The Impact of the bug number on Effort-Aware Defect Prediction: An Empirical Study.
Internetware(2023)
摘要
Previous research have utilized public software defect datasets such as NASA, RELINK, and SOFTLAB, which only contain class label information. Almost all Effort-Aware Defect Prediction (EADP) studies are carried out around these datasets. However, EADP studies typically relying on bug density (i.e., the ratio between bug numbers and the lines of code) for ranking software modules. In order to investigate the impact of neglecting bug number information in software defect datasets on the performance of EADP models, we examine the performance degradation of the best-performing learning to rank methods when class labels are utilized instead of bug numbers. The experimental results show that neglecting bug number information in building EADP models results in an increase in the detected bugs. However, it also leads to a significant increase in the initial false alarms, ranging from 45.5% to 90.9% of the datasets, and an significant increase in the modules that need to be inspected, ranging from 5.2% to 70.4%. Therefore, we recommend not only the class labels but also the bug number information should be disclosed when publishing software defect datasets, in order to construct more accurate EADP models.
更多查看译文
AI 理解论文
溯源树
样例
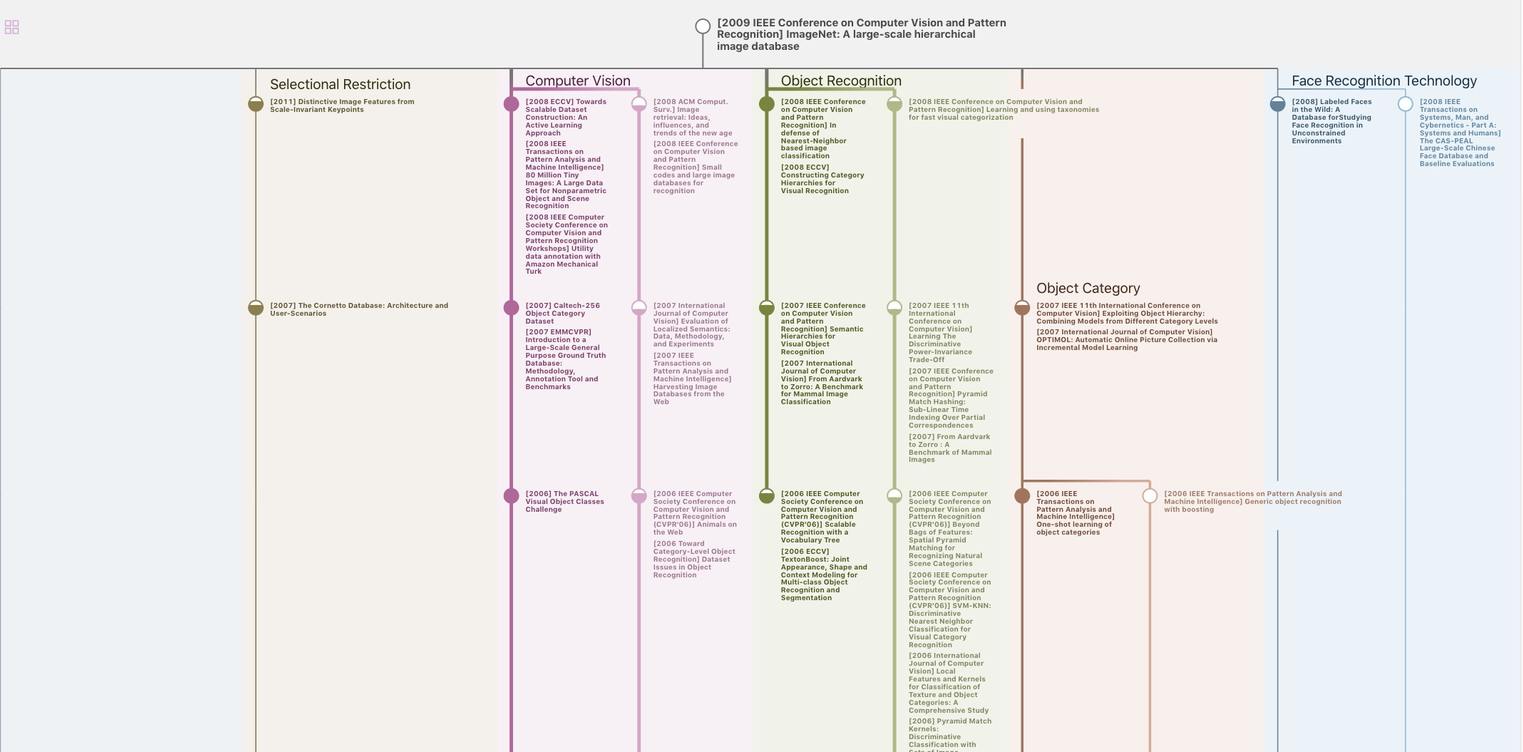
生成溯源树,研究论文发展脉络
Chat Paper
正在生成论文摘要