Energy-efficient Resource Allocation for the 6G Computing Network Based on Deep Reinforcement Learning
2023 IEEE INTERNATIONAL CONFERENCE ON COMMUNICATIONS WORKSHOPS, ICC WORKSHOPS(2023)
摘要
Based on native-AI, the 6G wireless network has the ability to handle higher-level and time-sensitive tasks, which leads to difficulties in efficient resource allocation. In this paper, the deep reinforcement learning is used to optimize system energy consumption and service quality by training joint allocation model of communication resources and computing resources. Firstly, a two-tier heterogeneous network of control base stations and data base stations is modeled considering computing power. Then, an intelligent resource allocation strategy is proposed that base stations coordinate communication and computing resources. Finally, DDPG, COMA, and MAPPO are conducted in a native-AI simulation platform for the task. The results show that native-AI architecture is benefit for auto-optimized network, multi-agent has better global control than single-agent, and MAPPO has superior trade-off in energy consumption and service quality. Compared with DDPG, MAPPO reduces average service delay and system energy consumption by 15.6% and 43.8% respectively.
更多查看译文
关键词
Native-AI,computing resources,resource allocation,deep reinforcement learning,multi-agent,6G
AI 理解论文
溯源树
样例
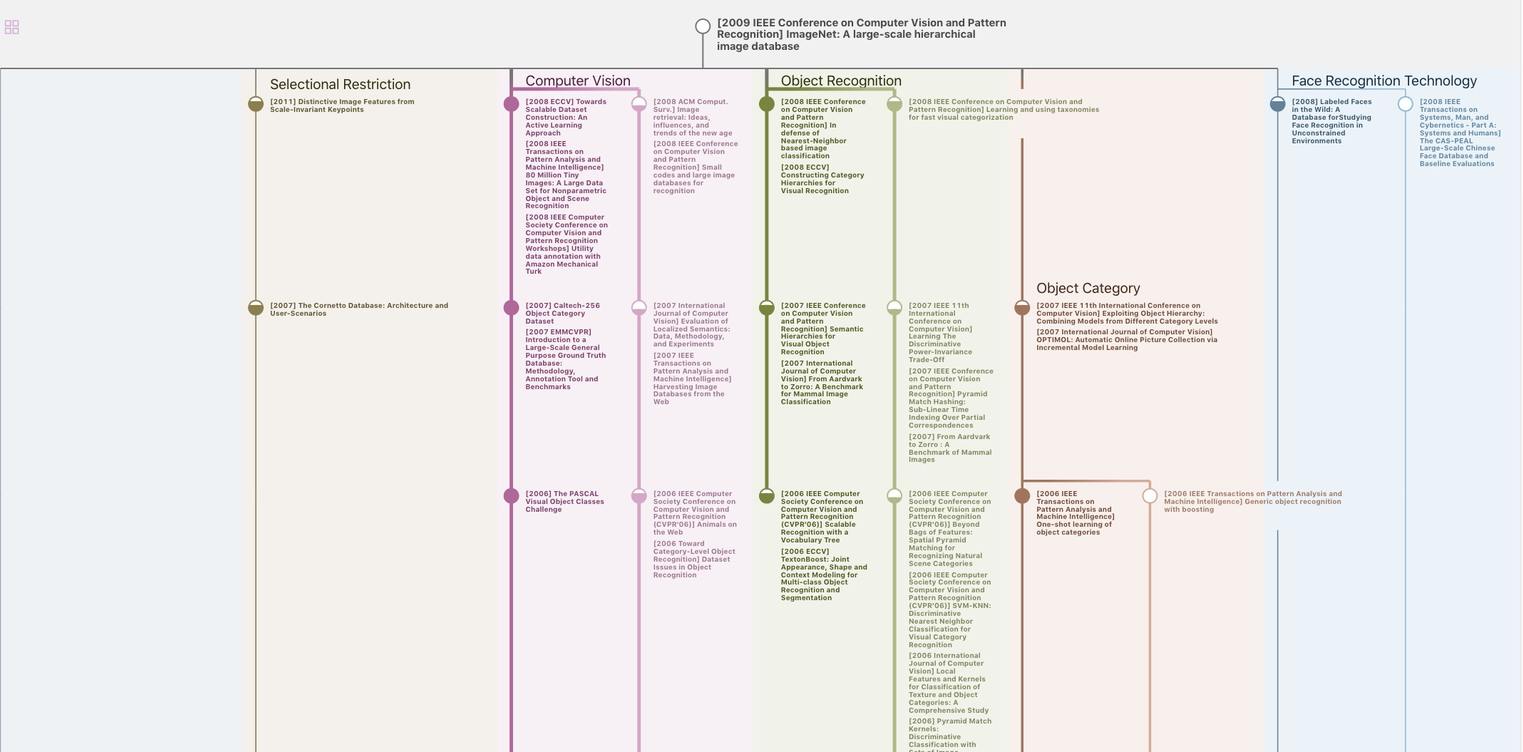
生成溯源树,研究论文发展脉络
Chat Paper
正在生成论文摘要