Fine-Tuned SqueezeNet Lightweight Model for Classifying Surface Defects in Hot-Rolled Steel
ADVANCES IN COMPUTATIONAL INTELLIGENCE, IWANN 2023, PT I(2023)
摘要
The advent of powerful artificial intelligence-based tools is opening up new opportunities to improve the efficiency of processes in the manufacturing industry. One of those processes is visual inspection, where deep learning approaches, particularly convolutional neural networks (CNNs), have achieved human-level defect classification performance. The problem in using CNNs is that training takes a long time and they are computationally expensive. However, in recent years, lightweight models have emerged as an alternative. The most representative lightweight model is SqueezeNet, which can compete with deep CNNs in terms of classification performance with few trainable parameters. In this paper, a SqueezeNet model is used to classify surface defects on hot-rolled steel plates in a public surface defect database. The model is fine-tuned using a grid search algorithm and evaluated by 5-fold cross-validation, achieving an F1-score of 0.96984.
更多查看译文
关键词
Lightweight CNN model,SqueezeNet,Defect classification,Automated inspection systems,Industry 4.0
AI 理解论文
溯源树
样例
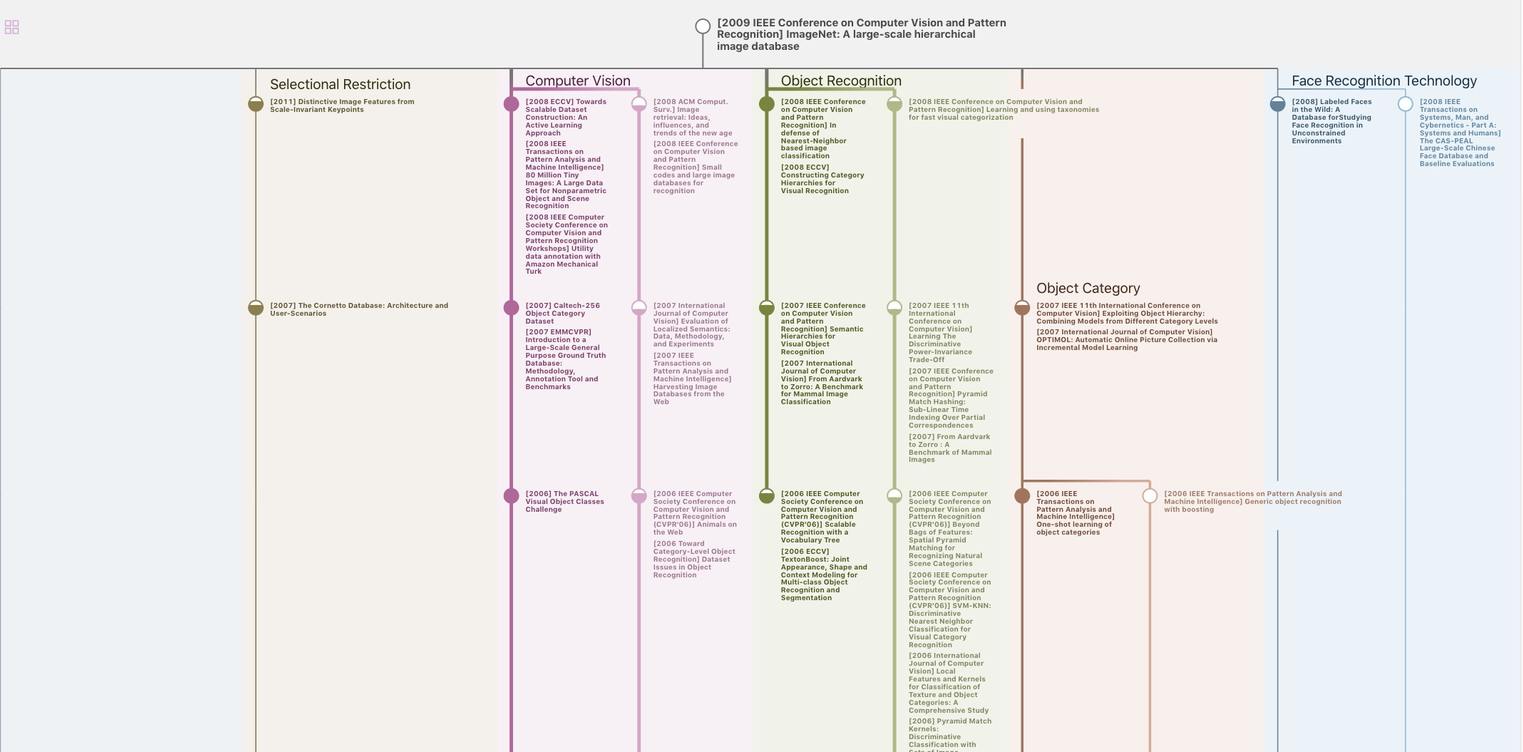
生成溯源树,研究论文发展脉络
Chat Paper
正在生成论文摘要