Calibration-Free Transfer Learning for EEG-Based Cross-Subject Motor Imagery Classification.
CASE(2023)
摘要
Motor imagery based brain-computer interfaces (MI-BCIs) have been widely used in intelligent medical applications such as post-stroke rehabilitation and mobile assistant robots. However, the high inter-subject variability and the non-stationarity of EEG characteristics limit the cross-subject applications of MI-BCIs. Long-term calibration can be used to improve EEG-based performance, but which will cause low efficiency and reduce practicality. To overcome the limitation, data from other subjects can be used for transfer learning to reduce calibration time. Therefore, a calibration-free transfer learning method for EEG-based cross-subject MI classification is proposed in this paper. On one hand, Euclidean alignment and Riemannian alignment are introduced to reduce domain differences. On the other hand, the similarity is calculated by Multiple Kernel-Maximum Mean Discrepancy (MK-MMD) to select appropriate source domain samples, which is followed by domain adversarial training of neural network (DANN) for the final model construction. In order to achieve calibration-free, the new subjects' resting-state data was used only. Extensive experiments were conducted on BCI competition IV dataset 2a. The results show that the proposed method can achieve 75.96% classification accuracy without using subjects' labeled data, which demonstrates the feasibility of the proposed method in calibration time reduction and classification accuracy improvement.
更多查看译文
关键词
Calibration-free,transfer learning,motor imagery,cross-subject,EEG
AI 理解论文
溯源树
样例
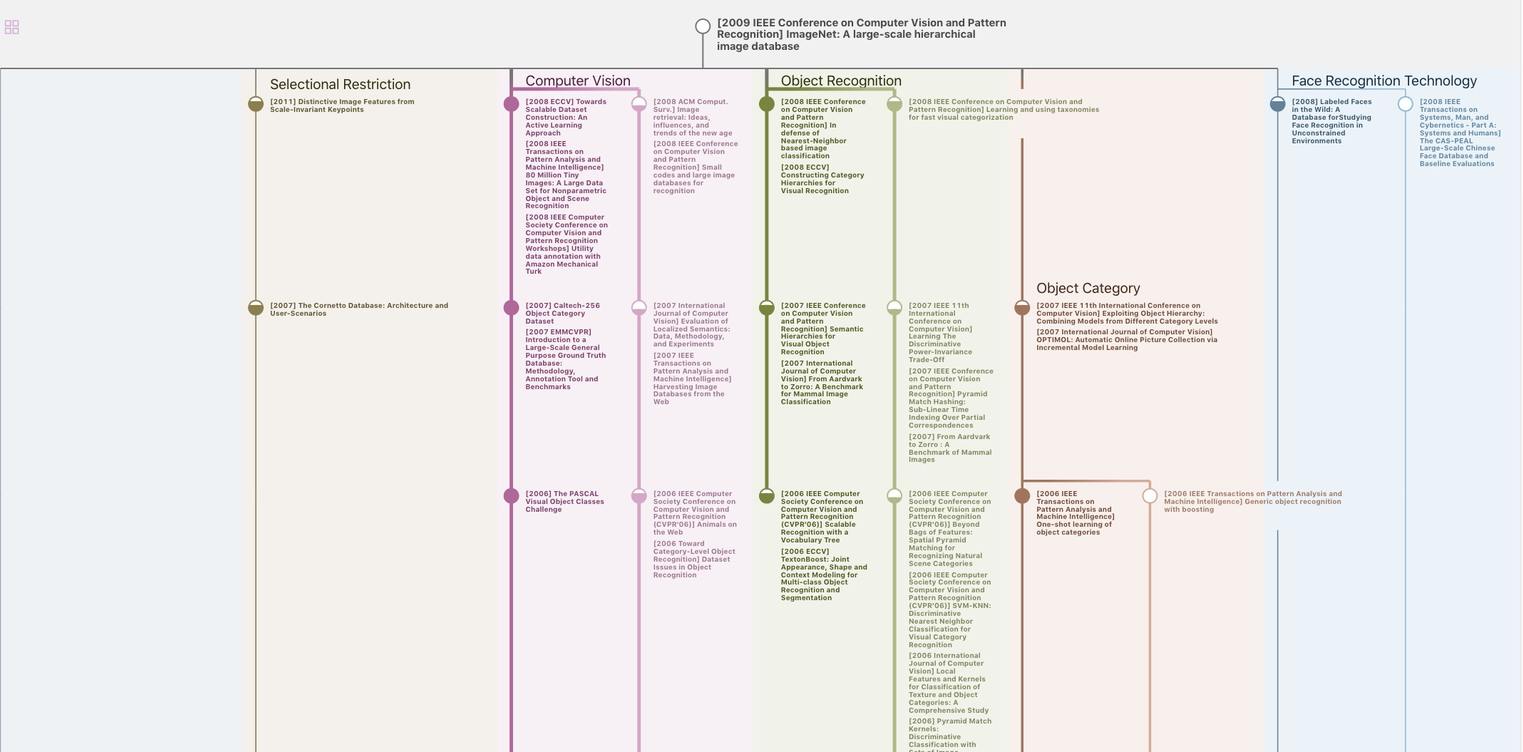
生成溯源树,研究论文发展脉络
Chat Paper
正在生成论文摘要