Enhancing In-Vehicle Network Security Through Bitstream Feature Extraction-Based Intrusion Detection.
IC3(2023)
摘要
The in-vehicle network (IVN) is highly vulnerable due to its inherent structure, and the continuous introduction of new features in next-generation vehicles only exacerbates this issue. To address this problem, a proposed intrusion detection approach for in-vehicle networks is based on bitstream feature extraction and autocorrelation analysis. This approach aims to overcome the limitations associated with memory utilization and processing overhead vulnerability by comparing payload features using nibble. The Controller Area Network (CAN) data is highly susceptible, and organizations worldwide are working on developing Intrusion Detection Systems (IDSs) to protect vehicles. Nibbles are four-bit units of digital information, and autocorrelation measures the correlation of a variable with itself over time. In the proposed approach, the CAN payload is processed using nibble feature extraction and fed into a deep-learning CNN for attack classification. This approach presents a promising solution for enhancing the security of in-vehicle networks, by utilizing advanced machine-learning techniques to improve the security of vehicle systems.
更多查看译文
AI 理解论文
溯源树
样例
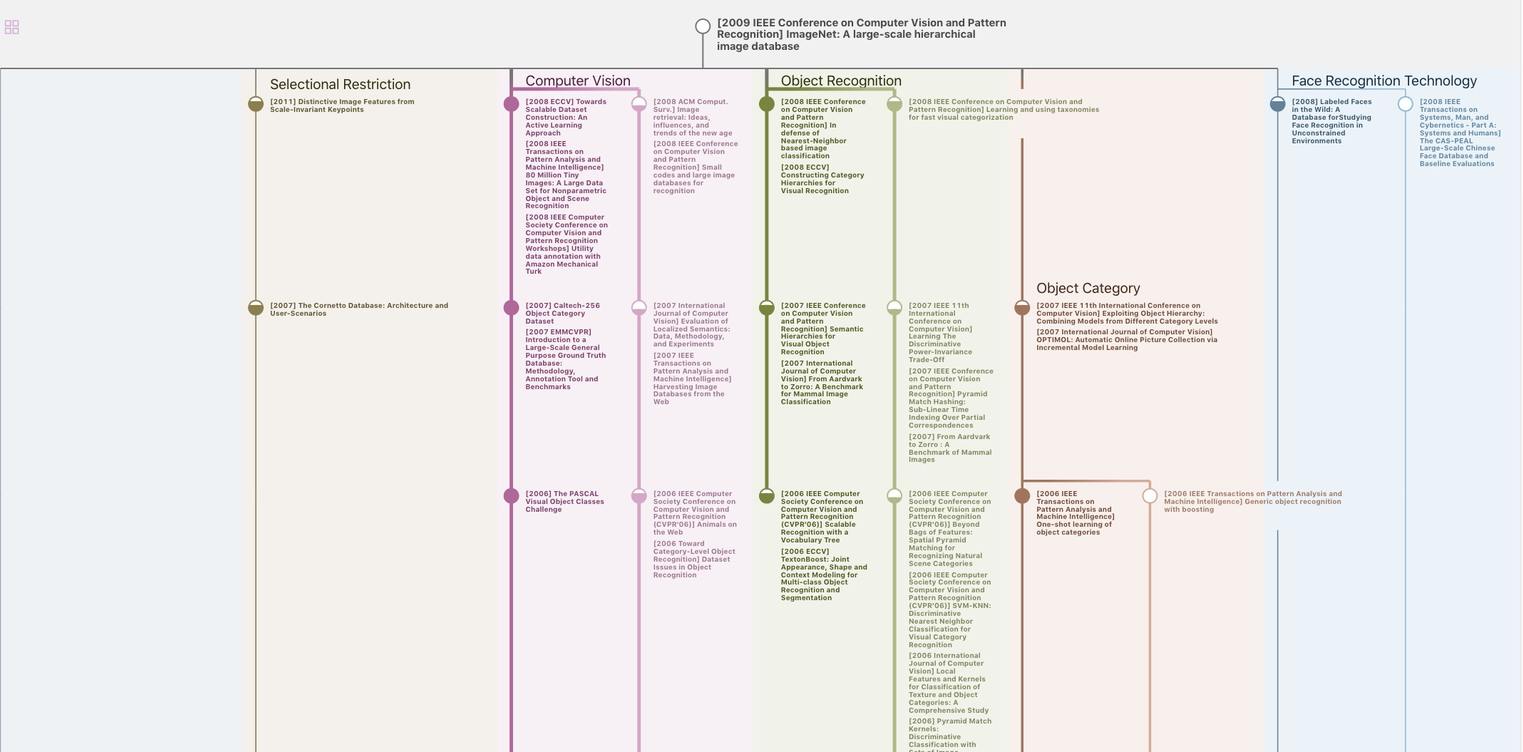
生成溯源树,研究论文发展脉络
Chat Paper
正在生成论文摘要