Predicting Age from Human Lung Tissue Through Multi-modal Data Integration.
DS(2023)
摘要
The accurate prediction of biological age can bring important benefits in promoting therapeutic and behavioural strategies for healthy aging. We propose the development of age prediction models using multi-modal datasets, including transcriptomics, methylation and histological images from lung tissue samples of 793 human donors. From a technical point of view this is a challenging problem since not all donors are covered by the same data modalities and the datasets have a very high feature dimensionality with a relatively smaller number of samples. To fairly compare performance across different data types, we’ve created a test set including donors represented in each modality. Given the unique characteristics of the data distribution, we developed gradient boosting tree and convolutional neural network models for each dataset. The performance of the models can be affected by several covariates, including smoking history, and, most importantly, by a skewed distribution of age. Data-centric approaches, including feature engineering, feature selection, data stratification and resampling, proved fundamental in building models that were optimally adapted for each data modality, resulting in significant improvements in model performance for imbalanced regression. The models were then applied to the test set independently, and later combined into a multi-modal ensemble through a voting strategy, predicting age with a median absolute error of 4 years. Even if prediction accuracy remains a challenge, in this work we provide insights to address the difficulties of multi-modal data integration and imbalanced data prediction.
更多查看译文
关键词
human lung tissue,lung tissue,data,multi-modal
AI 理解论文
溯源树
样例
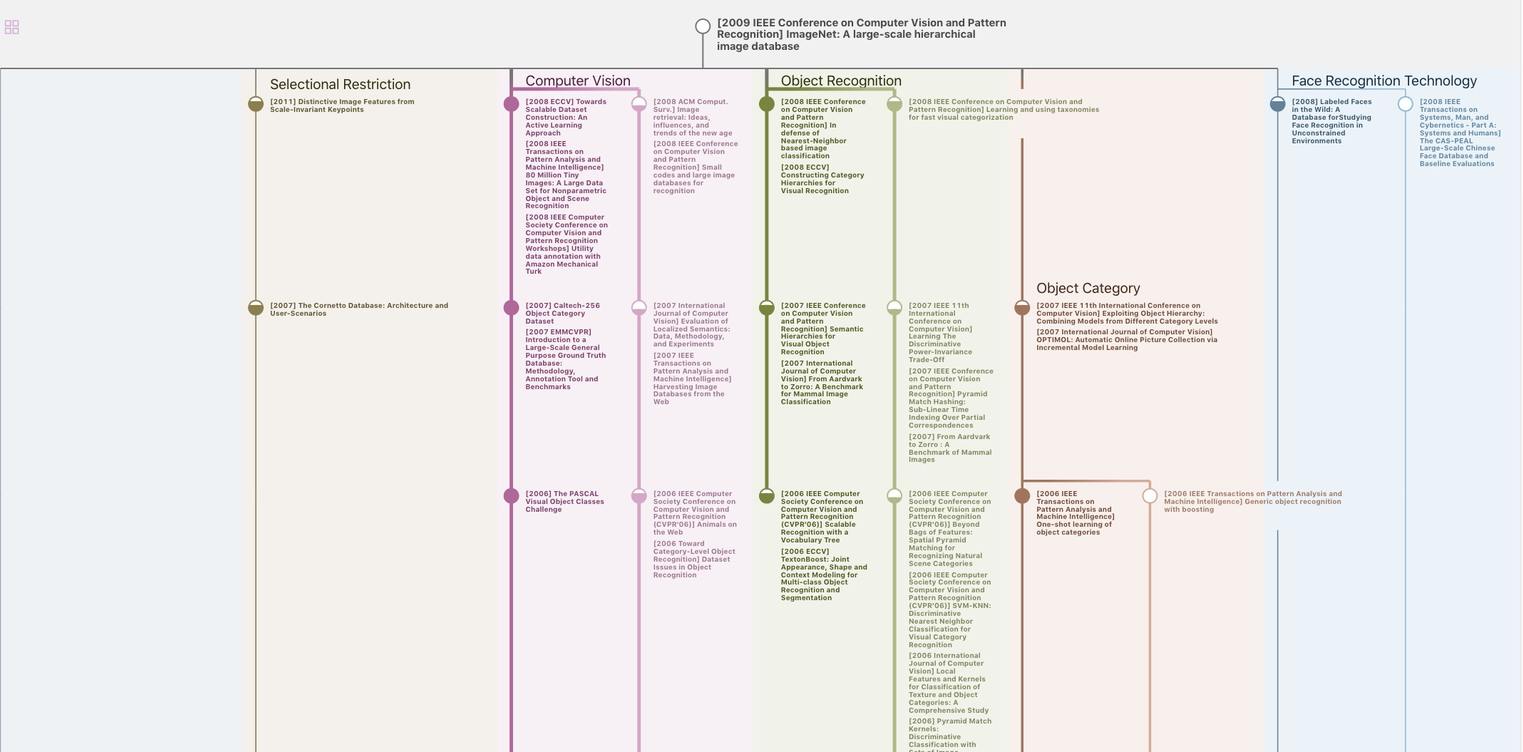
生成溯源树,研究论文发展脉络
Chat Paper
正在生成论文摘要