TUNE: Transfer Learning in Unseen Environments for V2X mmWave Beam Selection
ICC 2023 - IEEE INTERNATIONAL CONFERENCE ON COMMUNICATIONS(2023)
摘要
The use of non-RF data can potentially speed up millimeter wave-band sector-steering in vehicular mobility scenarios by gaining contextual knowledge of the environment. While several works have demonstrated the benefits of this approach, especially applying machine learning models on inputs from LiDAR and image sensors, adapting such models in 'unseen' environments remains an open problem. State-of-the-art techniques generally use a single, pre-trained model for all different scenarios, which assumes that the network has 'seen' representative examples of all future scenarios. In this paper, we propose the TUNE framework, which solves this problem by: (a) transfer learning (TL) for better performance with similar convergence times in comparison to non-TL-generated model testing, (b) utilizing statistical properties to select the best-suited starting 'seen' scenario (and by extension the model trained for it), and (c) a refinement of the transfer learning framework by dynamically selecting the most pertinent layers for retaining, thus reducing the overhead compared to fully retraining a model. We validate TUNE on publicly available synthetic and real-world datasets for mmWave beam selection for V2X communication, revealing that TUNE generally outperforms non-TL methods in a variety of tasks where a different number of beams is available between the training and testing environments.
更多查看译文
关键词
Transfer Learning,Multimodal Data,mmWave,Beam Selection
AI 理解论文
溯源树
样例
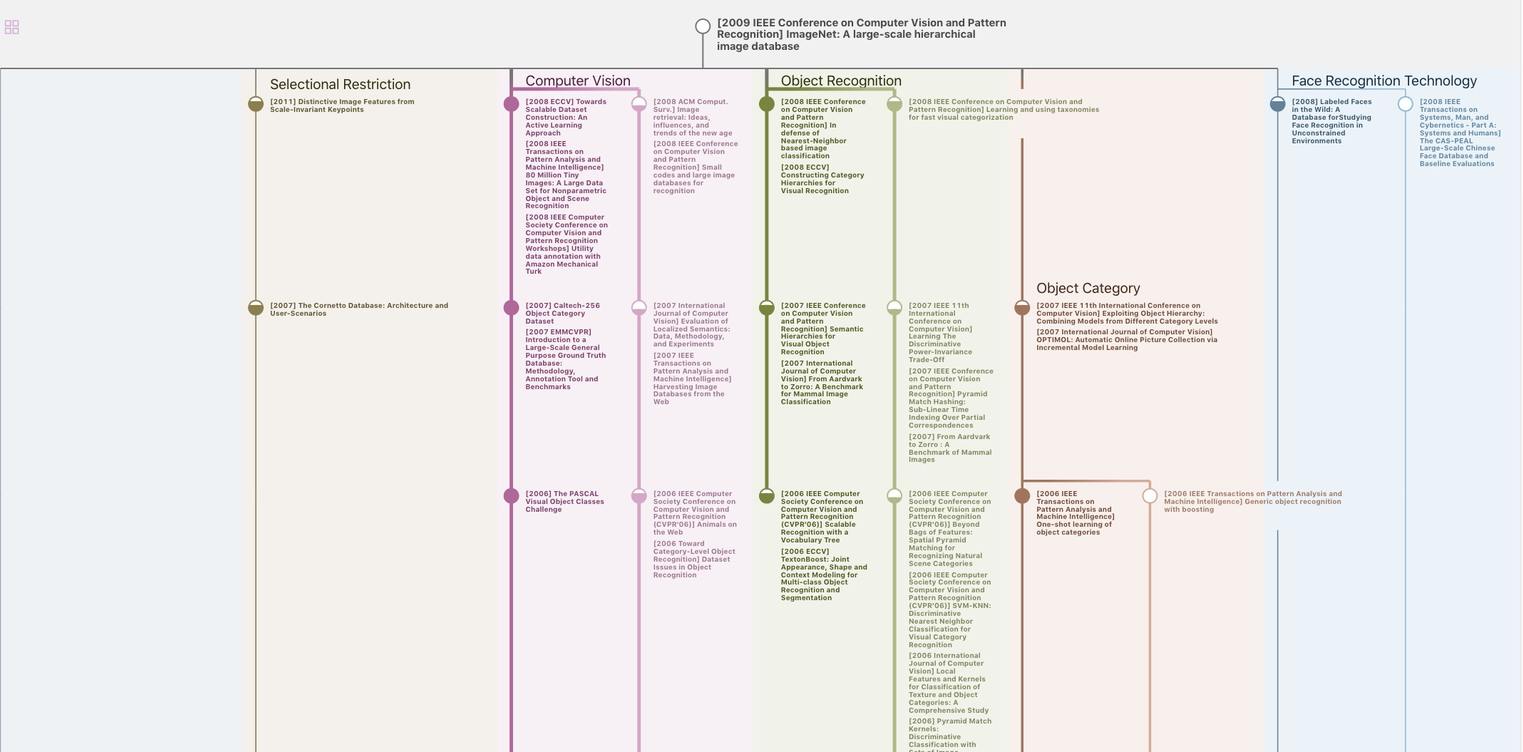
生成溯源树,研究论文发展脉络
Chat Paper
正在生成论文摘要