Refining Temporal Visualizations Using the Directional Coherence Loss.
DS(2023)
摘要
Many real-world data sets contain a temporal component or include transitions from state to state. For exploratory data analysis, we can present these high-dimensional data sets in two-dimensional maps, using embeddings of data objects under exploration and representing their temporal relations with directed edges. Most existing dimensionality reduction techniques, such as t-SNE and UMAP, disregard the temporal or relational nature of the data during embedding construction, leading to cluttered visualizations obscuring potentially interesting temporal patterns. To address this issue, we introduce Directional Coherence Loss (DCL), a differentiable loss function that we can incorporate into existing dimensionality reduction techniques. We have designed DCL to highlight the temporal aspects of the data, revealing temporal patterns that might otherwise remain unnoticed. By encouraging local directional coherence of the directed edges, the DCL produces more temporally-meaningful and less-cluttered visualizations. We demonstrate the effectiveness of our approach on a real-world multivariate time-series data set tracking the progression of the COVID-19 pandemic in Slovenia. We show that incorporating the DCL into the t-SNE algorithm elucidates the time progression of the pandemic in the embedding and reveals interesting cyclical patterns otherwise hidden in standard embeddings.
更多查看译文
关键词
visualizations,directional coherence loss
AI 理解论文
溯源树
样例
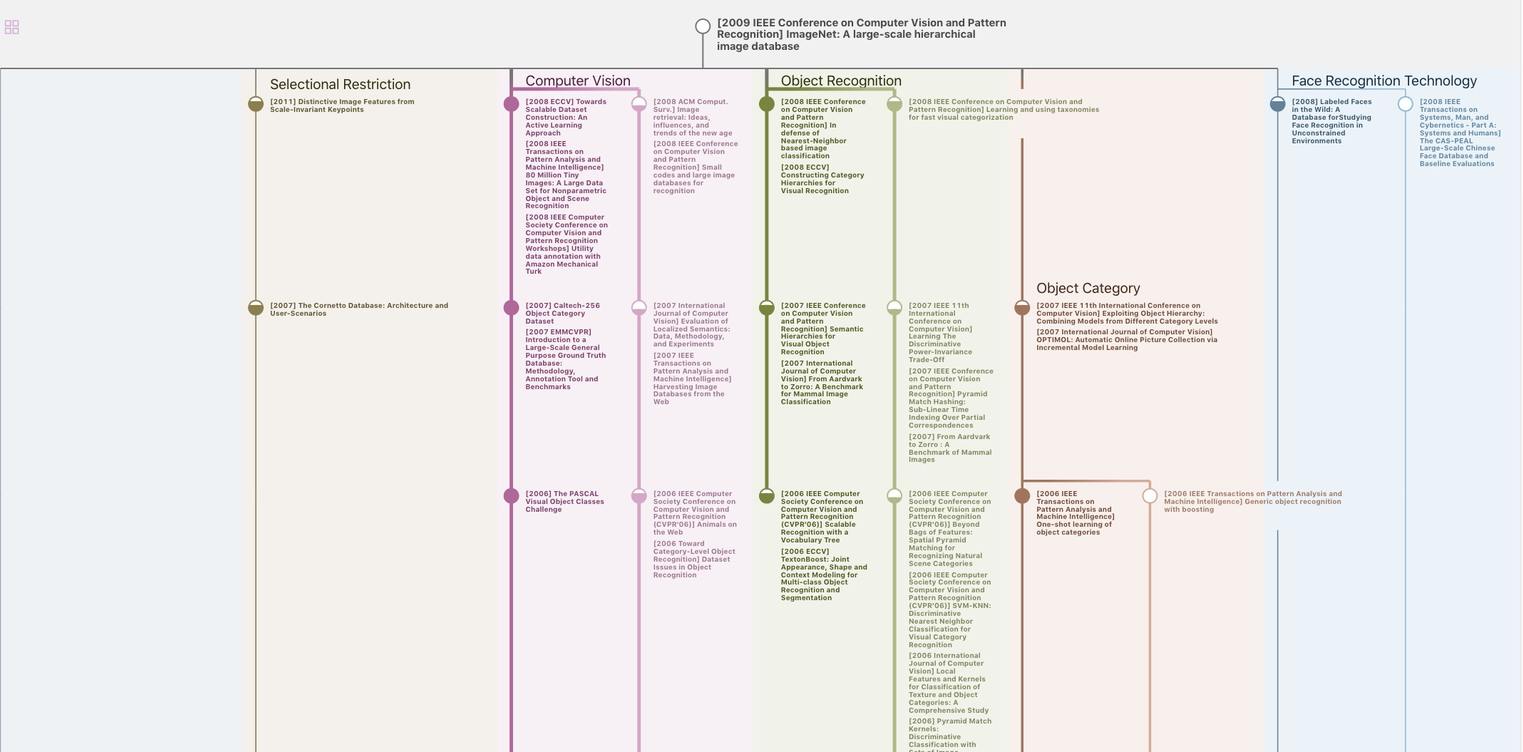
生成溯源树,研究论文发展脉络
Chat Paper
正在生成论文摘要