Studying the Impact of Varying Sample Length of ECG Signal on Classification Accuracy.
ICAC(2023)
摘要
ECG Signals in digital format are being used for gathering various vital information and for predicting diseases in patients using advanced artificial intelligence and digital signal processing technologies. It is important to process the data sufficiently to get the optimal results. In addition to cleaning the dataset, it is important to select the right signal length. This study uses three publicly available datasets (namely autonomic aging dataset, PTB-XL dataset, and PTB Diagnostic dataset) to study the impact of varying sample lengths on classification accuracy i.e., understanding whether varying sample lengths can impact the accuracy and if so how different sample lengths for certain classification problems can affect it. The experiments performed used three machine learning algorithms which are Decision Tree Classifier, Random Forest Classifier, and State Vector Machine Classifier, and it was found that the signal length does impact the accuracy when predicting diseases using an ECG signal, and the behavior can vary depending on the type of data being used and the nature of classification problem, even if the same model is used.
更多查看译文
关键词
machine learning,ecg,disease classification,digital signals,sample length analysis
AI 理解论文
溯源树
样例
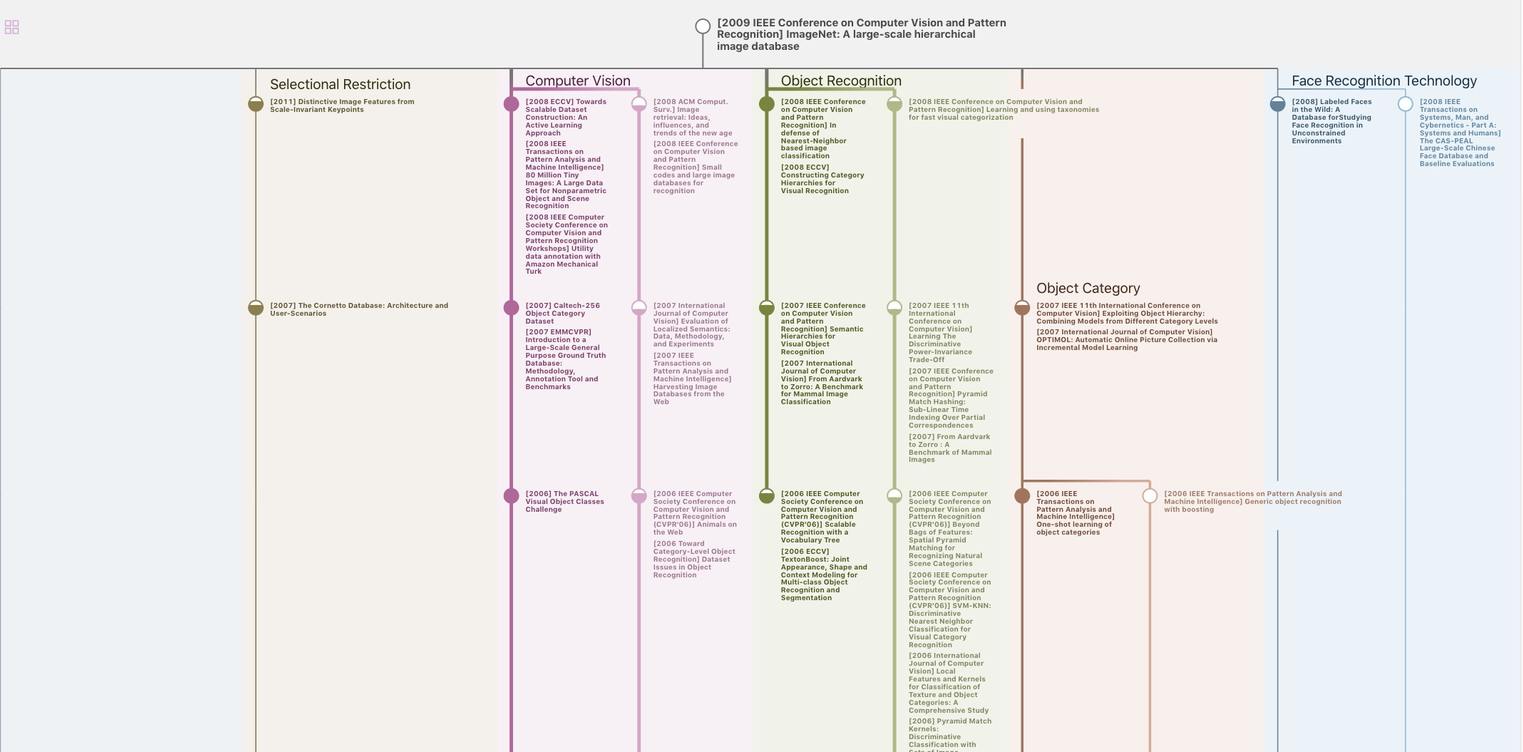
生成溯源树,研究论文发展脉络
Chat Paper
正在生成论文摘要