Structural-semantics Guided Program Simplification for Understanding Neural Code Intelligence Models.
Internetware(2023)
摘要
Neural code intelligence models are cutting-edge automated code understanding technologies that have achieved remarkable performance in various software engineering tasks. However, the lack of deep learning models’ interpretability hinders the application of deep learning based code intelligence models in real-world scenarios, particularly in security-critical domains. Previous studies use program simplification to understand neural code intelligence models, but they have overlooked the fact that the most significant difference between source code and natural language is the code’s structural semantics. In this paper, we first conduct an empirical study to identify the critical code structural semantic features valued by neural code intelligence models, and then we propose a novel program simplification method called SSGPS (Structural-Semantics Guided Program Simplification). Results on three code summarization models show that SSGPS can reduce training and testing time by 20-40% while controlling the decrease in model performance by less than 4%, demonstrating that our method can retain the critical code structural semantics for understanding neural code intelligence models.
更多查看译文
AI 理解论文
溯源树
样例
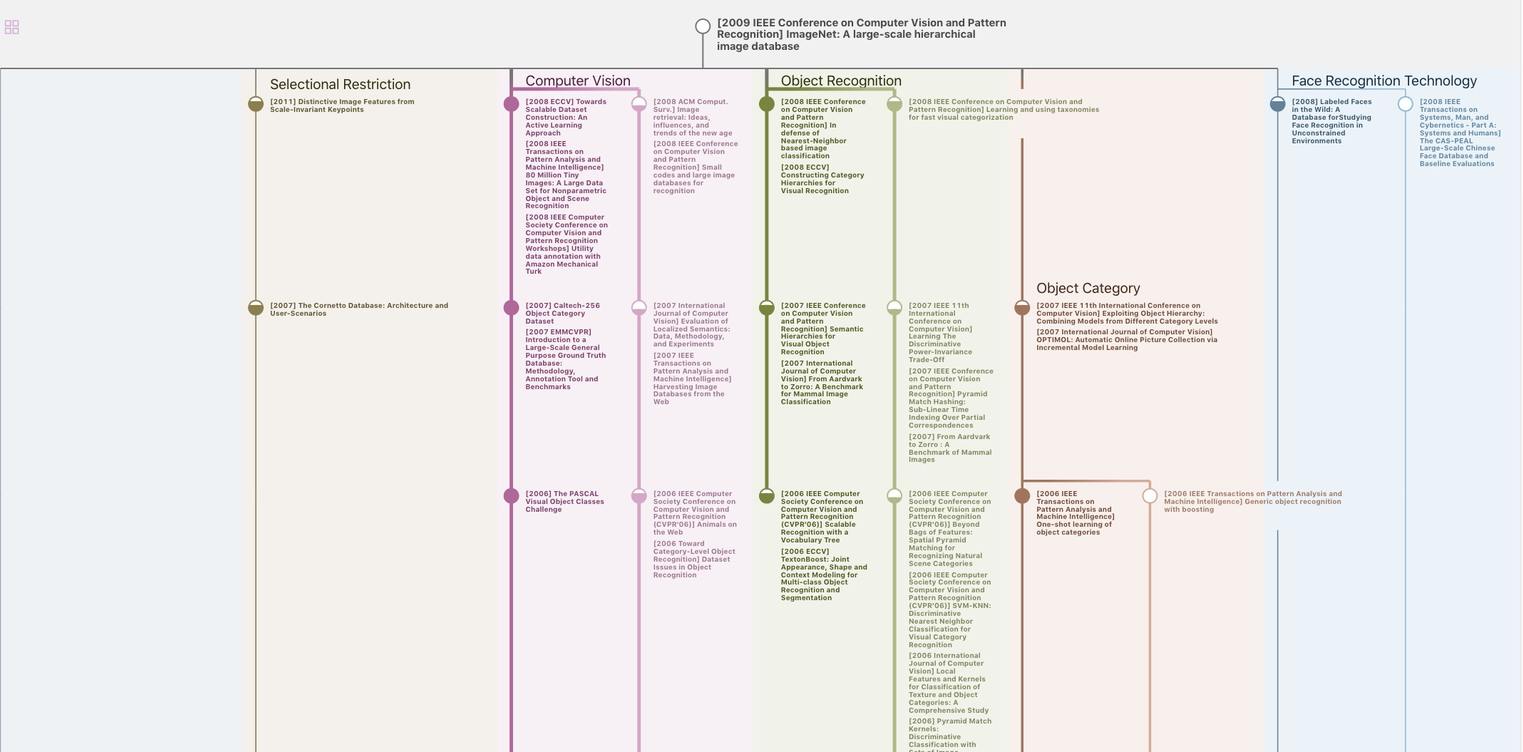
生成溯源树,研究论文发展脉络
Chat Paper
正在生成论文摘要