CheXstray: A Real-Time Multi-Modal Monitoring Workflow for Medical Imaging AI
MEDICAL IMAGE COMPUTING AND COMPUTER ASSISTED INTERVENTION, MICCAI 2023, PT III(2023)
摘要
Clinical AI applications, particularly medical imaging, are increasingly being adopted in healthcare systems worldwide. However, a crucial question remains: what happens after the AI model is put into production? We present our novel multi-modal model drift framework capable of tracking drift without contemporaneous ground truth using only readily available inputs, namely DICOM metadata, image appearance representation from a variational autoencoder (VAE), and model output probabilities. CheXStray was developed and tested using CheXpert, PadChest and Pediatric Pneumonia Chest X-ray datasets and we demonstrate that our framework generates a strong proxy for ground truth performance. In this work, we offer new insights into the challenges and solutions for observing deployed medical imaging AI and make three key contributions to real-time medical imaging AI monitoring: (1) proof-of-concept for medical imaging drift detection including use of VAE and domain specific statistical methods (2) a multi-modal methodology for measuring and unifying drift metrics (3) new insights into the challenges and solutions for observing deployed medical imaging AI. Our framework is released as open-source tools so that others may easily run their own workflows and build upon our work. Code available at: https://github.com/microsoft/MedImaging-ModelDriftMonitoring
更多查看译文
关键词
Medical imaging,Model drift,AI monitoring
AI 理解论文
溯源树
样例
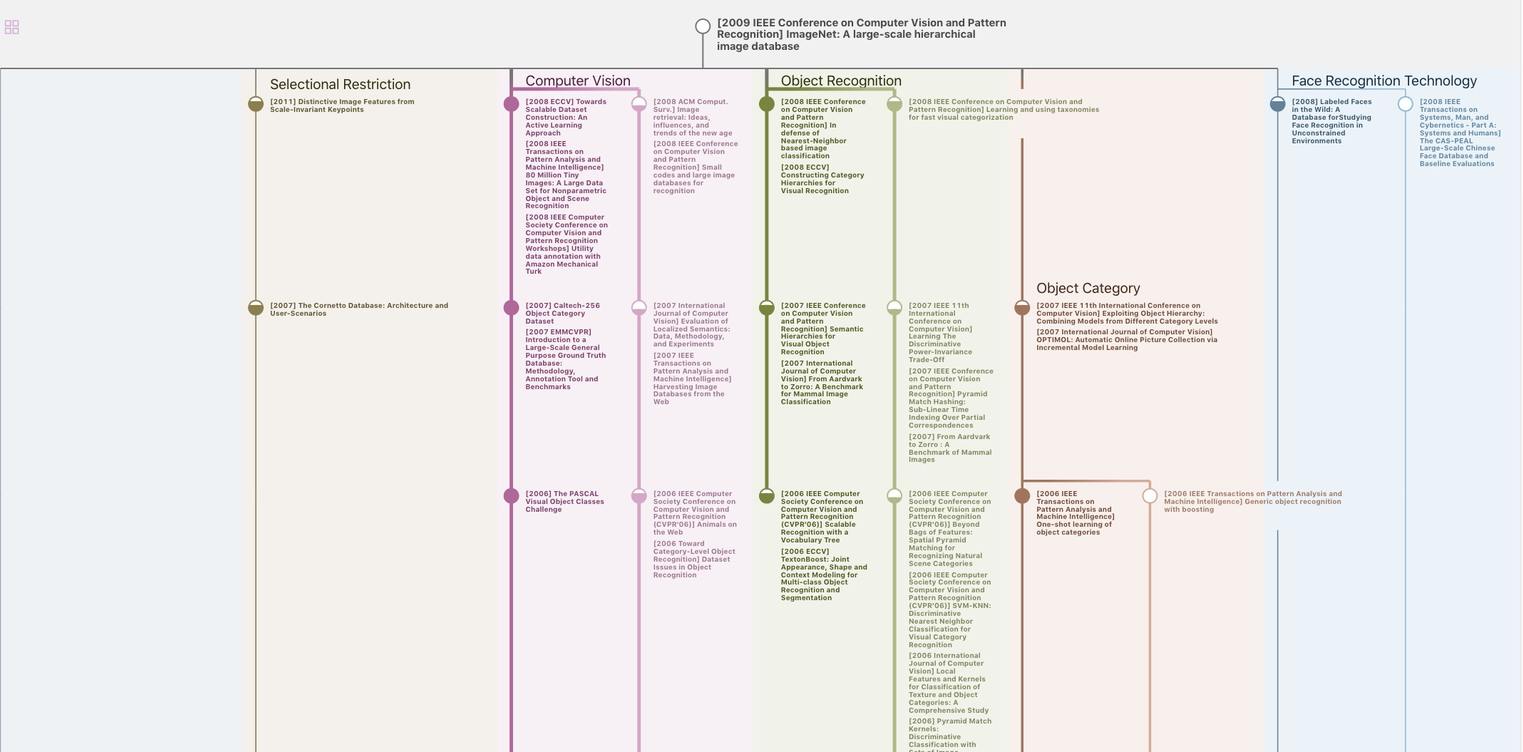
生成溯源树,研究论文发展脉络
Chat Paper
正在生成论文摘要