Deep Learning for the Analysis of Solar Radiation Prediction with Different Time Horizons and Data Acquisition Frequencies
ADVANCES IN COMPUTATIONAL INTELLIGENCE, IWANN 2023, PT I(2023)
摘要
This study aims to develop and compare different AI systems for predicting solar radiation and evaluate their performance across different prediction horizons. Predicting solar radiation is of crucial importance for harnessing renewable energy sources. The models were designed to predict radiation over 6-h and 15-min horizons with the lowest possible error. The impact of prediction horizon and data acquisition frequency on prediction accuracy is discussed, emphasizing the need to consider the number of parameters and training time when comparing models. To improve the accuracy of short-term solar radiation predictions, five deep learning models, including classical, convolutional, and recurrent neural networks, were analyzed. The accuracy of the predictions was compared using two error metrics: root mean square error and mean absolute error.
更多查看译文
关键词
Solar irradiance prediction,prediction horizon,forecast,data acquisition frequencies,deep learning,renewable energy forecasting
AI 理解论文
溯源树
样例
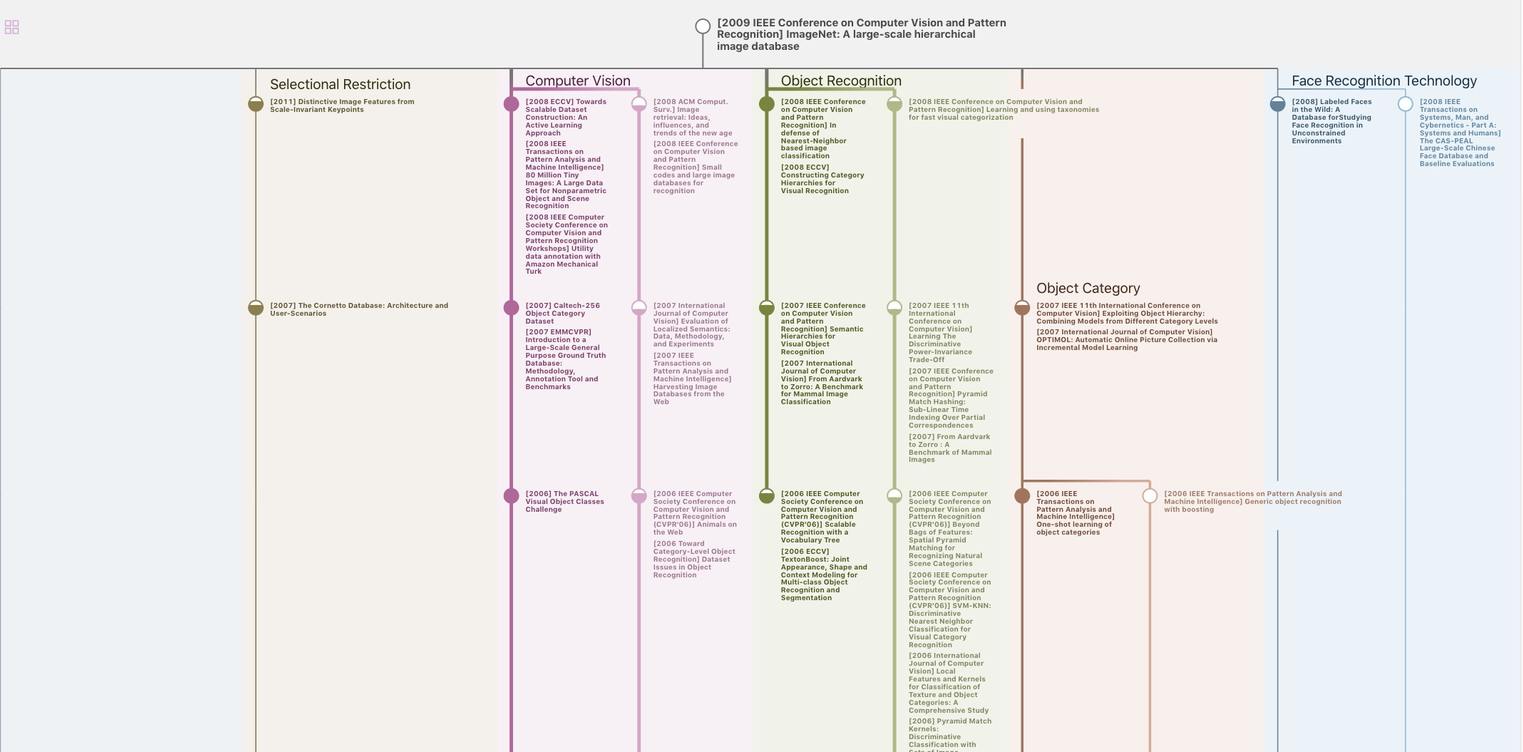
生成溯源树,研究论文发展脉络
Chat Paper
正在生成论文摘要