GPDCCL: Cross-Domain Named Entity Recognition with Span-Based Domain Confusion Contrastive Learning.
ICPCSEE (2)(2023)
摘要
The goal of cross-domain named entity recognition is to transfer models learned from labelled source domain data to unlabelled or lightly labelled target domain datasets. This paper discusses how to adapt a cross-domain sentiment analysis model to the field of named entity recognition, as the sentiment analysis model is more relevant to the tasks and data characteristics of named entity recognition. Most previous classification methods were based on a tokenwise approach, and this paper introduces entity boundary information to prevent the model from being affected by a large number of nonentity labels. Specifically, adversarial training is used to enable the model to learn domain-confusing knowledge, and contrastive learning is used to reduce domain shift problems. The entity boundary information is transformed into a global boundary matrix representing sentence-level target labels, enabling the model to learn explicit span boundary information. Experimental results demonstrate that this method achieves good performance compared to multiple cross-domain named entity recognition models on the SciTech dataset. Ablation experiments reveal that the method of introducing entity boundary information significantly improves KL divergence and contrastive learning.
更多查看译文
关键词
cross-domain,span-based
AI 理解论文
溯源树
样例
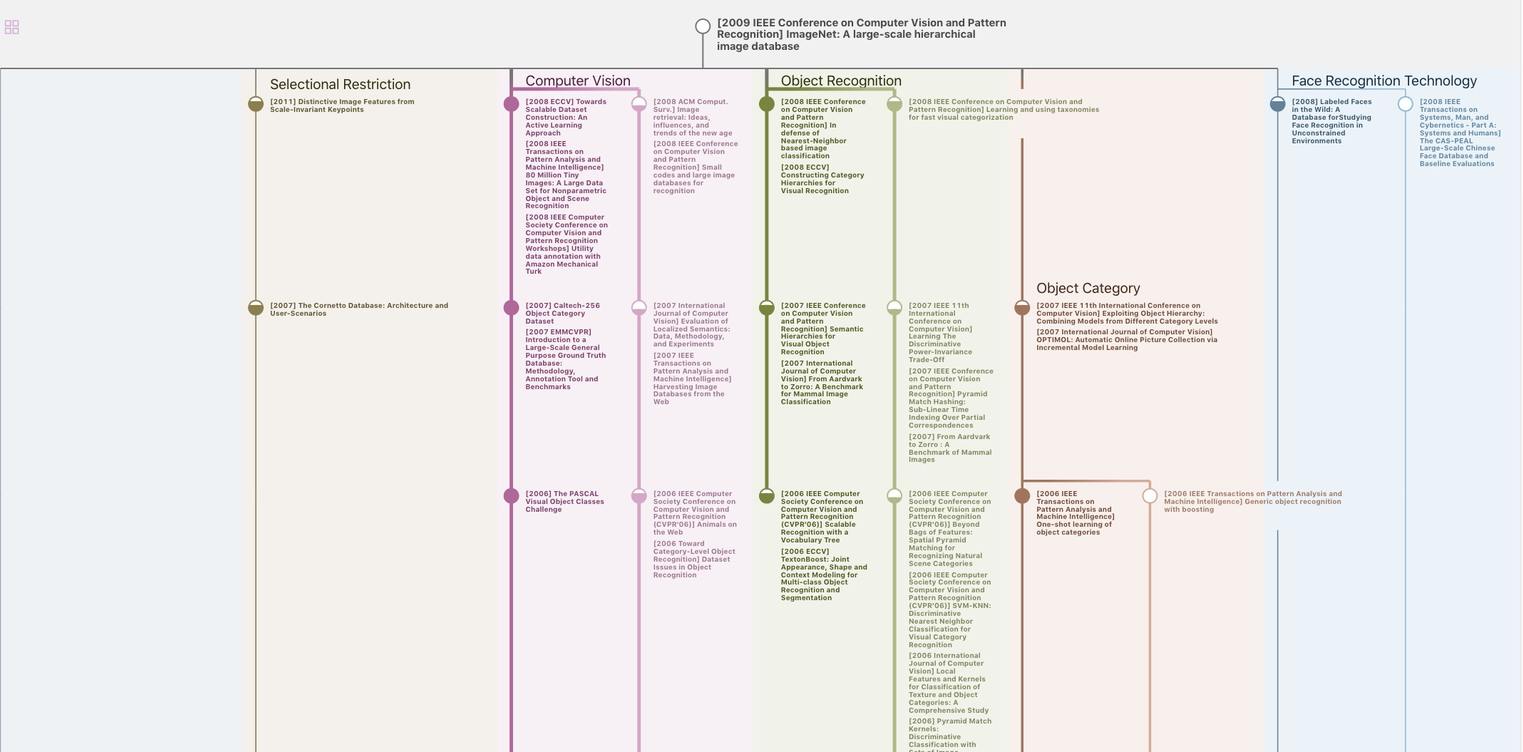
生成溯源树,研究论文发展脉络
Chat Paper
正在生成论文摘要