A Deep Learning-based Hybrid Precoding with Attention Mechanism for THz Massive MU-MIMO Systems.
ICC(2023)
摘要
Terahertz (THz) massive multiple-input multiple-output (MIMO) is considered as a key technology for future sixth-generation (6G) wireless communications, in which hybrid precoding facilitates an important trade-off of hardware cost and spectrum efficiency. However, the performance of traditional schemes is limited owing to the beam split effect and the non-convex optimization problem as well as the inter-user interference under imperfect channel state information (CSI) in THz massive multi-user (MU)-MIMO systems. To overcome these challenging problems, we propose an unsupervised convolutional neural network (CNN)-based hybrid precoding scheme with attention mechanism. Specifically, we first adopt the true-time-delay (TTD) structure to mitigate beam splitting. Then, to solve the non-convex optimization problem of TTD hybrid precoding and to further mitigate inter-user interference, we propose a robust hybrid precoding scheme by applying the attention mechanism and CNN, which can be trained to generate an optimal analog precoder targeting at an achievable rate maximization under imperfect CSI. Simulation results show that the proposed algorithm has good robustness and can maintain excellent achievable rate performance in the case of imperfect CSI.
更多查看译文
关键词
THz communication,massive MIMO,hybrid precoding,beam splitting,attention mechanism
AI 理解论文
溯源树
样例
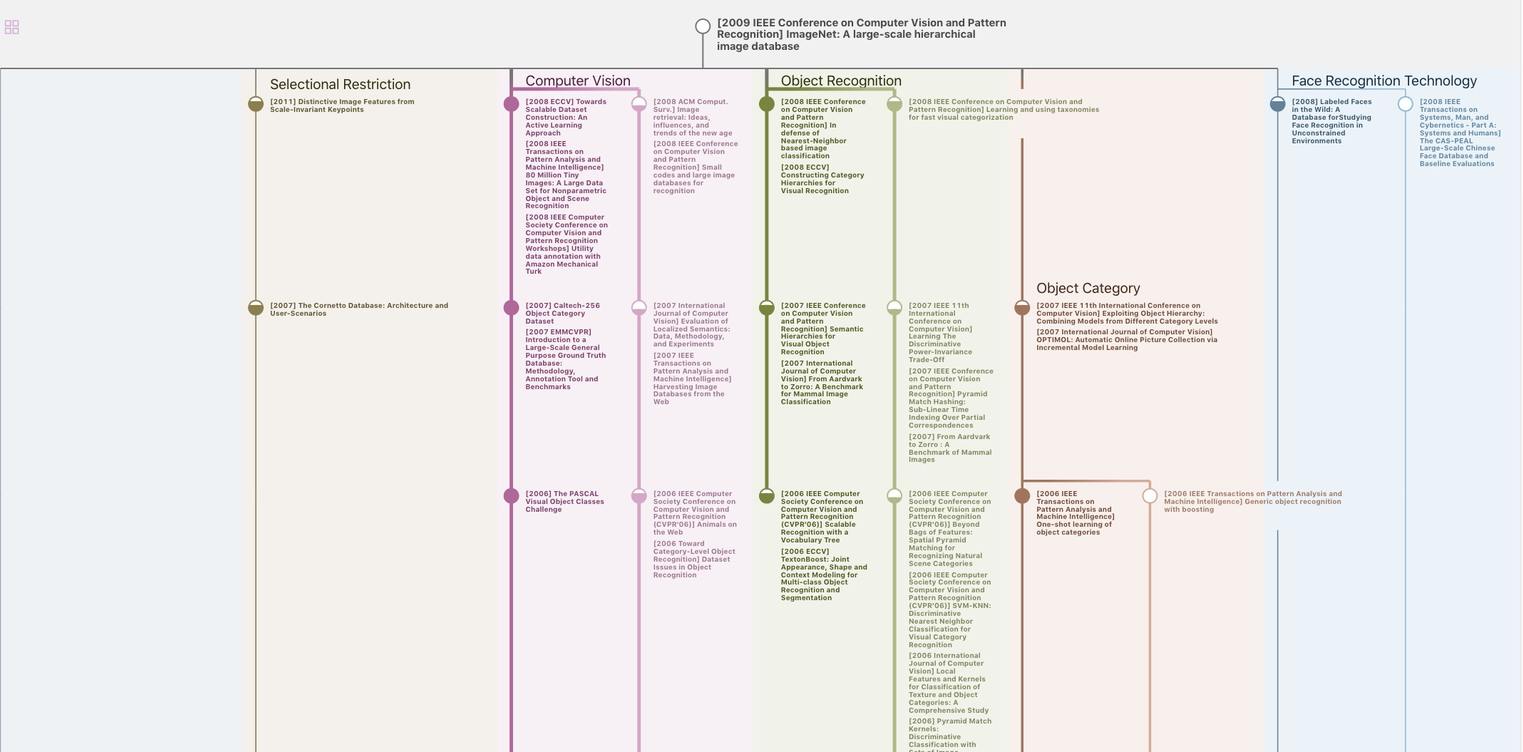
生成溯源树,研究论文发展脉络
Chat Paper
正在生成论文摘要