Machine Learning Models for Depression Detection Using the Concept of Perceived Control
ADVANCES IN COMPUTATIONAL INTELLIGENCE, IWANN 2023, PT II(2023)
摘要
In this paper, machine learning techniques are used to detect and predict the mental health status of individuals based on the concept of Perceived Control using a mobile app. Perceived control has long been established to have a strong link with an individual's mental health. Individuals with a high level of perceived control seem to have good mental health while those with low levels of perceived control usually suffer from depression, anxiety and stress. In the proposed method, an individual's measure of perceived control is solicited by allowing them to download and install an android app called the Judgement App. The users then participate in an experiment, where they perform a number of trials and make a judgement after 8 trials. The data generated is then analysed and used to train supervised machine learning models to predict whether an individual is suffering from depression or not. Data generated for internal and external perceived control were of both tabular and time-series types. The data is labelled by the subject's Beck Depressive Inventory (BDI-II) score, which is performed by the individual answering the 21-questions before the experiment begins. Due to the imbalanced nature of the data available, Synthetic Minority Oversampling Technique (SMOTE) and some of its variants were used to process the training data before being used to trainML algorithms. Simple evaluation criteria consisting of Precision, Recall, F1-score and overall model efficiency were used. The evaluation was completed by analyzing 274 samples from 140 participants. Out of the 274 samples, 53 were labelled as mildly depressed and 221 as non-depressed.
更多查看译文
关键词
Perceived Control,Internal Control,External Control,contingency,trial,judgement,depression
AI 理解论文
溯源树
样例
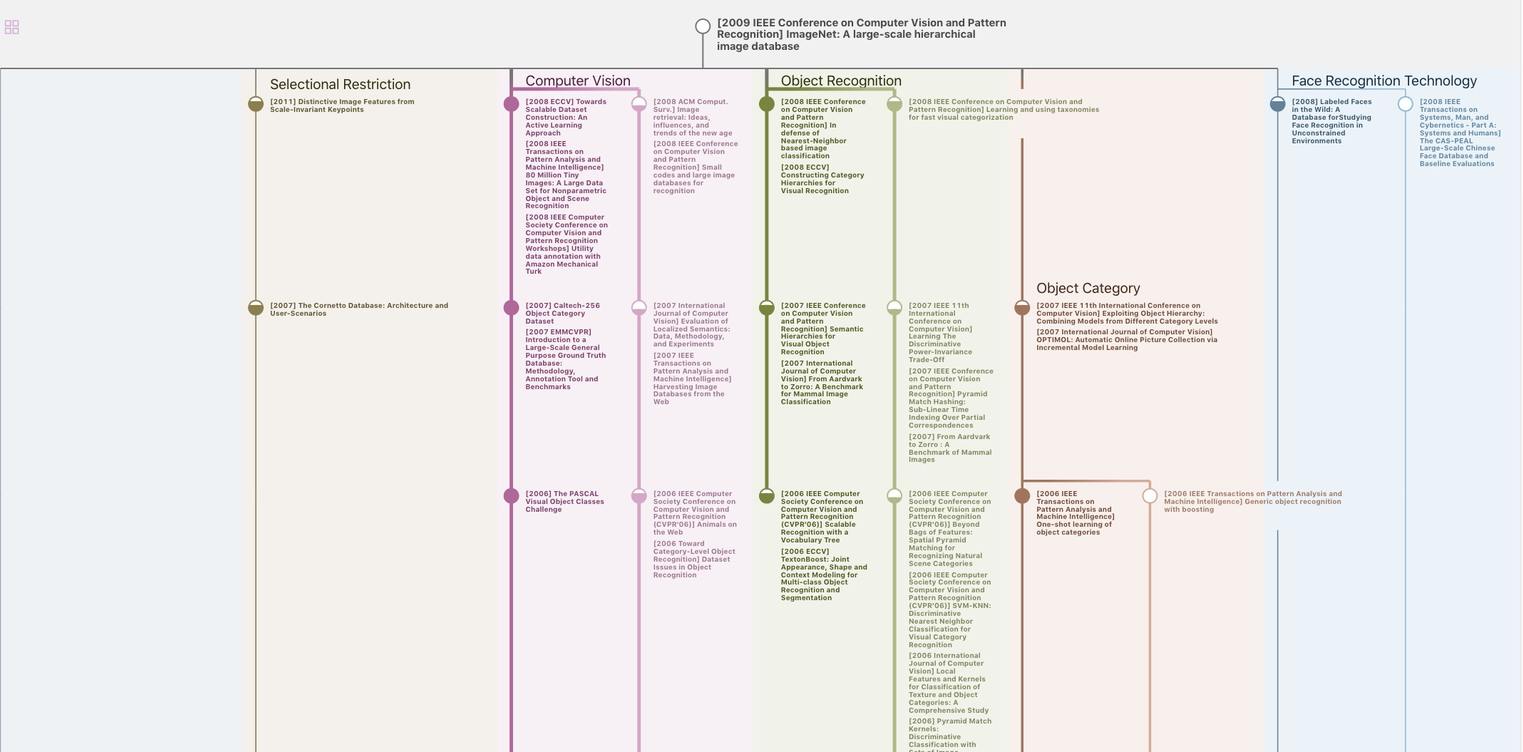
生成溯源树,研究论文发展脉络
Chat Paper
正在生成论文摘要