Collision-Free Trajectory Planning of Mobile Robots by Integrating Deep Reinforcement Learning and Model Predictive Control.
CASE(2023)
关键词
collision avoidance,collision-free guidance,collision-free trajectory planning,computational efficiency,deep Q-learning,integrating deep reinforcement learning,local trajectory,mobile robot,model predictive control,MPC trajectory-tracking framework,real-time collision-free navigation,reference trajectory,solution quality,time efficiency,time steps
AI 理解论文
溯源树
样例
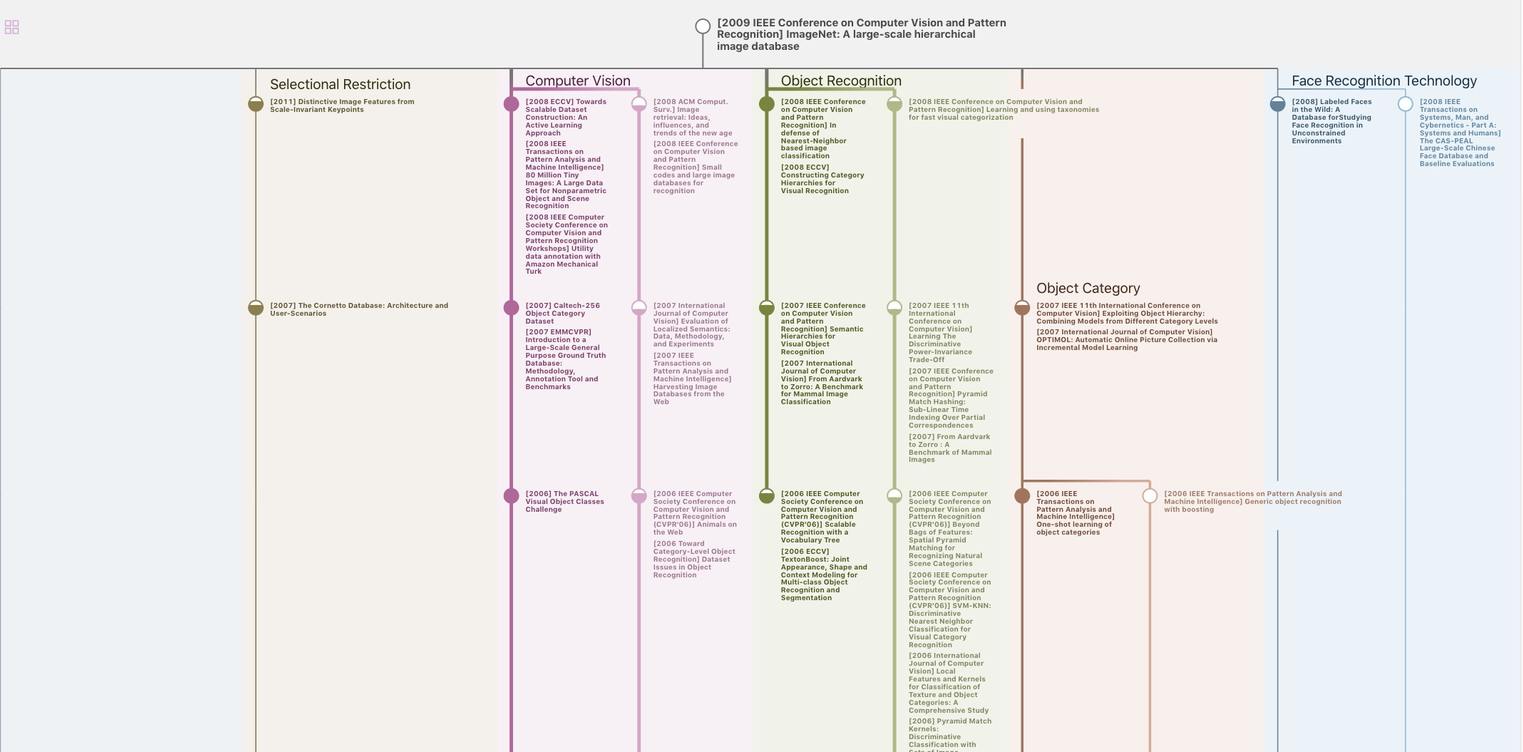
生成溯源树,研究论文发展脉络
Chat Paper
正在生成论文摘要