Non-Inducible RF Fingerprint Hiding via Feature Perturbation
ICC 2023 - IEEE INTERNATIONAL CONFERENCE ON COMMUNICATIONS(2023)
摘要
Machine learning mechanisms are applied to detect the unique characteristics of the wireless interface or signaler that can distinguish one device's signal pattern from the others, which has been widely researched as the fingerprint for user identification. However, such fingerprinting can also be used for malicious purposes, i.e., identification tracking, undesired positioning, etc., as the unique features of the radio signal from a device is determined at the manufacturing stage and often cannot be easily removed afterward. To prevent privacy leakage from such radio frequency (RF) fingerprinting, in this paper, we propose an adversarial mechanism to hide the fingerprint whereby the device's identification cannot be induced by machine learning models from the preamble. Specially, we apply the adversarial attack method to attack the fingerprinting model by adding optimized adversarial perturbation to the preamble that can mislead the model classification results. To alleviate the adversarial sample's impact on communications and ensure the execution of packet detection at receivers, we improve the identification protection strategy with sparse perturbed features. In order to prevent further fingerprinting of re-training over the perturbed RF feature, we extend our method with the time-varying perturbations to further hide the device's identity. Extensive experiments are conducted, and we show that the proposed method can effectively hide the device identification from both the dedicated fingerprint model and the re-trained one from perturbed signals without disturbing the preamble functionality, which provides a gratifying confirmation of the proposed method.
更多查看译文
关键词
RF fingerprinting,Privacy protection,Physical-layer security,Adversarial attack
AI 理解论文
溯源树
样例
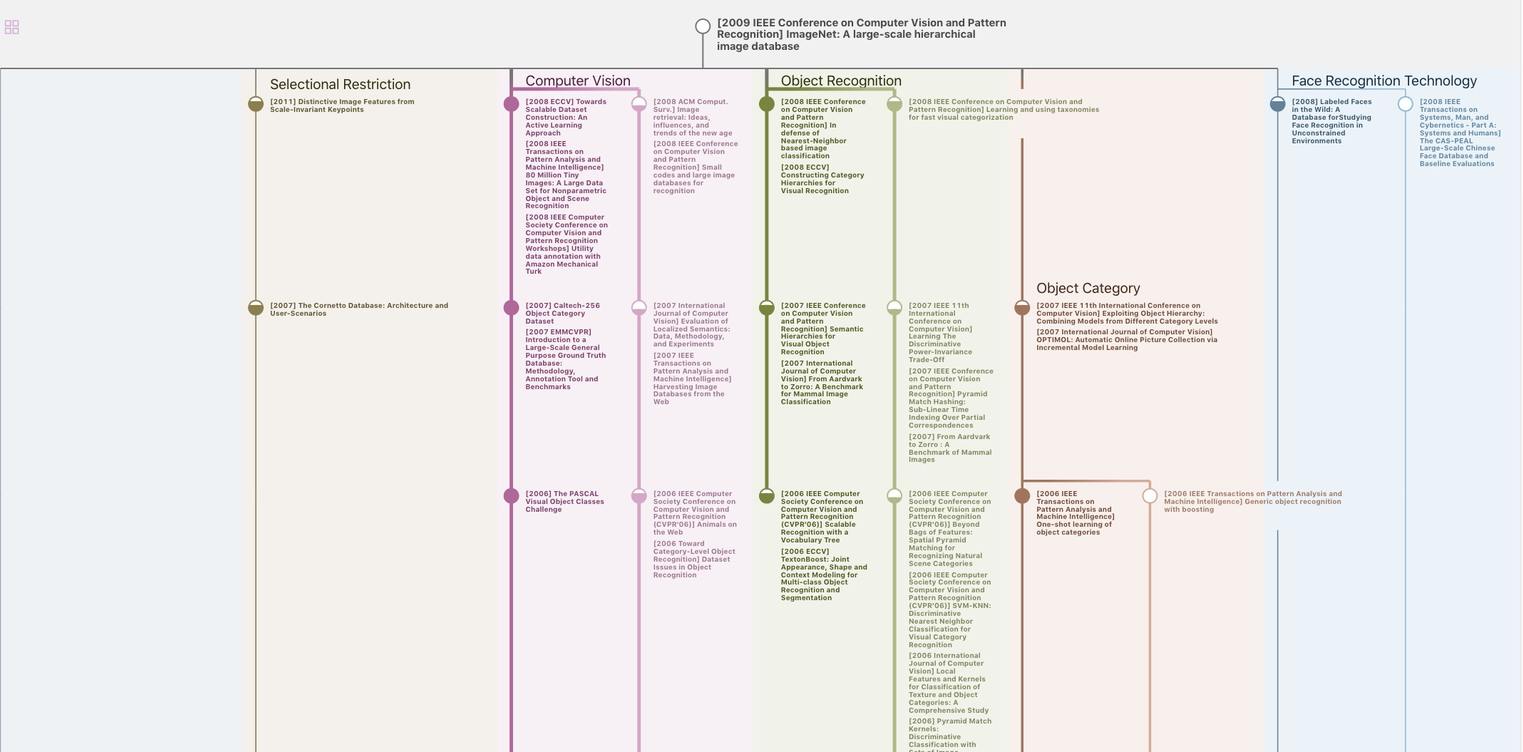
生成溯源树,研究论文发展脉络
Chat Paper
正在生成论文摘要