Demonstration Shaped Reward Machine for Robot Assembly Reinforcement Learning Tasks.
ICIRA (5)(2023)
摘要
Reinforcement Learning has been proven to be successfully applied in robotic manipulation tasks, but it has been confronted with problems about interacting with the dynamic environment and generating high-level policies. Existing reward functions include sparse and shaped reward, and other way to view the reward functions as black boxes and then discover the best strategies by interacting with the environment. In this paper, a reward machine with demonstration definition for robot assembly is presented, which is a finite state machine that supports generating the specified reward function from the demonstration data and displays the reward function structure. By dividing the task demonstration data, extracting the key points of the task and defining the state of the robot, a reward function based on state migration is generated. The robot chooses the best reward according to the current state, which is conducive to using the reward structure to improve the sample efficiency and the quality of the result strategy. In this paper, we focus on the robot assembly reinforcement learning task. The structure of the proposed method is used to carry out experiments in the robot simulator. We compare the design methods with different reward functions and it proves that the proposed method can effectively improve the training efficiency of robot reinforcement learning.
更多查看译文
关键词
demonstration shaped reward machine,robot,assembly
AI 理解论文
溯源树
样例
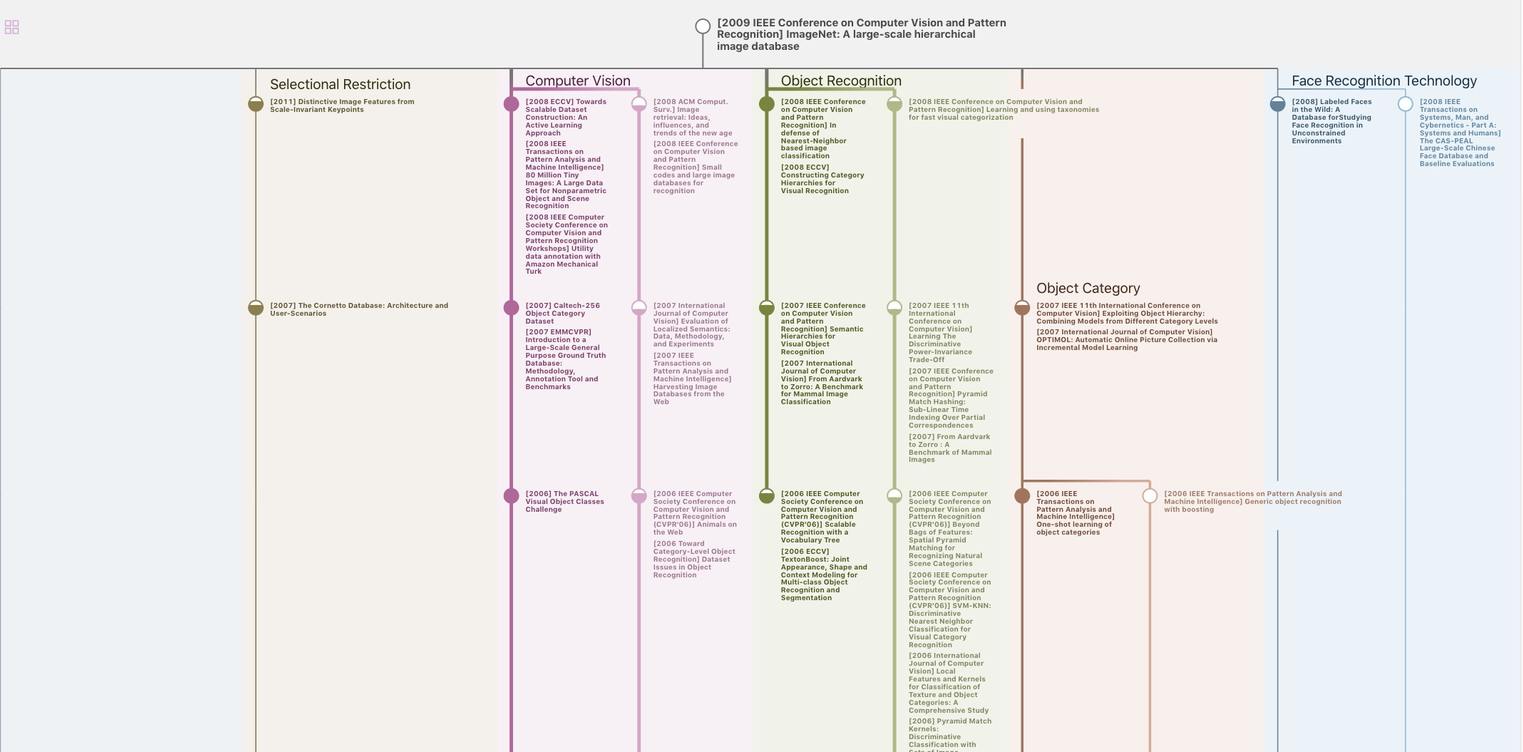
生成溯源树,研究论文发展脉络
Chat Paper
正在生成论文摘要