TransUNet-Lite: A Robust Approach to Cell Nuclei Segmentation.
ICMHI(2023)
摘要
Deep convolutional neural networks have demonstrated superior performance in a variety of vision tasks. For biomedical applications, these methods suffer from problems such as predicting reliable segmentation masks for variable size input images, insufficient data and imbalanced datasets. This paper introduces an efficient and lightweight TransUNet, termed as TransUNet-Lite, that exploits rich feature representations produced by the convolution-based feature extractor, an external attention module instead of conventional self-attention, a fast token selector module, and skip connections from the feature extractor to the decoder to provide lost rich contextual information. The proposed network takes patches as input rather than resized images that fail to care for the original aspect ratio. For the nuclei segmentation task on the 2018 Science Bowl dataset, our TransUNet-Lite outperformed other SOTA networks, with the highest DSC of 93.08% and IoU of 87.95%. The results of our experiments provide insight into the impact of certain network design decisions. By configuring a transformer in a simplistic and efficient manner, it is possible to achieve segmentation quality that is at least equal to SOTA network architectures.
更多查看译文
AI 理解论文
溯源树
样例
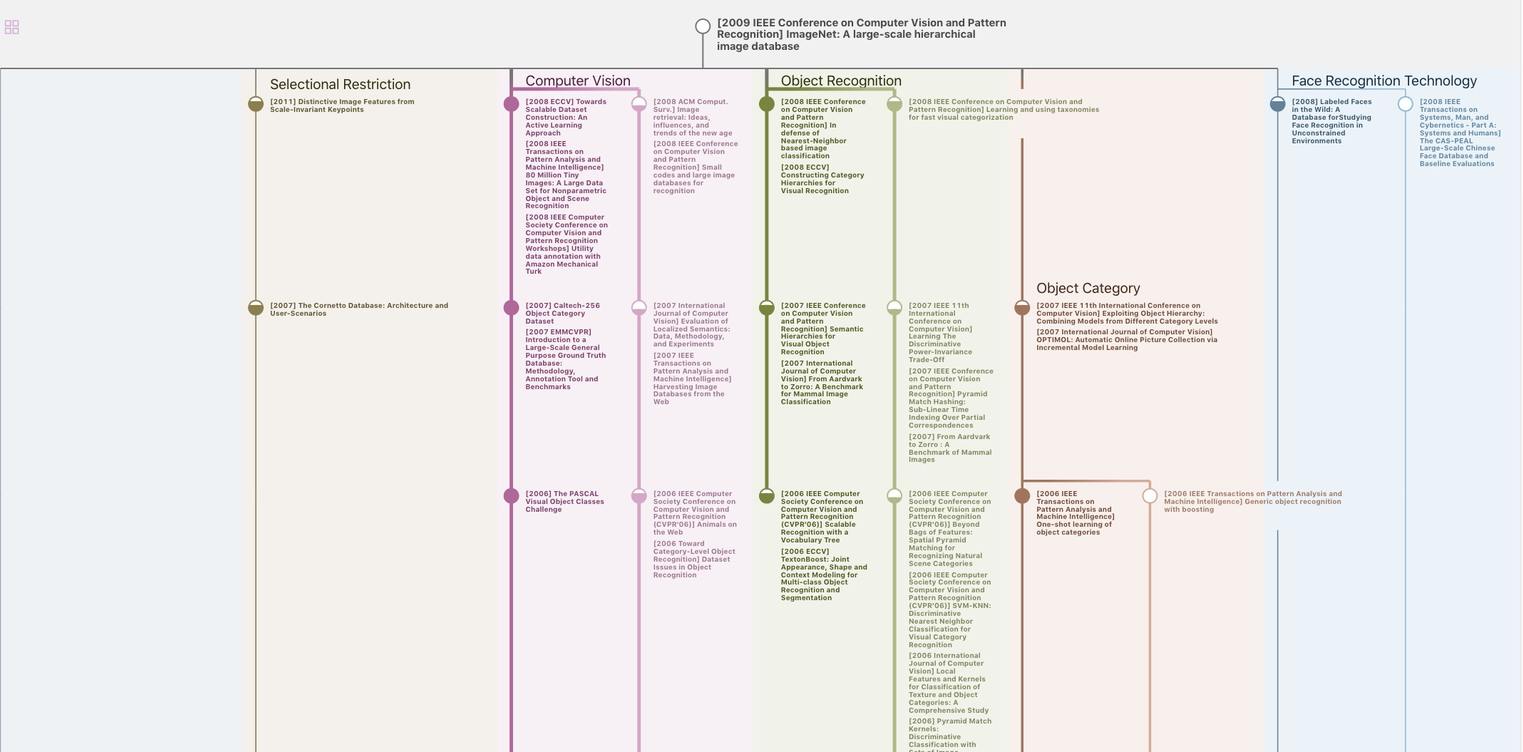
生成溯源树,研究论文发展脉络
Chat Paper
正在生成论文摘要