Enhancing Predictive Battery Maintenance Through the Use of Explainable Boosting Machine.
NiDS (2)(2023)
摘要
Battery Remaining Useful Life (RUL) prediction is crucial for the predictive maintenance of lithium-ion batteries. This paper presents a study on applying an Explainable Boosting Machine (EBM) for RUL prediction of lithium-ion batteries. EBM is a machine learning technique that combines the benefits of gradient boosting and rule-based systems, making it highly interpretable and suitable for applications in safety-critical domains. We evaluated the performance of EBM compared to other machine learning techniques and demonstrated its superiority in accuracy, interpretability, and robustness. The results show that EBM can accurately predict the RUL of lithium-ion batteries; the interpretability of EBM provides insights into the factors that affect battery RUL and enables a better understanding of battery degradation mechanisms. The proposed method highlights the potential of EBM for battery RUL prediction and guarantees the secure and reliable functioning of batteries.
更多查看译文
关键词
predictive battery maintenance,explainable boosting machine
AI 理解论文
溯源树
样例
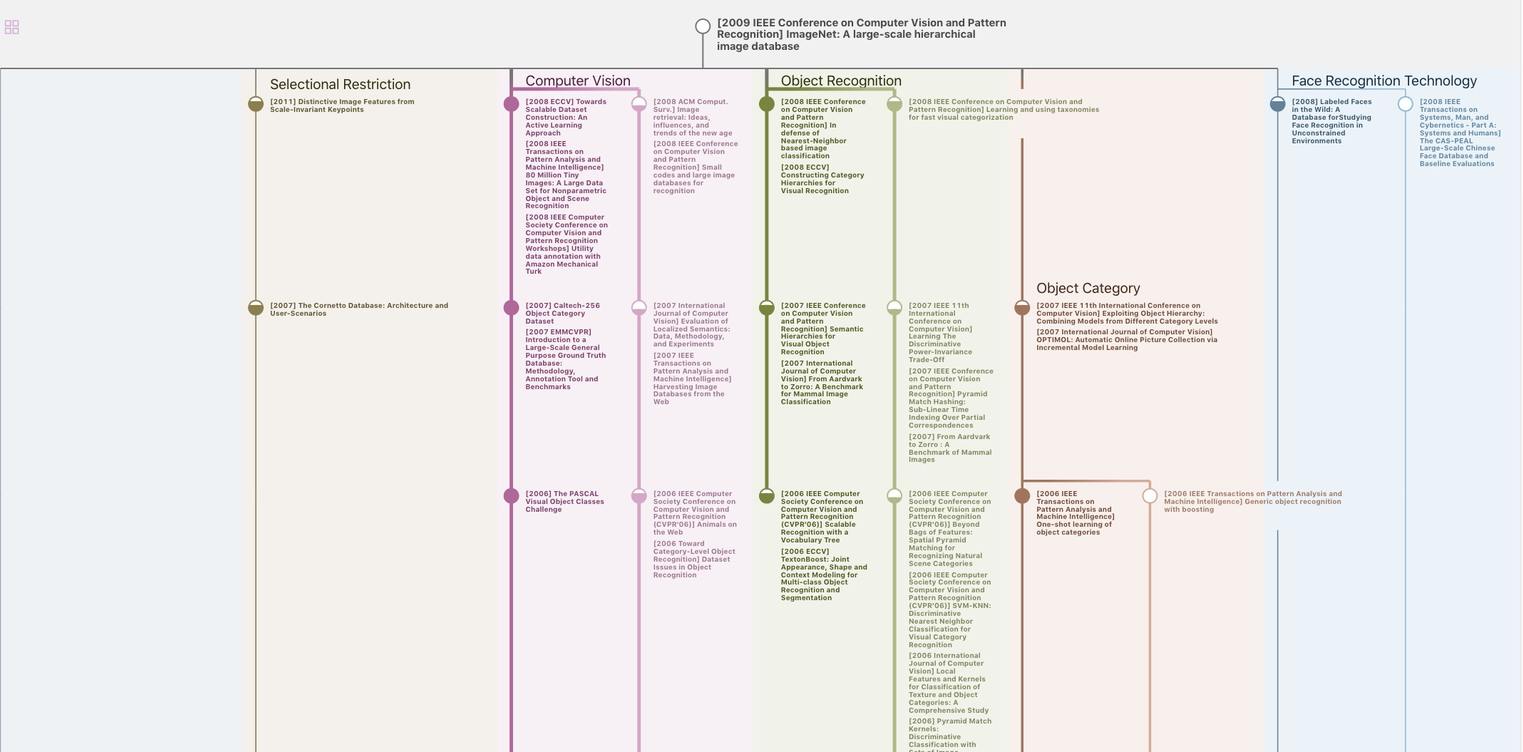
生成溯源树,研究论文发展脉络
Chat Paper
正在生成论文摘要