Novel ODDM Signal Detection using Contrastive Learning for High Reliability and Fast Convergence
ICC 2023 - IEEE INTERNATIONAL CONFERENCE ON COMMUNICATIONS(2023)
摘要
Orthogonal delay-Doppler division multiplexing (ODDM) modulation was recently proposed as a promising solution for high-mobility communication systems. To achieve the potential of ODDM, reliable signal detection is essential, hence, in this work, we propose a contrastive learning-based signal detection approach for ODDM systems, named CL-ODDM. Unlike the conventional deep learning-based methods which focus on positive samples alone, we creatively leverage contrastive learning to exploit both positive and negative samples in the training dataset. By doing so, more distinguishable information of signals can be captured and extracted, contributing to reliable detection results. Moreover, we employ a convolutional neural network and recurrent encoder-decoder (CREN) to represent the comprehensive properties and features of ODDM signals. In addition, an adaptive correction method (ACM) is proposed to increase the convergence rate and improve the stability of the detection model. Extensive simulation results validate that the proposed CL-ODDM is significantly superior state-of-the-art related work, regarding the detection accuracy and convergence rate.
更多查看译文
关键词
ODDM,deep learning,signal detection,contrastive learning
AI 理解论文
溯源树
样例
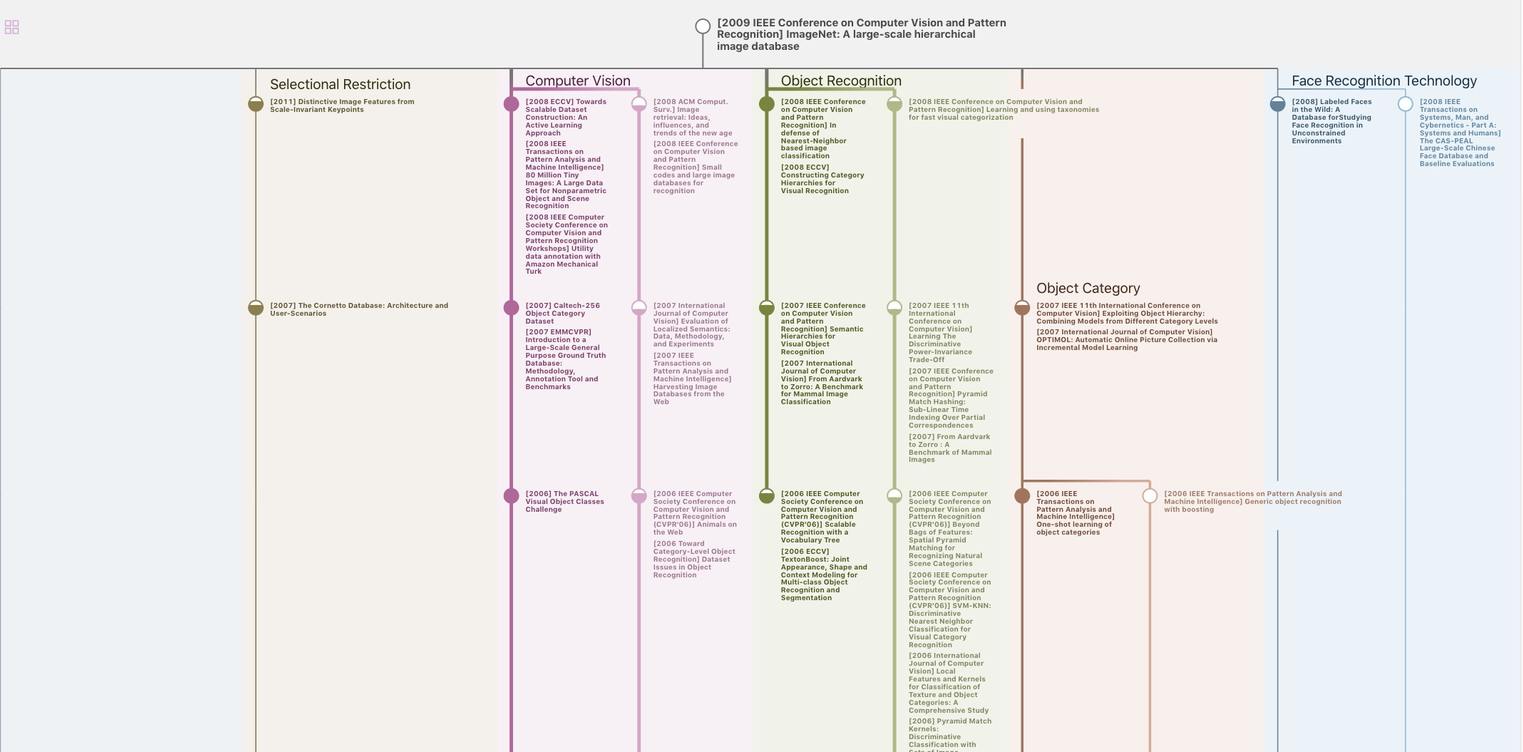
生成溯源树,研究论文发展脉络
Chat Paper
正在生成论文摘要