Robot Learning from Multiple Demonstrations Based on Generalized Gaussian Mixture Model.
ICAC(2023)
摘要
The quality of skills learned by robots from demonstrations depends on the level of demonstration by the instructor, while models such as Dynamic Motion Primitives (DMP) are extremely sensitive to the quality of demonstrations because they can only learn from a single demonstration. In this paper, we propose a method of learning from multiple demonstration. First, we model multiple demonstration data by generalized Gaussian mixture models (GMMs), then generate a smooth trajectory by the Gaussian mixture regression (GMR), and finally, the generated trajectory is modeled by DMP. This method can capture features from multiple demonstrations of the same task, reducing the impact of incorrect demonstrations on skill learning. Additionally, the generalized Gaussian mixture model has strong data fitting ability, enabling robots to learn smooth and high-quality motion skills. Finally, the effectiveness of the proposed method was verified by performing a Chinese character writing task on a Baxter robot.
更多查看译文
关键词
Baxter robot,Chinese character writing task,data fitting ability,DMP,dynamic motion primitives,Gaussian mixture regression,generalized Gaussian mixture model,GMM,GMR,high-quality motion skills,robot learning,skill learning,smooth trajectory generation
AI 理解论文
溯源树
样例
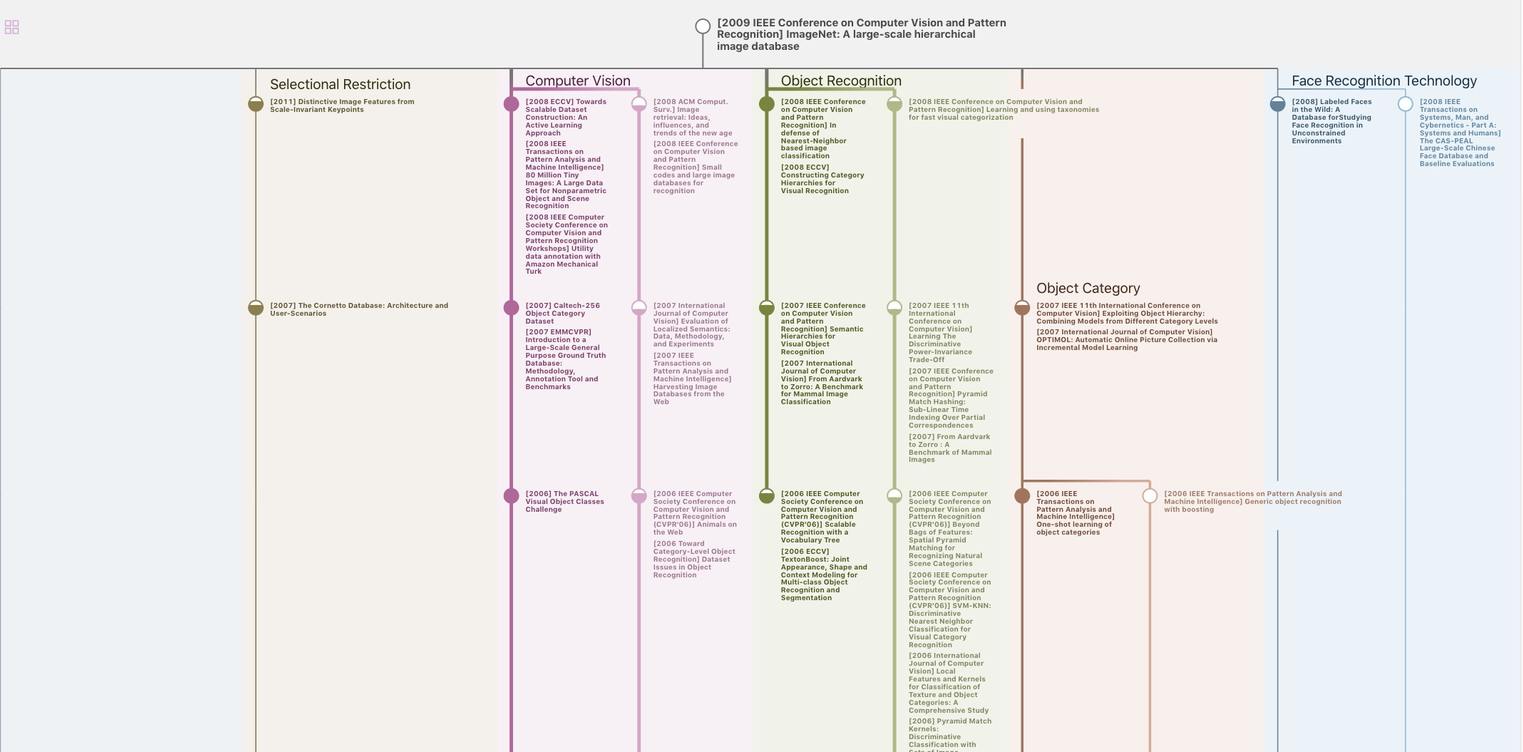
生成溯源树,研究论文发展脉络
Chat Paper
正在生成论文摘要