Nonlinear-by-Linear: Guaranteeing Error Bounds in Compressive Autoencoders.
IC3(2023)
摘要
Neural network-based autoencoders have been successfully used in many image and video compression applications. Unfortunately, these approaches do not provide error guarantees on an instance by instance basis, making their use limited in scientific and other applications. In this paper, we propose a new Guaranteed Autoencoder (GAE) that provides error guarantees on all instances. Our approach is based on a key observation that neural networks with piecewise linear activation units (PLUs) can be represented as instance-specific linear operators. This implies that a global model can be converted into a set of local models. The generated linear operators then enable us to find the direct and linear relationship between the compressed data and reconstructed data. We are then able to represent the residual of the reconstruction of each instance in the SVD basis of the corresponding linear operator and then augment the reconstruction until an error bound is met. Additionally, we show that the above approach based on the use of linear operators can be applied in the presence of both fully connected and convolutional layers. Thus, our approach is reasonably general and should find a large number of applications. We demonstrate our approach using both large-scale scientific data and public-domain image datasets. Experimental results show that the GAE can successfully satisfy user-provided error bounds at an instance level while achieving high compression. Additionally, the computational overhead of our approach is small.
更多查看译文
AI 理解论文
溯源树
样例
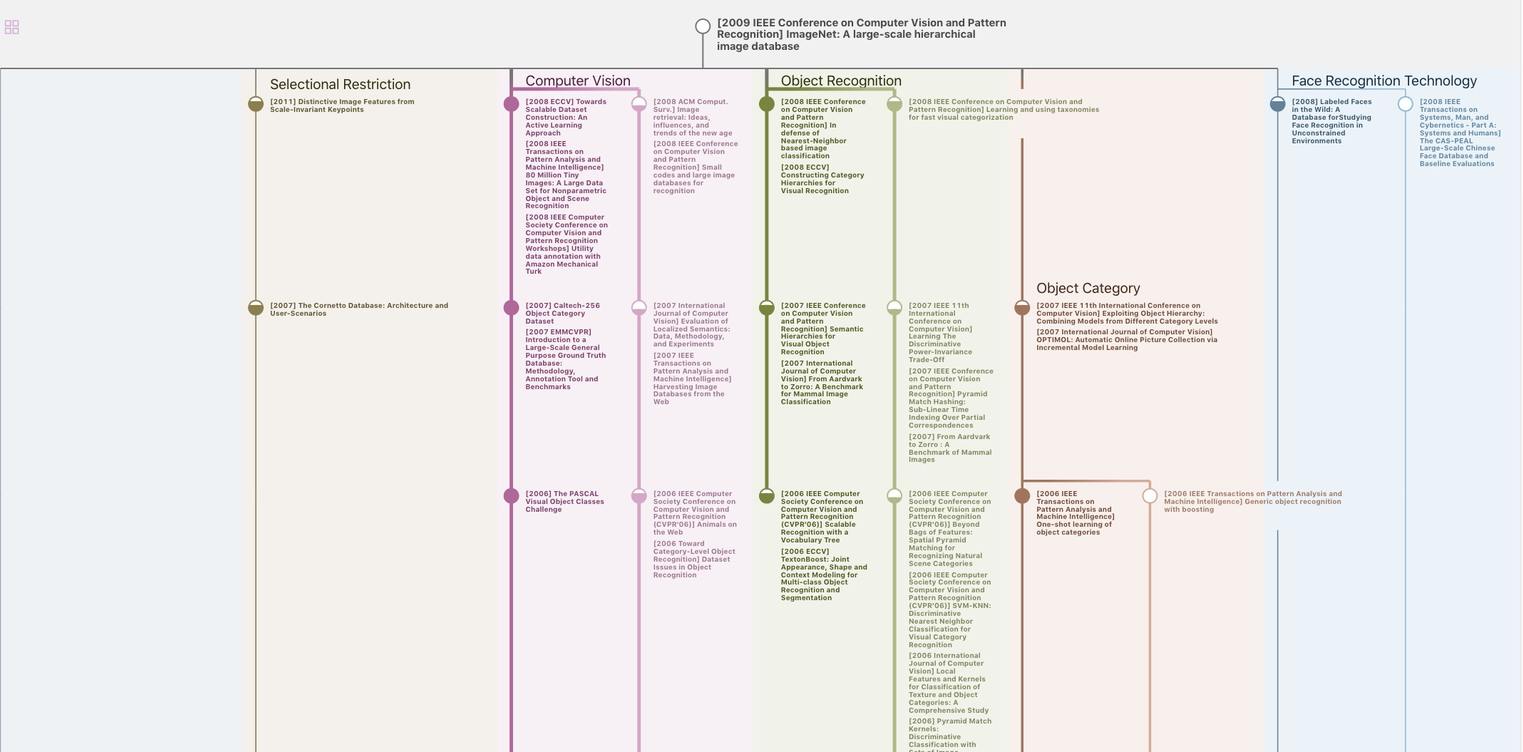
生成溯源树,研究论文发展脉络
Chat Paper
正在生成论文摘要