Towards Efficient Task Offloading at the Edge based on Meta-Reinforcement Learning with Hybrid Action Space
ICC 2023 - IEEE INTERNATIONAL CONFERENCE ON COMMUNICATIONS(2023)
摘要
As a critical concern of multi-access edge computing (MEC), task offloading has received extensive attention. Although deep reinforcement learning (DRL) has achieved great success in resolving the task offloading problem, most existing DRL-based offloading schemes only consider either continuous action space or discrete action space, which results in the loss of optimality of decisions. Moreover, the generalization ability of the existing schemes is still far from adaptive to dynamic changes in the environment. This leads to offloading strategies having to conduct re-sampling and re-training, which largely impairs the offloading efficiency. To address these issues, we propose a novel efficient MEC task offloading scheme based on parameterized meta-reinforcement learning taking hybrid action space into account. We first formulate this problem as a non-convex multi-objective optimization problem. Then, we design a parameterized meta-reinforcement learning algorithm, named Meta-Hybrid-PPO, with hybrid action space to solve the optimization problem. Comprehensive experimental results show that our Meta-Hybrid-PPO not only performs better than existing state-of-the-art methods in reducing task processing latency and computational energy consumption but also achieves better adaptability.
更多查看译文
关键词
Edge Computing,Task Offloading,Deep Reinforcement Learning,Meta-learning
AI 理解论文
溯源树
样例
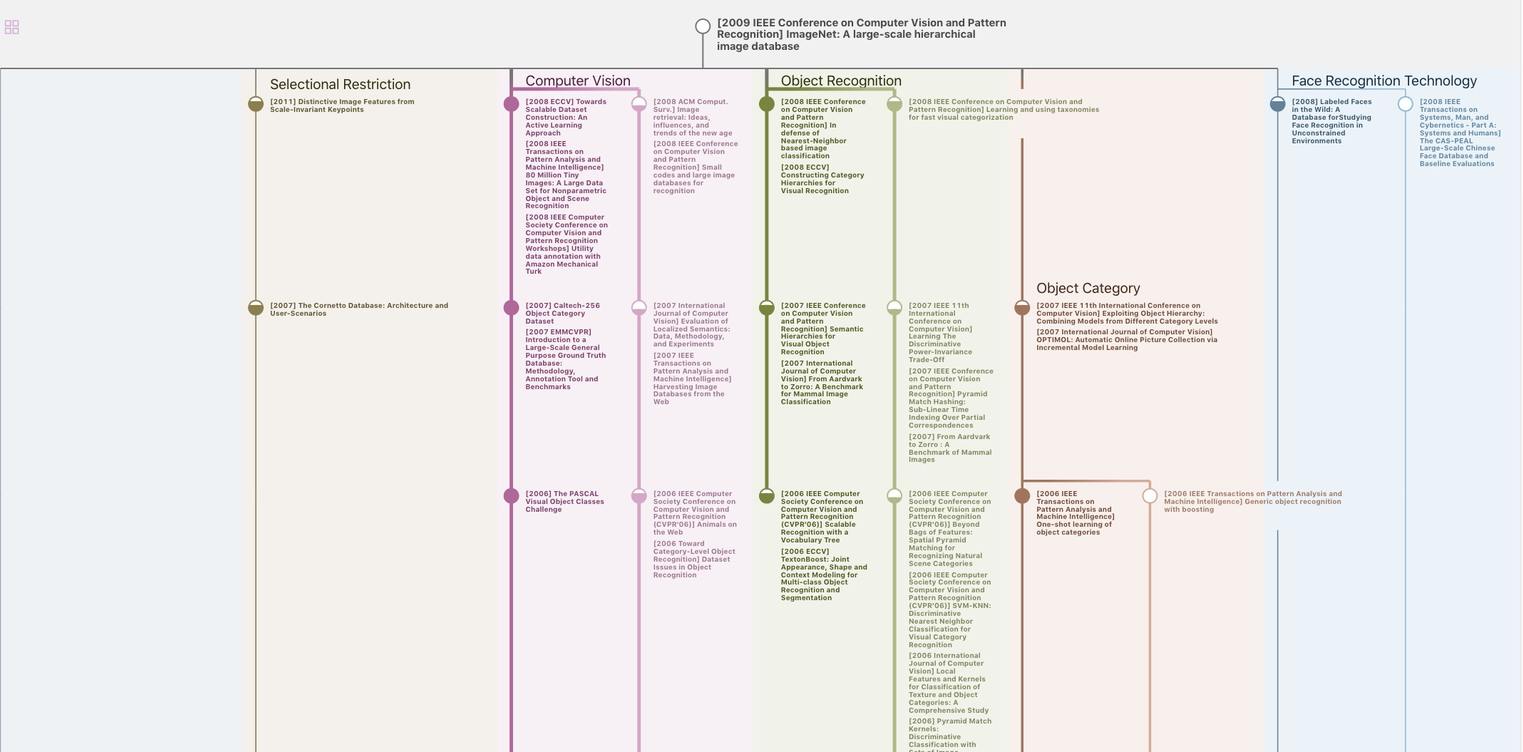
生成溯源树,研究论文发展脉络
Chat Paper
正在生成论文摘要