Enhanced Lightweight Network with CNN and Improved Transformer for Image Super-Resolution.
ICNC-FSKD(2023)
Chengdu University of Information Technology | Advanced Cryptography and System Security Key Laboratory of Sichuan Province
Abstract
In recent years, there have been significant advancements in deep learning-based lightweight image super-resolution (SR) reconstruction techniques. However, in practical applications, there are still challenges to be addressed. Many existing lightweight SR reconstruction algorithms simplify the model by reducing the number of model parameters or changing the combination of convolutions, which results in significantly decreased model performance and stability, leading to poor reconstruction results. To address this issue, we proposed a Cosine Self-Attention mechanism and deepens the network depth to improve the Swin Transformer, enhanced the model’s performance and stability. Experimental results show that the proposed approach achieves stronger and more stable reconstruction performance with lower parameter count than existing lightweight SR models, outperforming them in reconstruction results and model complexity. The PSNR/SSIM of our proposed method for ×2 and ×4 SR reconstruction on the Set5, Set14, BSD100, Urban100, and Manga109 datasets exceed those of most existing lightweight SR models.
MoreTranslated text
Key words
Deep learning,Image super-resolution,Attention mechanism,Swin Transformer
求助PDF
上传PDF
View via Publisher
AI Read Science
AI Summary
AI Summary is the key point extracted automatically understanding the full text of the paper, including the background, methods, results, conclusions, icons and other key content, so that you can get the outline of the paper at a glance.
Example
Background
Key content
Introduction
Methods
Results
Related work
Fund
Key content
- Pretraining has recently greatly promoted the development of natural language processing (NLP)
- We show that M6 outperforms the baselines in multimodal downstream tasks, and the large M6 with 10 parameters can reach a better performance
- We propose a method called M6 that is able to process information of multiple modalities and perform both single-modal and cross-modal understanding and generation
- The model is scaled to large model with 10 billion parameters with sophisticated deployment, and the 10 -parameter M6-large is the largest pretrained model in Chinese
- Experimental results show that our proposed M6 outperforms the baseline in a number of downstream tasks concerning both single modality and multiple modalities We will continue the pretraining of extremely large models by increasing data to explore the limit of its performance
Upload PDF to Generate Summary
Must-Reading Tree
Example
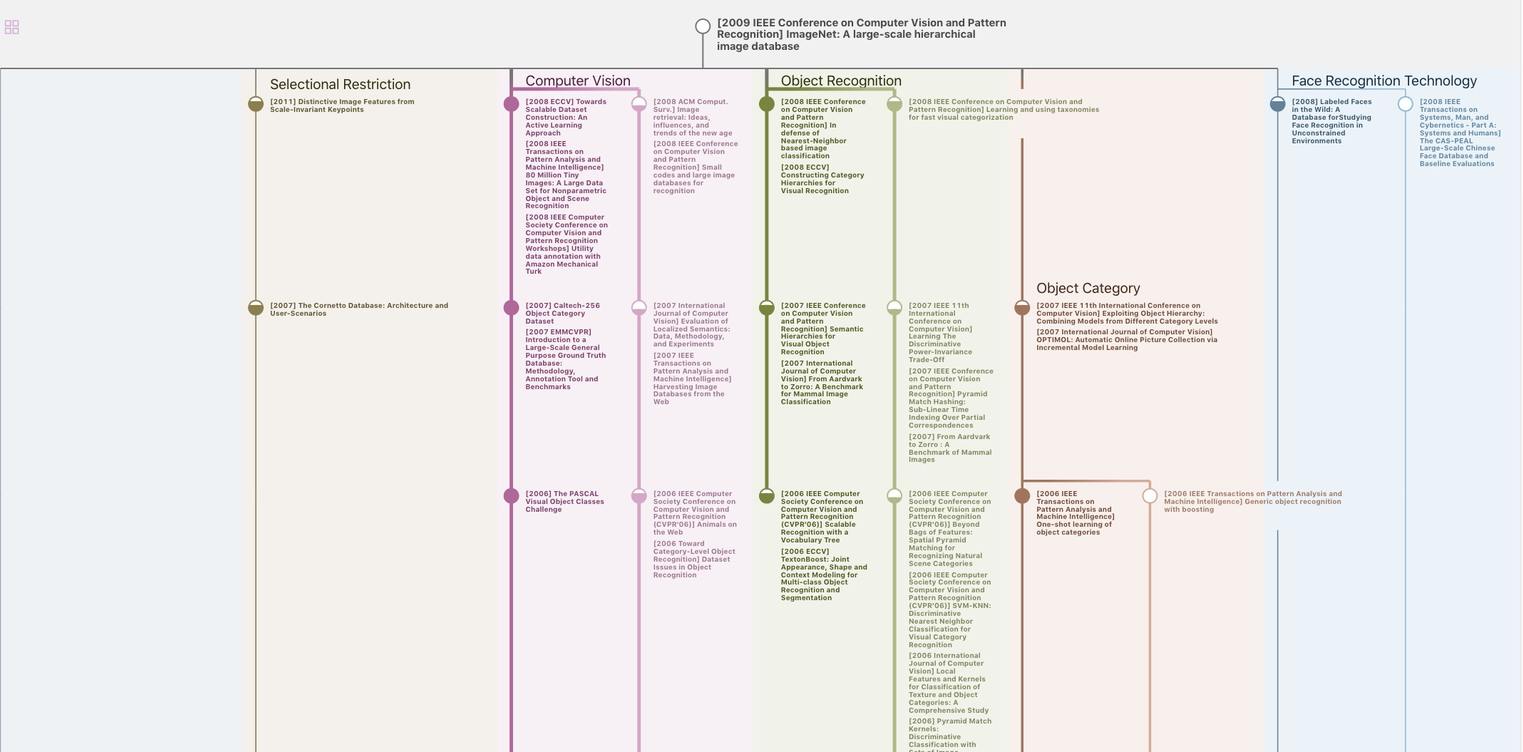
Generate MRT to find the research sequence of this paper
Related Papers
2018
被引用1019 | 浏览
2022
被引用30 | 浏览
Data Disclaimer
The page data are from open Internet sources, cooperative publishers and automatic analysis results through AI technology. We do not make any commitments and guarantees for the validity, accuracy, correctness, reliability, completeness and timeliness of the page data. If you have any questions, please contact us by email: report@aminer.cn
Chat Paper