Deformable Medical Image Registration Under Distribution Shifts with Neural Instance Optimization
MACHINE LEARNING IN MEDICAL IMAGING, MLMI 2023, PT I(2024)
摘要
Deep-learning deformable image registration methods often struggle if test-image characteristic shifts from the training domain, such as the large variations in anatomy and contrast changes with different imaging protocols. Gradient descent-based instance optimization is often introduced to refine the solution of deep-learning methods, but the performance gain is minimal due to the high degree of freedom in the solution and the absence of robust initial deformation. In this paper, we propose a new instance optimization method, Neural Instance Optimization (NIO), to correct the bias in the deformation field caused by the distribution shifts for deep-learning methods. Our method naturally leverages the inductive bias of the convolutional neural network, the prior knowledge learned from the training domain and the multi-resolution optimization strategy to fully adapt a learning-based method to individual image pairs, avoiding registration failure during the inference phase. We evaluate our method with gold standard, human cortical and subcortical segmentation, and manually identified anatomical landmarks to contrast NIO's performance with conventional and deep-learning approaches. Our method compares favourably with both approaches and significantly improves the performance of deep-learning methods under distribution shifts with 1.5% to 3.0% and 2.3% to 6.2% gains in registration accuracy and robustness, respectively.
更多查看译文
关键词
distribution shifts,optimization
AI 理解论文
溯源树
样例
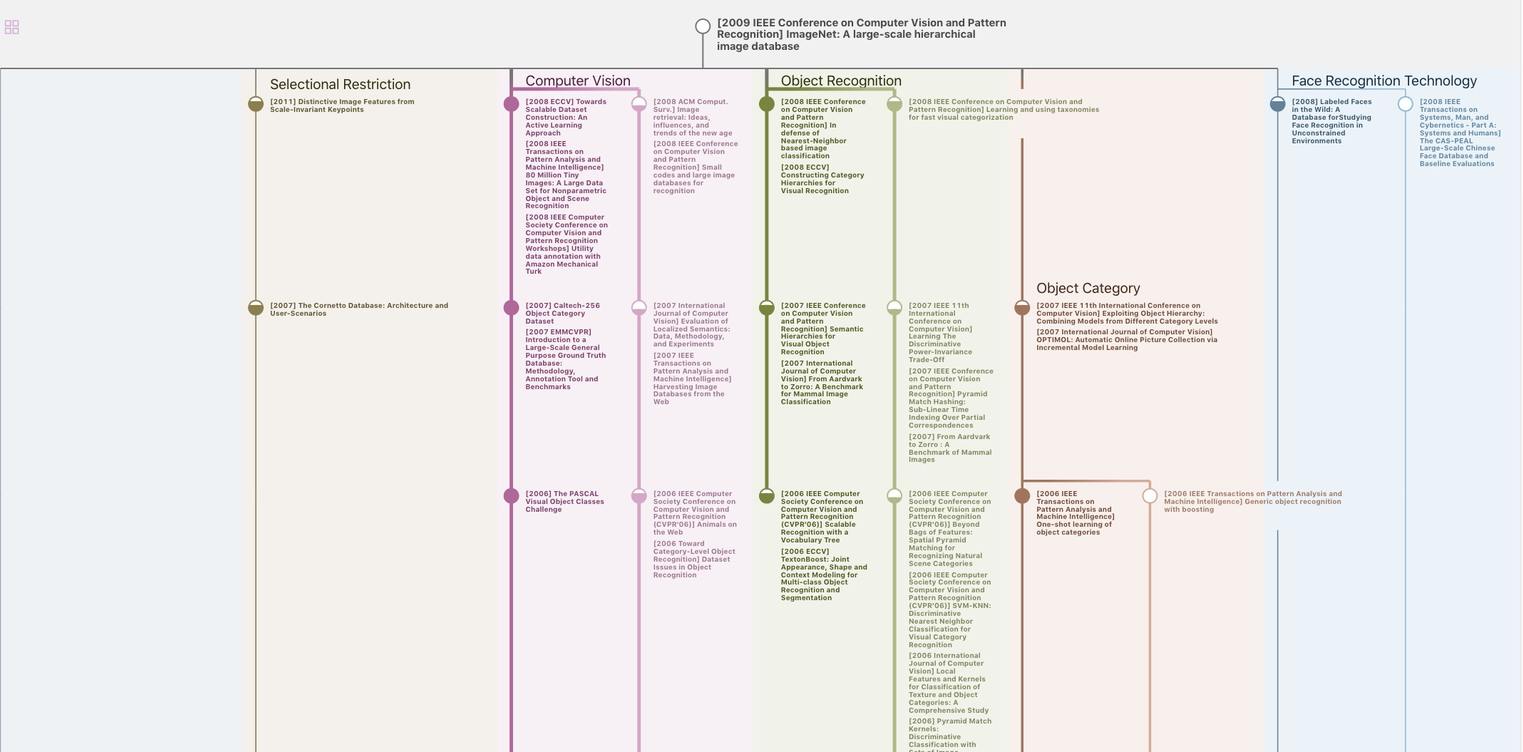
生成溯源树,研究论文发展脉络
Chat Paper
正在生成论文摘要