Study on Quantitative Precipitation Estimation and Model's Transfer Performance by Incorporating Dual Polarization Radar Variables.
ICIRA (4)(2023)
摘要
Short-time heavy precipitation belongs to a sudden and catastrophic convective weather. Accurate quantitative precipitation estimation is of great significance for preventing heavy precipitation disaster caused by convective systems. At present, quantitative precipitation estimation mostly relies on radar reflectivity (Ref) maps provided by the conventional Doppler radar, but the physical information that reflectivity can provide is quite limited. Dual-polarization radar can provide additional polarization variables, these variables have additional storm microphysical state and dynamic structure information, which helps to improve the accuracy of quantitative precipitation estimation. To this end, a neural network model incorporating multiple radar polarization variables is designed in this paper. We also introduce an attention mechanism module based on the reflectivity to combine the differential reflectivity (Zdr) and differential phase shift rate (Kdp) for inference constraints and result correction. The experimental results show that the model in this paper reduced the prediction RMSE by 11.49%−27.23% compared with the traditional Z-R relationship and by 7.51%−18.27% compared with the deep learning model QPENet. Meanwhile, this paper tested the transfer performance of the model, using data from Central China and South China respectively after training with data from the Central USA. The results show that the model performs better in Central China at the same latitude, which fully illustrates the obvious difference of convective system evolution mechanism in different latitudes.
更多查看译文
关键词
dual polarization radar variables,quantitative precipitation estimation,transfer performance
AI 理解论文
溯源树
样例
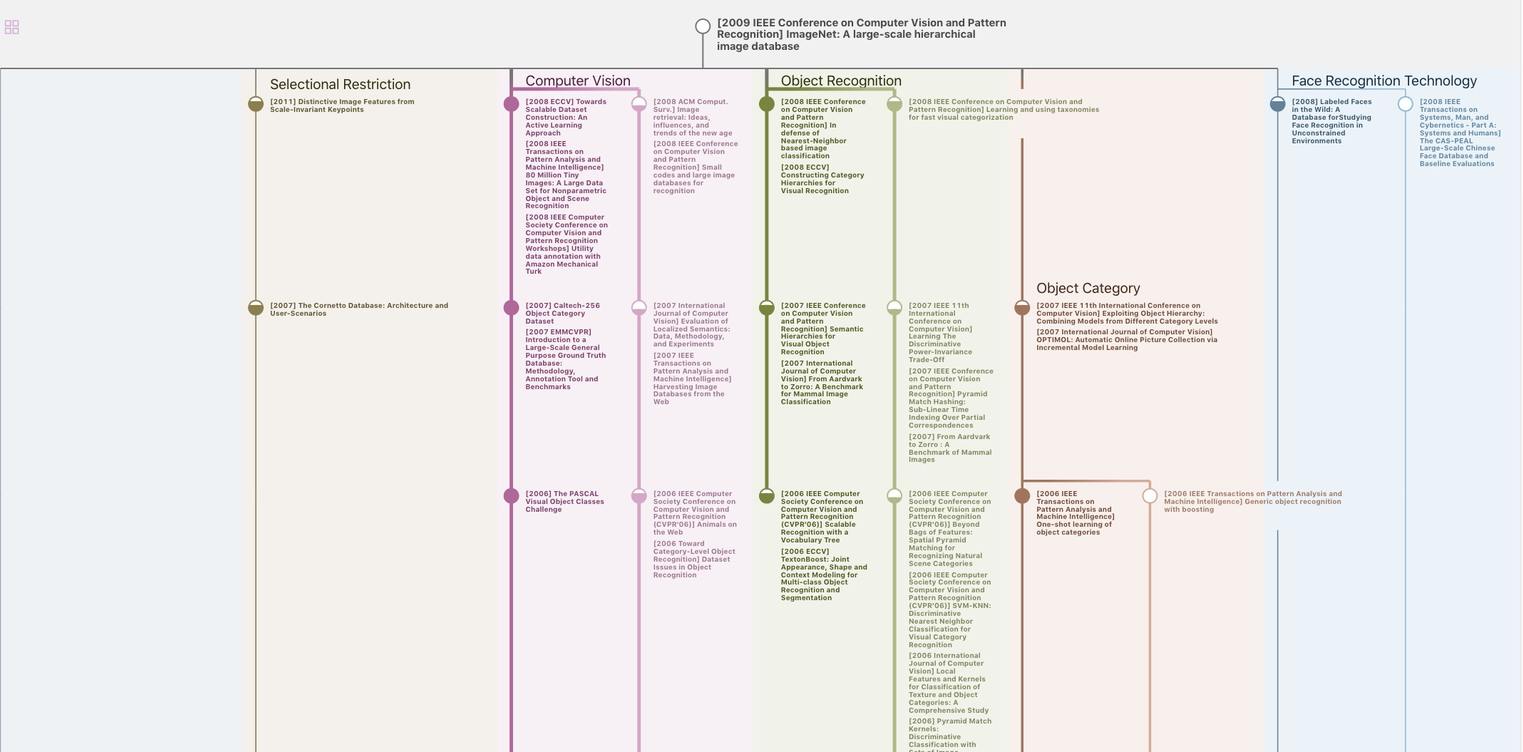
生成溯源树,研究论文发展脉络
Chat Paper
正在生成论文摘要