Bridging Ex-Vivo Training and Intra-operative Deployment for Surgical Margin Assessment with Evidential Graph Transformer
MEDICAL IMAGE COMPUTING AND COMPUTER ASSISTED INTERVENTION, MICCAI 2023, PT VII(2023)
摘要
PURPOSE: The use of intra-operative mass spectrometry along with Graph Transformer models showed promising results for margin detection on ex-vivo data. Although highly interpretable, these methods lack the ability to handle the uncertainty associated with intra-operative decision making. In this paper for the first time, we propose Evidential Graph Transformer network, a combination of attention mapping and uncertainty estimation to increase the performance and interpretability of surgical margin assessment. METHODS: The Evidential Graph Transformer was formulated to output the uncertainty estimation along with intermediate attentions. The performance of the model was compared with different baselines in an ex-vivo cross-validation scheme, with extensive ablation study. The association of the model with clinical features were explored. The model was further validated for a prospective ex-vivo data, as well as a breast conserving surgery intra-operative data. RESULTS: The purposed model outperformed all baselines, statistically significantly, with average balanced accuracy of 91.6%. When applied to intra-operative data, the purposed model improved the false positive rate of the baselines. The estimated attention distribution for status of different hormone receptors agreed with reported metabolic findings in the literature. CONCLUSION: Deployment of ex-vivo models is challenging due to the tissue heterogeneity of intra-operative data. The proposed Evidential Graph Transformer is a powerful tool that while providing the attention distribution of biochemical subbands, improve the surgical deployment power by providing decision confidence.
更多查看译文
关键词
Intra-operative deployment,Uncertainty estimation,Interpretation,Graph transformer network,Breast cancer margin
AI 理解论文
溯源树
样例
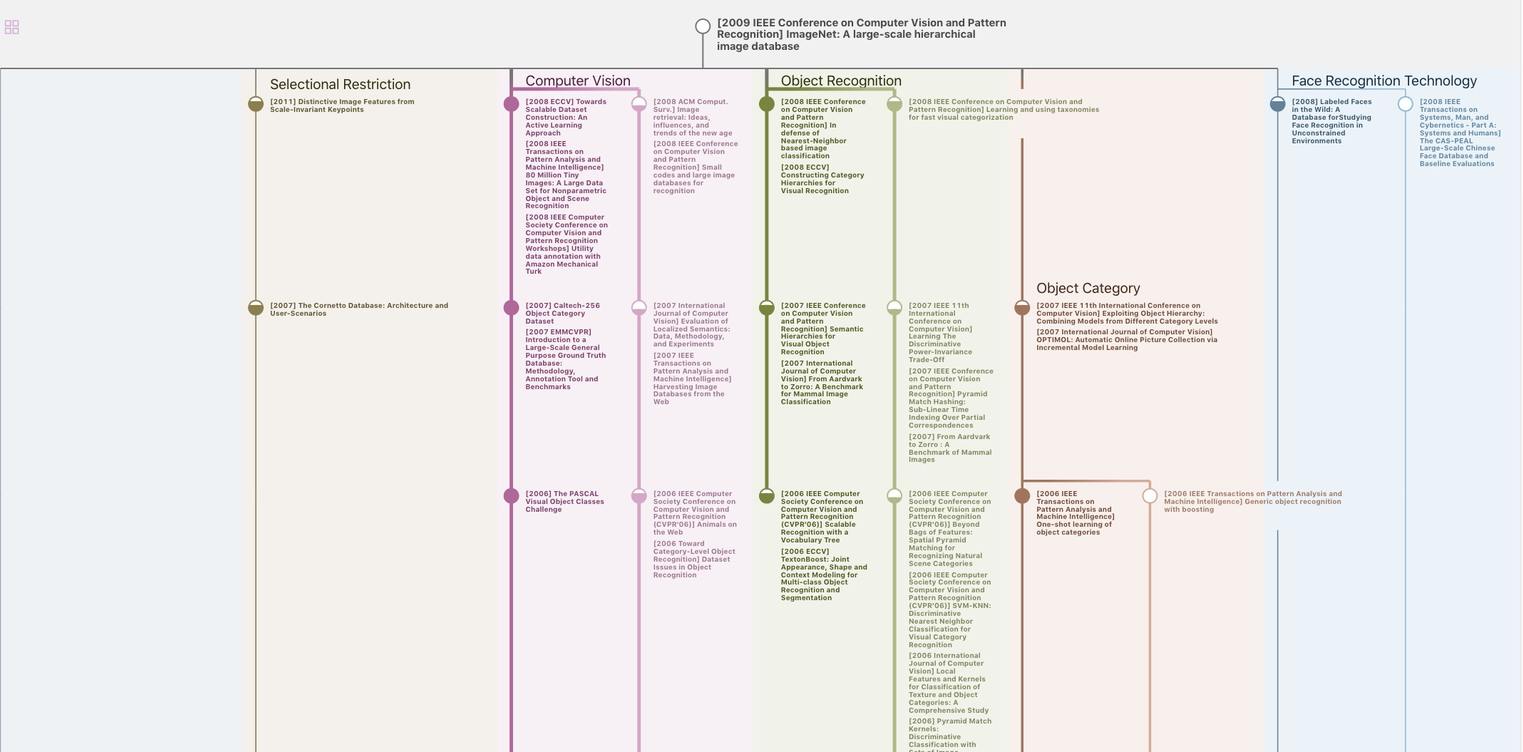
生成溯源树,研究论文发展脉络
Chat Paper
正在生成论文摘要