Application of Machine Learning Algorithms in Cold-Rolled Strip Steel Surface Defect Grading Systems.
ICAC(2023)
摘要
Surface defects in cold-rolled strip steel can have a direct impact on the quality of downstream products and have received an increasing amount of attention recently. While the inspection system can detect and classify individual defects, grading the surface quality of an entire coil of strip necessitates the analysis of additional information. Current strip surface quality inspection research has focused on how to improve the accuracy of surface defect classification, but little study has been conducted on strip surface quality grading. The main factor affecting the accuracy of surface quality grading is aggregated defects, which frequently occur on cold rolling lines. This paper addresses this issue by creating a grading method for surface defects in cold rolled strip steel. Aggregated defects are initially discovered from the defects identified by the inspection system using the Histogram-based Outlier Score (HBOS) algorithm. To produce a rapid and explainable grading model for surface defects, the image feature parameters of the aggregated defects are extracted using the Gray-level Co-occurrence Matrix (GLCM), while the geometry characteristics of these defects are together fed into a decision tree model. Finally, the model's grading results are displayed via the system interface to provide direction on surface quality grading for industrial production, certifying the accuracy and validity of the work.
更多查看译文
关键词
Machine learning,cold-rolled strip steel,image processing,GLCM,decision tree
AI 理解论文
溯源树
样例
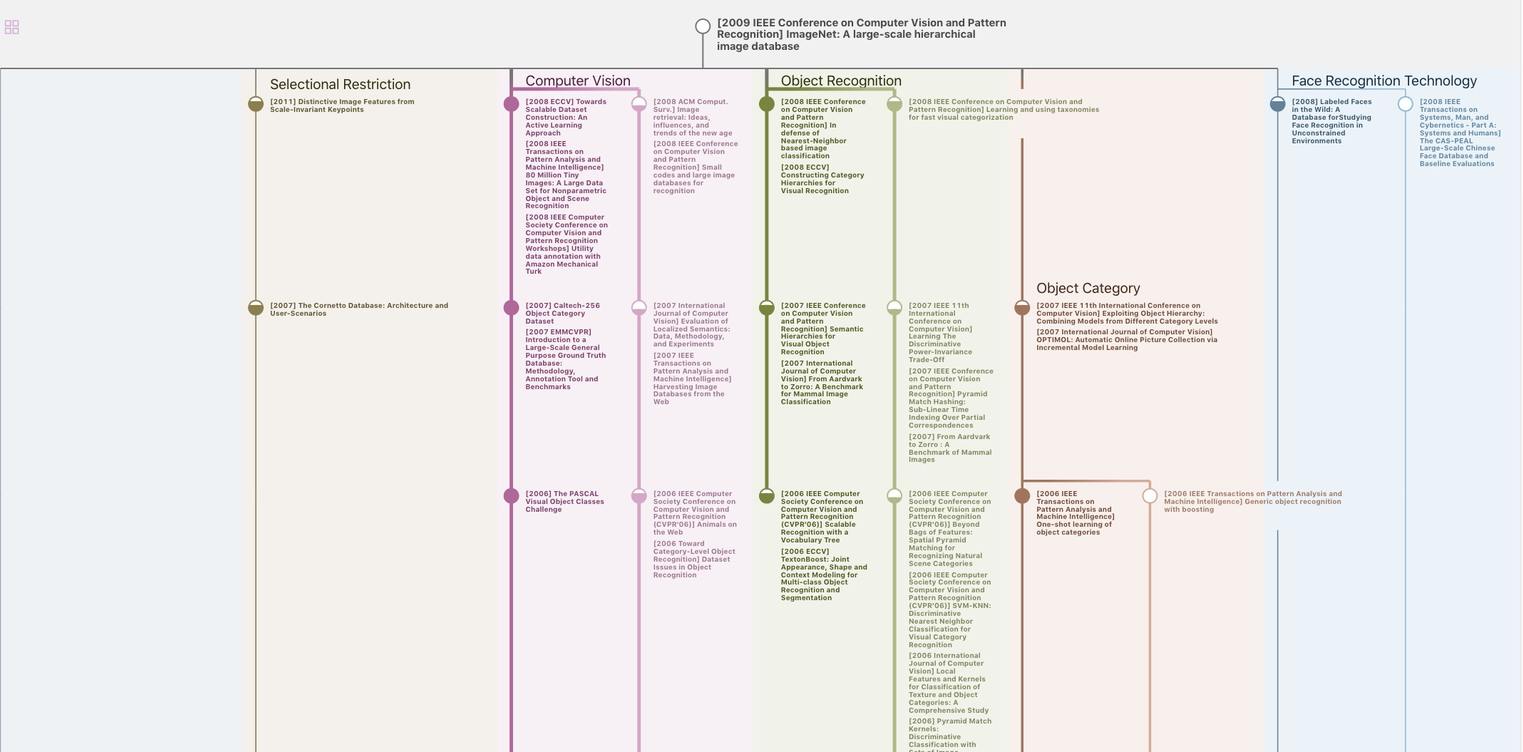
生成溯源树,研究论文发展脉络
Chat Paper
正在生成论文摘要