Combating Label Ambiguity with Smooth Learning for Facial Expression Recognition.
ICIRA (2)(2023)
摘要
Accurately learning facial expression recognition (FER) features using convolutional neural networks (CNNs) is a non-trivial task because of the presence of significant intra-class variability and inter-class similarity as well as the ambiguity of the expressions themselves. Deep metric learning (DML) methods, such as joint central loss and softmax loss optimization, have been adopted by many FER methods to improve the discriminative power of expression recognition models. However, equal supervision of all features with DML methods may include irrelevant features, which ultimately reduces the generalization ability of the learning algorithm. We propose the Attentive Cascaded Network (ACD) method to enhance the discriminative power by adaptively selecting a subset of important feature elements. The proposed ACD integrates multiple feature extractors with smooth center loss to extract to discriminative features. The estimated weights adapt to the sparse representation of central loss to selectively achieve intra-class compactness and inter-class separation of relevant information in the embedding space. The proposed ACD approach is superior compared to state-of-the-art
更多查看译文
关键词
smooth learning,label ambiguity,expression
AI 理解论文
溯源树
样例
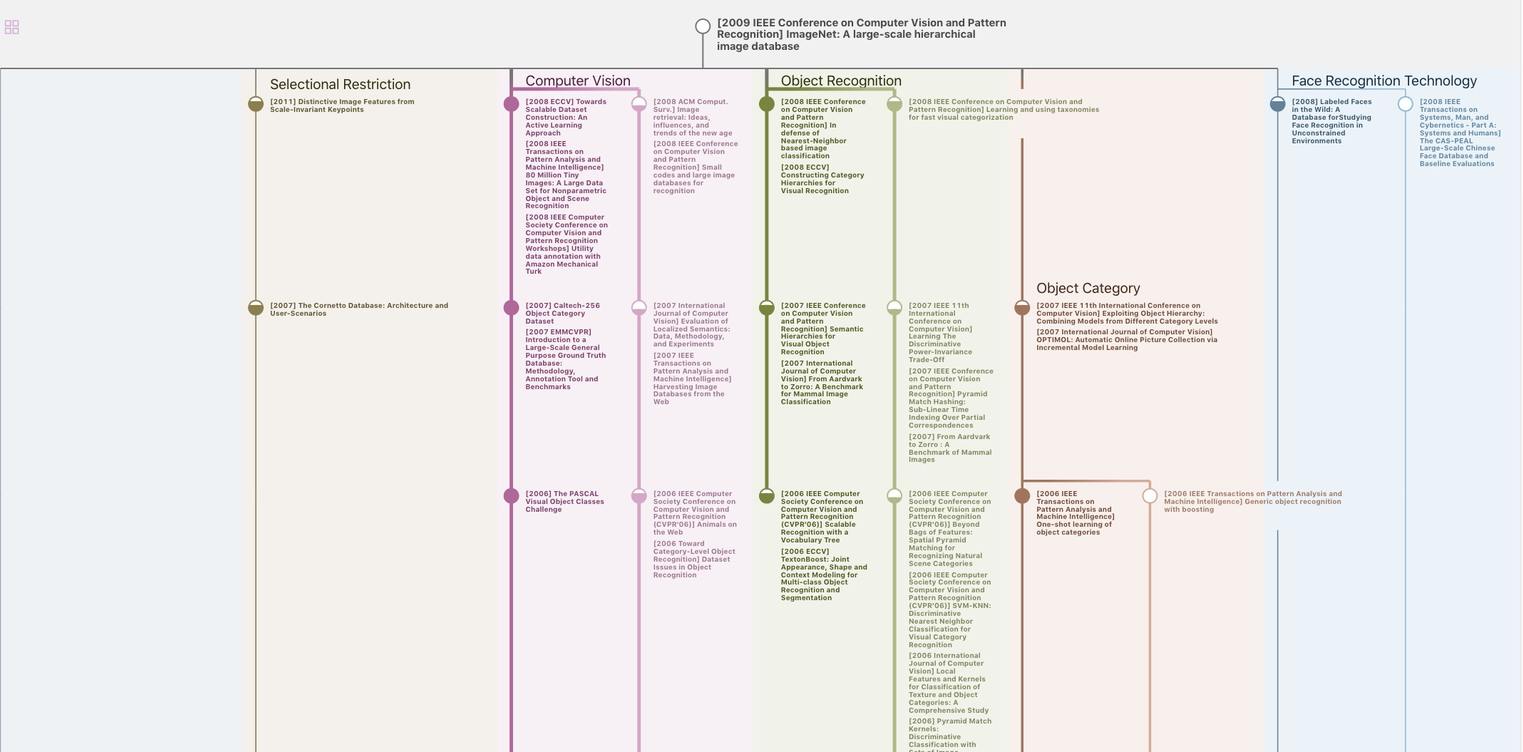
生成溯源树,研究论文发展脉络
Chat Paper
正在生成论文摘要