Experience Adapter: Adapting Pre-trained Language Models for Continual Task Planning.
ICIRA (5)(2023)
摘要
In this paper, we investigate the challenge of Pre-trained Language Models (PLMs) for continual task planning. PLM-based planner is difficult to incorporate incremental experience without risking catastrophic forgetting or overwhelming the model parameters. Inspired by human cognition, we propose the Experience Adapter, a novel method that avoids the need for model re-training or fine-tuning. The adapter continually collects experiences externally, including observation memory and human feedback, represented in memory graph and rules. Using these, the adapter directs task planning and corrects behavior not aligning with human expectations. Our method, not relying on the planner’s inherent structure, pairs easily with various foundational planning methods. In experiments on everyday tasks within the VirtualHome environment, we show that our approach significantly improves task success rate from 47% to 64%. This non-invasive method fits seamlessly within existing model-serving pipelines without altering the model training.
更多查看译文
关键词
language models,planning,task,experience adapter,pre-trained
AI 理解论文
溯源树
样例
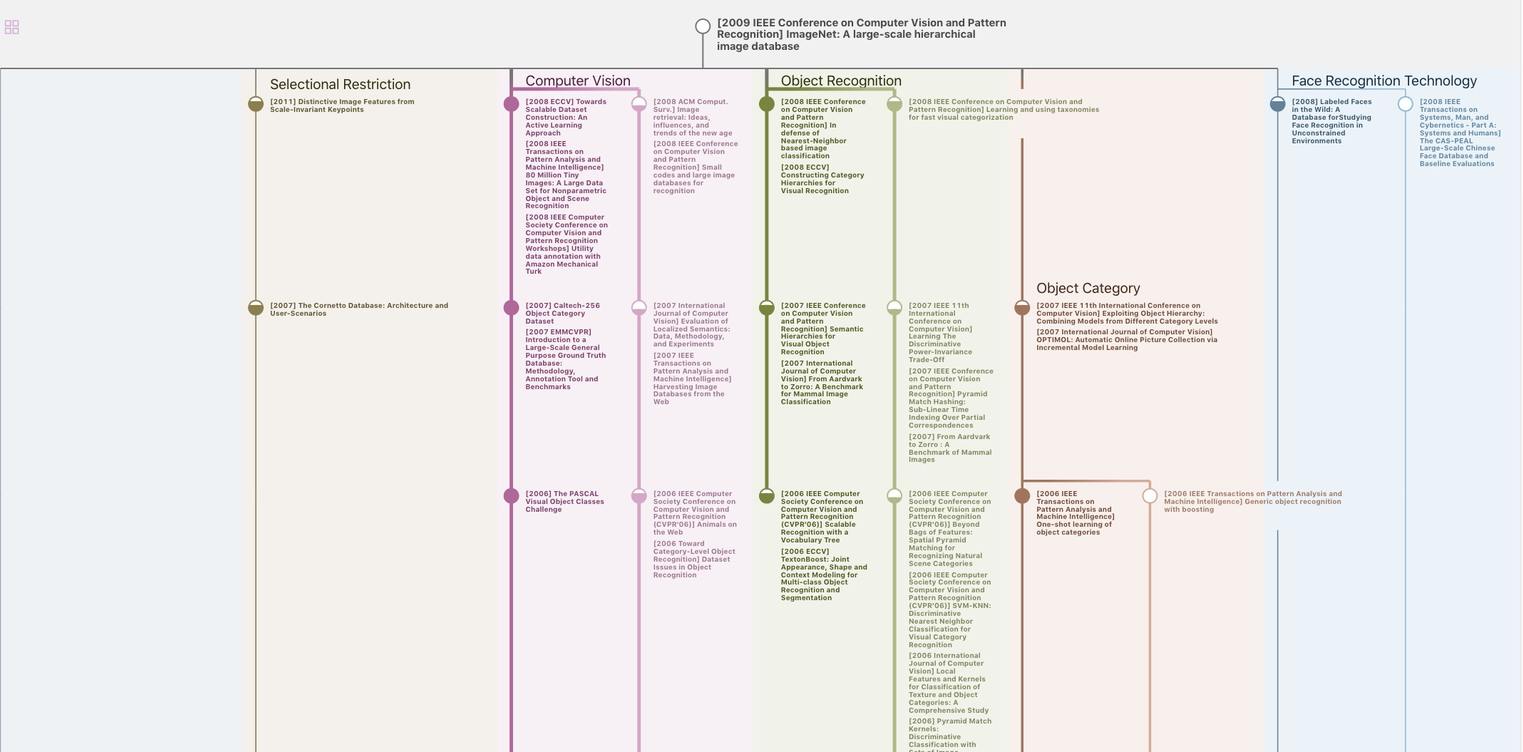
生成溯源树,研究论文发展脉络
Chat Paper
正在生成论文摘要