How reliable are posterior class probabilities in automatic music classification?
PROCEEDINGS OF THE 18TH INTERNATIONAL AUDIO MOSTLY CONFERENCE, AM 2023(2023)
摘要
Music classification algorithms use signal processing and machine learning approaches to extract and enrich metadata for audio recordings in music archives. Common tasks include music genre classification, where each song is assigned a single label (such as Rock, Pop, or Jazz), and musical instrument classification. Since music metadata can be ambiguous, classification algorithms cannot always achieve fully accurate predictions. Therefore, our focus extends beyond the correctly estimated class labels to include realistic confidence values for each potential genre or instrument label. In practice, many state-of-the-art classification algorithms based on deep neural networks exhibit overconfident predictions, complicating the interpretation of the final output values. In this work, we examine whether the issue of overconfident predictions and, consequently, non-representative confidence values is also relevant to music genre classification and musical instrument classification. Moreover, we describe techniques to mitigate this behavior and assess the impact of deep ensembles and temperature scaling in generating more realistic confidence outputs, which can be directly employed in real-world music tagging applications.
更多查看译文
关键词
music information retrieval,music classification,uncertainty,temperature scaling,deep ensembles
AI 理解论文
溯源树
样例
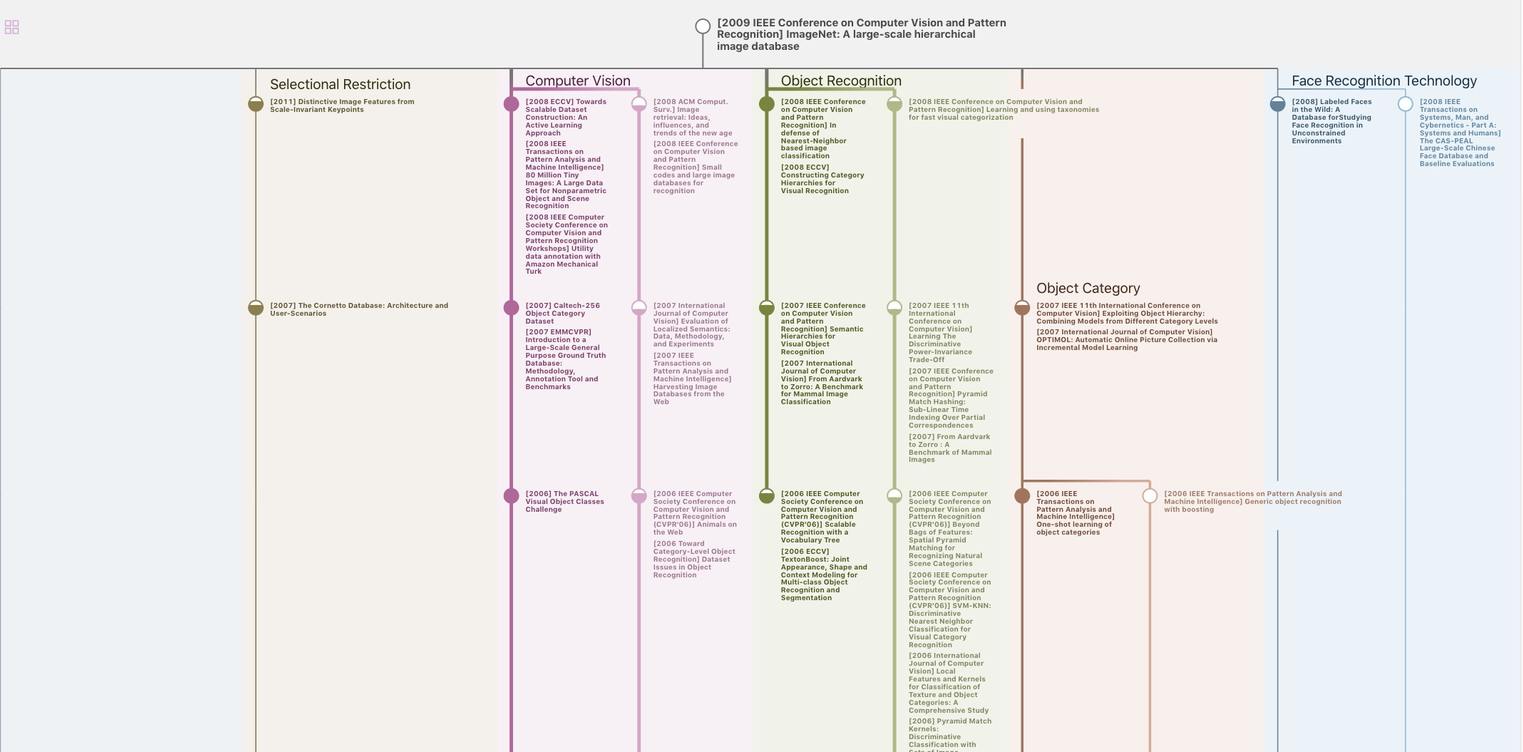
生成溯源树,研究论文发展脉络
Chat Paper
正在生成论文摘要