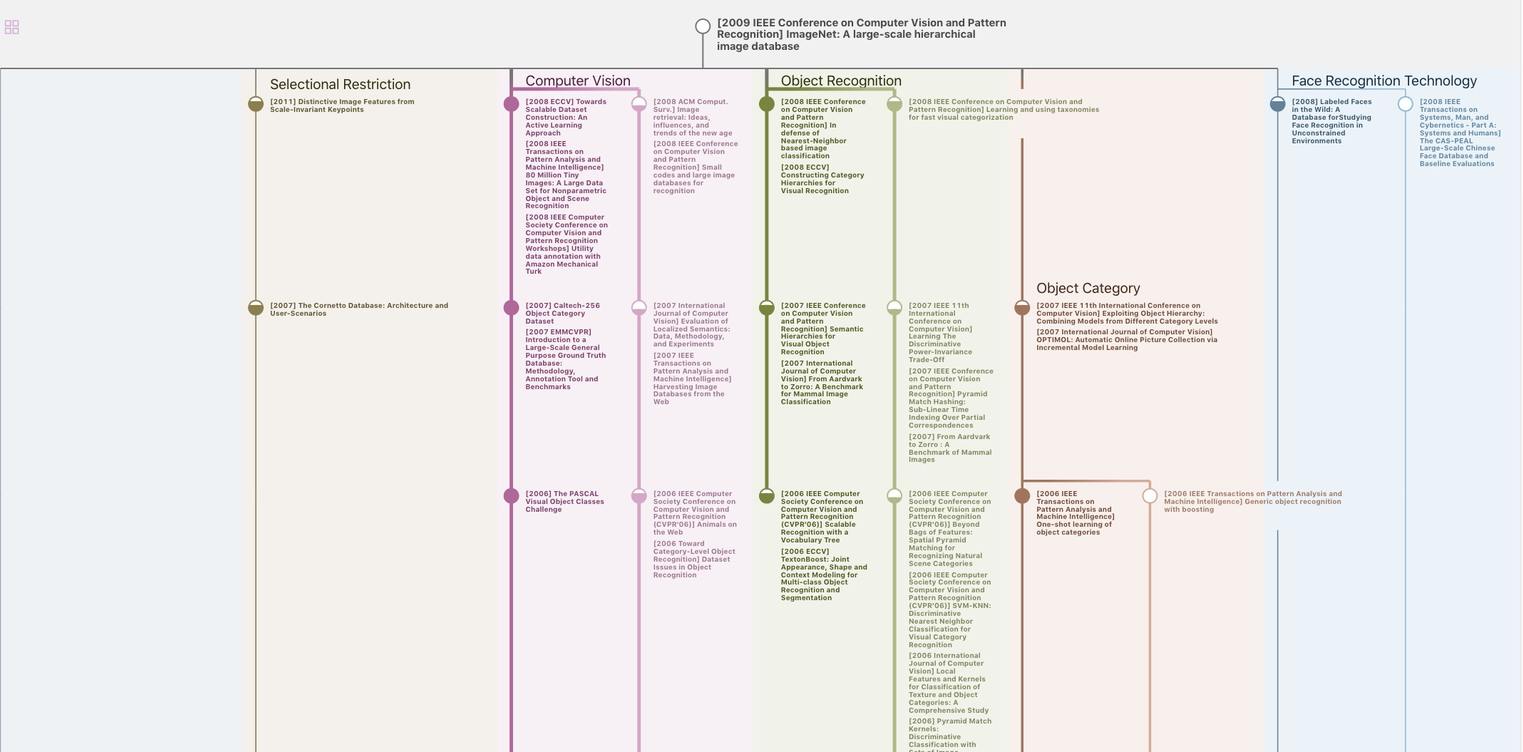
生成溯源树,研究论文发展脉络
Chat Paper
正在生成论文摘要