Time Synchronization-Aware Edge-End Collaborative Network Routing Management for FL-Assisted Distributed Energy Scheduling.
ICC(2023)
摘要
Federated learning (FL)-assisted model training plays an important role in distributed energy scheduling of smart park. However, the time synchronization error between edge and end sides and the adversarial routing competition cause poor accuracy and high delay of model training. In this paper, we address this challenge and propose a time synchronization-aware edge-end collaborative deep Q network-based routing management algorithm named TSA-RM. TSA-RM minimizes the weighted sum of model training loss function and delay via routing optimization. TSA-RM achieves time synchronization awareness and avoids adversarial competition by incorporating time synchronization related information in state space construction and relay selection related information in penalty function design. Simulation results verify the superior performance of TSA-RM in terms of global loss function, model training delay, and time synchronization error compared with two state-of-the-art algorithms.
更多查看译文
关键词
adversarial competition,adversarial routing competition cause poor accuracy,federated learning-assisted model training,FL-assisted distributed energy scheduling,management algorithm,model training delay,model training loss function,routing optimization,time synchronization awareness,time synchronization error,time synchronization related information,time synchronization-aware edge-end collaborative deep Q network,time synchronization-aware edge-end collaborative network routing management,TSA-RM minimizes
AI 理解论文
溯源树
样例
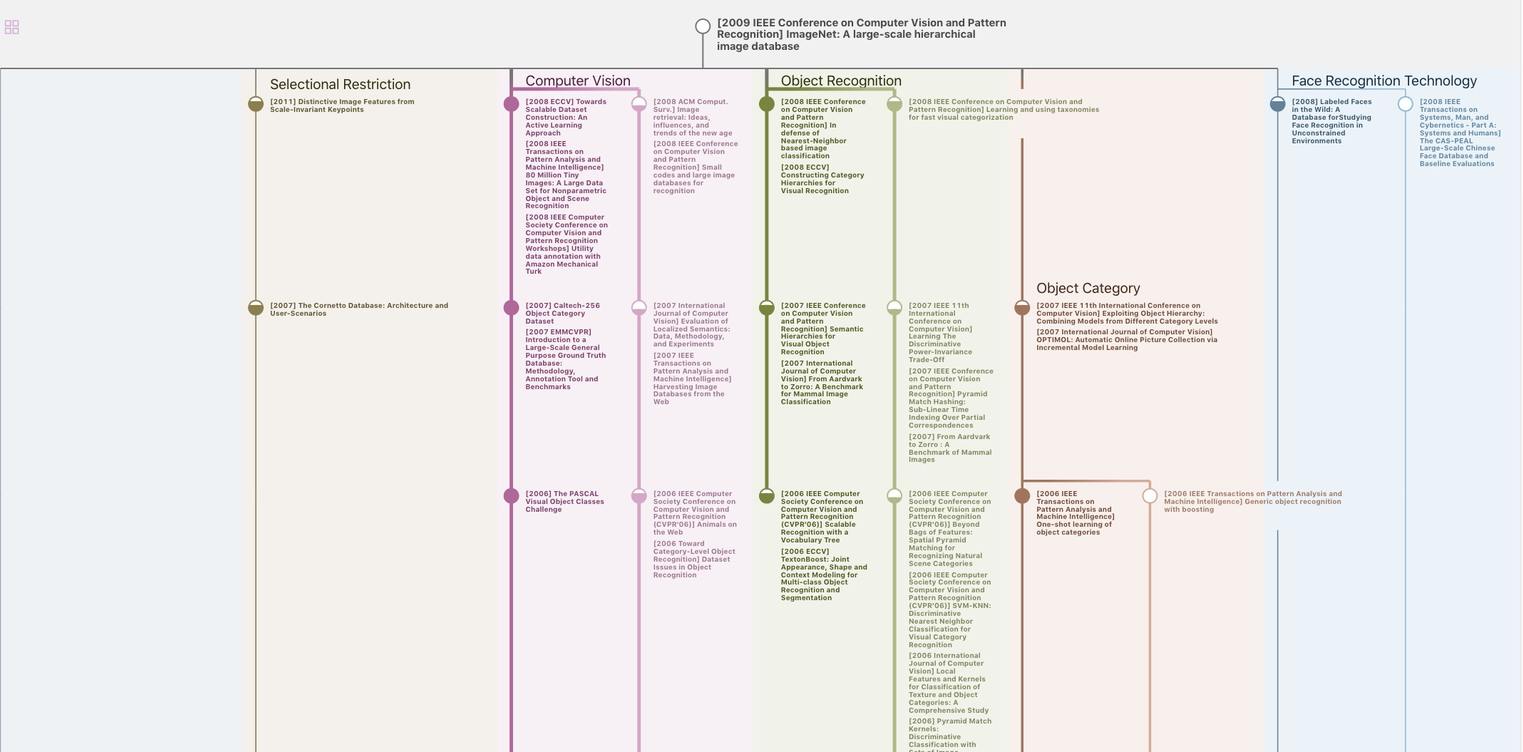
生成溯源树,研究论文发展脉络
Chat Paper
正在生成论文摘要