FedGrav: An Adaptive Federated Aggregation Algorithm for Multi-institutional Medical Image Segmentation
MEDICAL IMAGE COMPUTING AND COMPUTER ASSISTED INTERVENTION, MICCAI 2023, PT II(2023)
摘要
With the increasingly strengthened data privacy acts and the difficult data centralization, Federated Learning (FL) has become an effective solution to collaboratively train the model while preserving each client's privacy. FedAvg is a standard aggregation algorithm that makes the proportion of the dataset size of each client an aggregation weight. However, it can't deal with non-independent and identically distributed (non-IID) data well because of its fixed aggregation weights and the neglect of data distribution. The paper presents a new aggregation strategy called FedGrav, which is designed to handle non-IID datasets and is inspired by the law of universal gravitation in physics. FedGrav can dynamically adjust the aggregation weights based on the training condition of local models throughout the entire training process, making it an effective solution for non-IID data. The model affinity is creatively proposed by considering both the differences of sample size on the client and the discrepancies among local models. It considers the client sample size as the mass of the local model and defines the model graph distance based on neural network topology. By calculating the affinity among local models, FedGrav can explore internal correlations of them and improve the aggregation weights. The proposed FedGrav has been applied to the CIFAR-10 and the MICCAI Federated Tumor Segmentation (FeTS) Challenge 2021 datasets, and the validation results show that our method outperforms the previous state-of-the-art by 1.54 mean DSC and 2.89 mean HD95. The source code will be available on Github.
更多查看译文
关键词
Federated Learning,Brain Tumor Segmentation,FedGrav,Model Affinity,Graph Distance
AI 理解论文
溯源树
样例
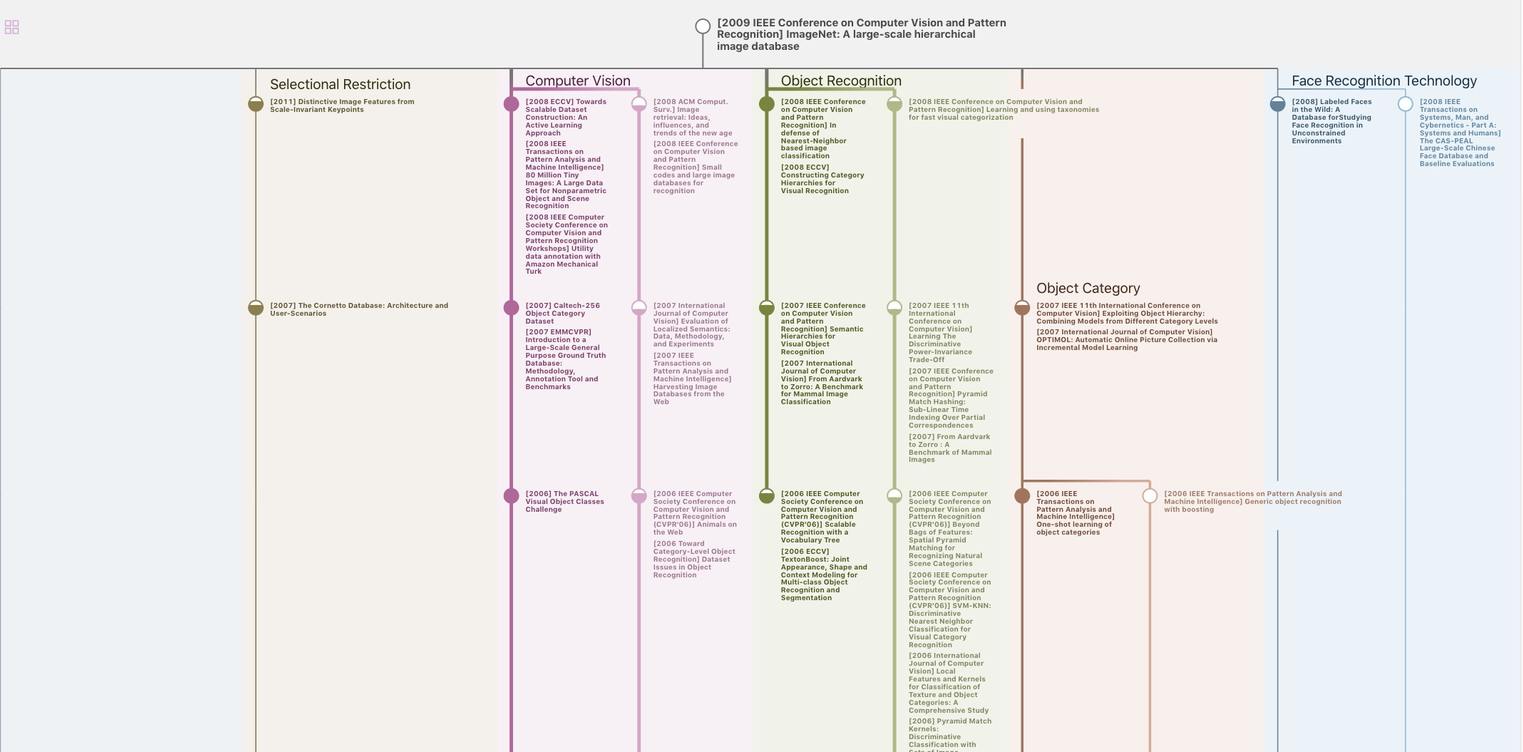
生成溯源树,研究论文发展脉络
Chat Paper
正在生成论文摘要