Multi-task Learning for Hierarchically-Structured Images: Study on Echocardiogram View Classification
SIMPLIFYING MEDICAL ULTRASOUND, ASMUS 2023(2023)
摘要
Echocardiography is a crucial and widely adopted imaging modality for diagnosing and monitoring cardiovascular diseases. Deep learning has been proven effective in analyzing medical images but is limited in echocardiograms due to the complexity of image acquisition and interpretation. One crucial initial step to address this is automatically identifying the correct echocardiogram video views. Several studies have used deep learning and traditional image-processing techniques for this task. The authors propose an ablation study on a multi-task learning scheme with a hierarchically structured model output that arranges views in a tree structure. The proposed model, named "Multi-task Residual Neural Network (MTRNN) with masked loss", uses a conditional probabilistic training method and demonstrates superior performance for echocardiogram view classification. While the model has only been validated for the echocardiogram video classification task, it can be easily generalized to any medical image classification scenario with a hierarchical structure among the data labels.
更多查看译文
关键词
Echocardiagram,Multi-task learning,View classification
AI 理解论文
溯源树
样例
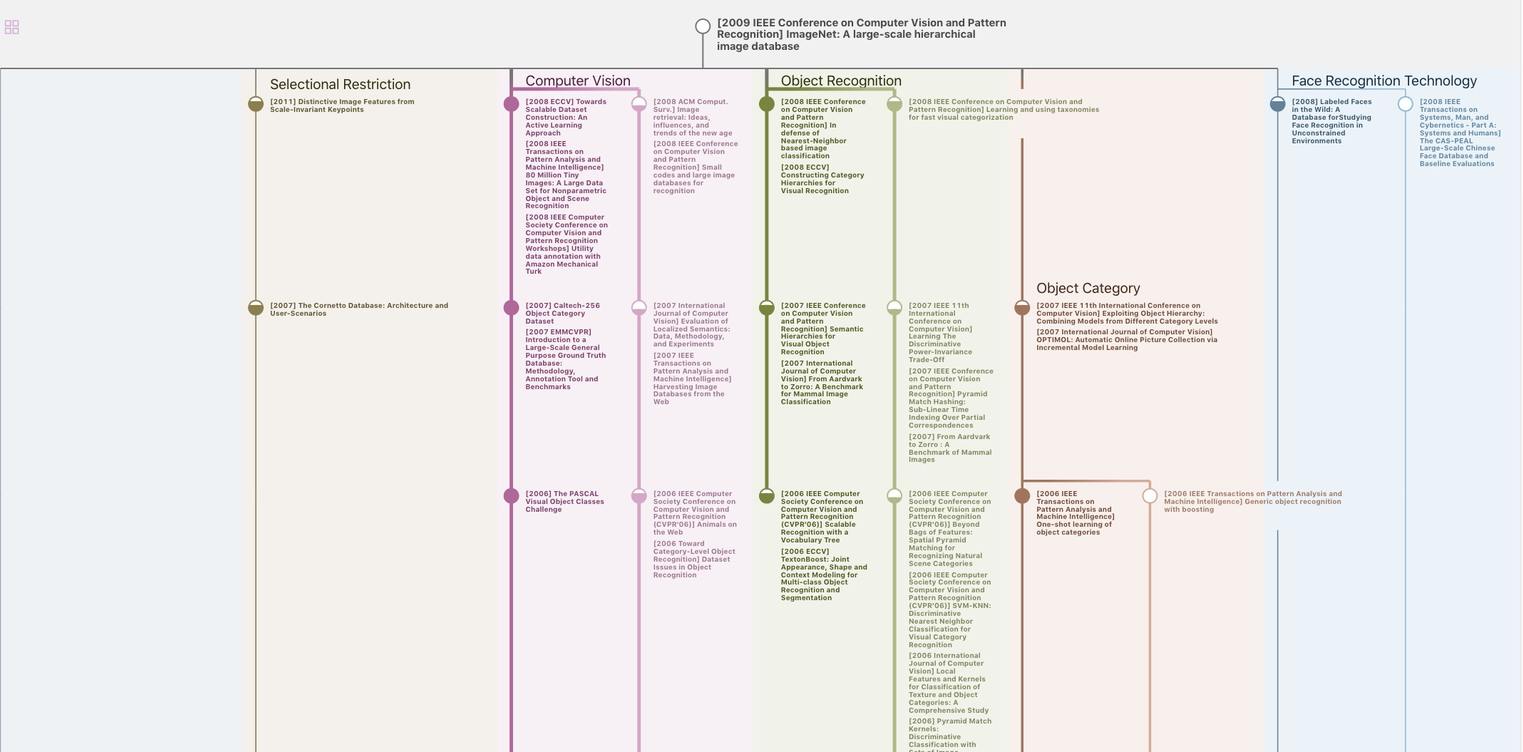
生成溯源树,研究论文发展脉络
Chat Paper
正在生成论文摘要