Enhance Early Diagnosis Accuracy of Alzheimer's Disease by Elucidating Interactions Between Amyloid Cascade and Tau Propagation
MEDICAL IMAGE COMPUTING AND COMPUTER ASSISTED INTERVENTION, MICCAI 2023, PT III(2023)
摘要
Amyloid-beta (A beta) deposition and tau neurofibrillary tangles (tau) are important hallmarks of Alzheimer's disease (AD). Although converging evidence shows that the interaction between A beta and tau is the gateway to understanding the etiology of AD, these two AD hallmarks are often treated as independent variables in the current state-of-the-art early diagnostic model for AD, which might be partially responsible for the issue of lacking explainability. Inspired by recent progress in systems biology, we formulate the evolving biological process of A beta cascade and tau propagation into a closed-loop feedback system where the system dynamics are constrained by region-to-region white matter fiber tracts in the brain. On top of this, we conceptualize that A beta-tau interaction, following the principle of optimal control, underlines the pathophysiological mechanism of AD. In this context, we propose a deep reaction-diffusion model that leverages the capital of deep learning and insights into systems biology, which allows us to (1) enhance the prediction accuracy of developing AD and (2) uncover the latent control mechanism of A beta-tau interactions. We have evaluated our novel explainable deep model on the neuroimaging data in Alzheimer's Disease Neuroimaging Initiative (ADNI), where we achieve not only a higher prediction accuracy for disease progression but also a better understanding of disease etiology than conventional ("black-box") deep models.
更多查看译文
关键词
Alzheimer's disease,Graph neural networks,Partial differential equations,A beta-tau interaction,Systems biology
AI 理解论文
溯源树
样例
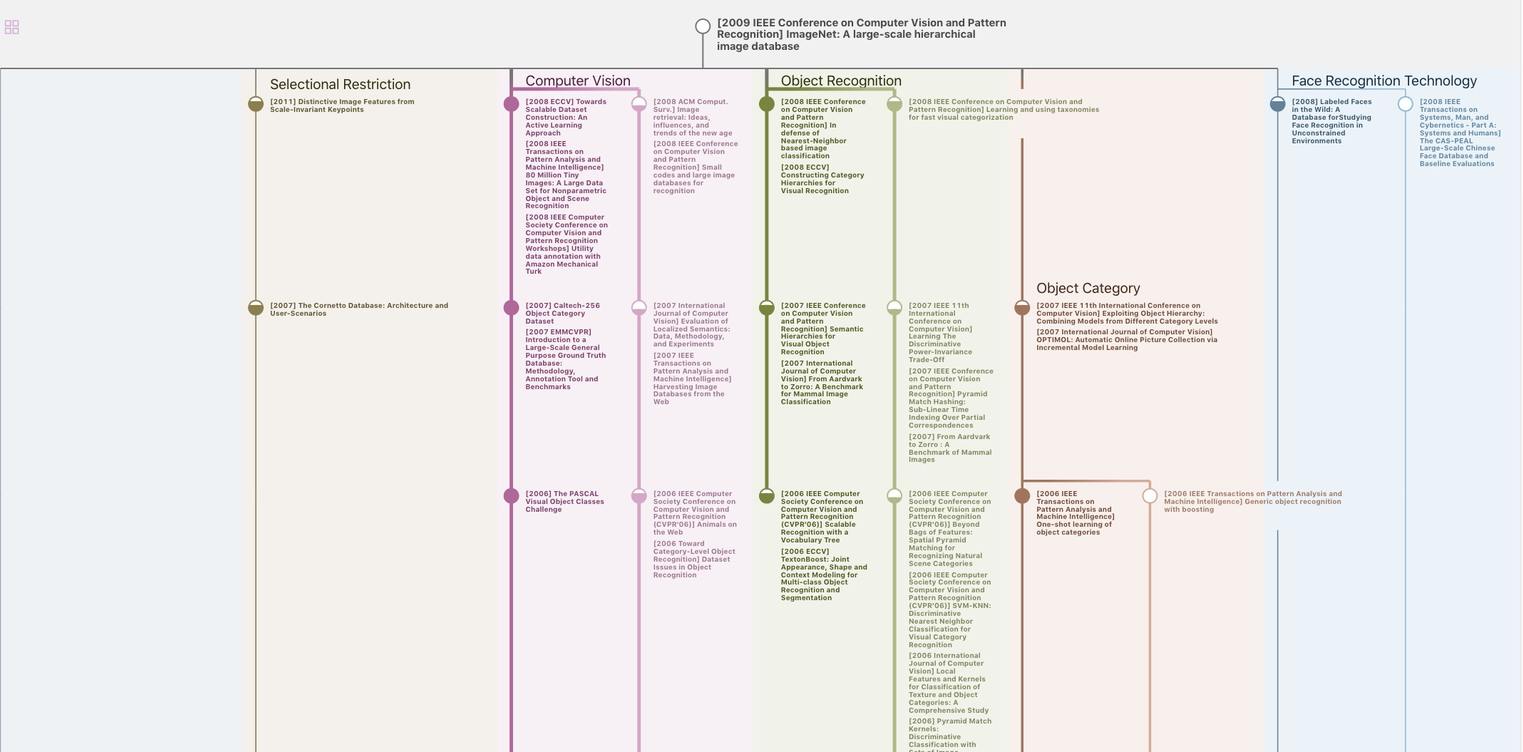
生成溯源树,研究论文发展脉络
Chat Paper
正在生成论文摘要