A Modified BiSeNet for Spinal Segmentation.
ICIRA (3)(2023)
摘要
Multi-class segmentation of vertebrae and intervertebral discs is of paramount importance for accurate diagnosis and treatment of various spinal diseases. However, achieving precise segmentation remains a challenging task. In this study, we propose a modified BiSeNet specifically designed for spine segmentation, aiming to address the limitations and improve the segmentation performance. Our motivation behind modifying BiSeNet lies in fully leveraging the strengths of both the context and spatial paths in feature extraction based on the BiSeNet. To enhance segmentation effectiveness, we fuse the two paths, which consist of a context extractor and a spatial extractor, using the feature fusion module (FFM) in BiSeNet. We utilize a U-shaped architecture for the context path, incorporating attention refinement modules (ARM) to leverage features from all encoder layers. Additionally, a specific residual structure is introduced to enhance the context extractor’s effectiveness. For the spatial path, we introduce a novel multi-scale convolution attention module based on the SegNext structure. These main contributions in our framework improve the segmentation effectiveness by capturing long-range relationships among vertebrae and intervertebral discs while considering inter-class similarity and intra-class variation. Experimental results on an MRI dataset comprising 172 subjects demonstrate impressive performance, with a mean Dice similarity coefficient of 82.365% across all spinal structures. These results indicate the considerable potential of our proposed method in aiding the diagnosis and treatment of spinal diseases.
更多查看译文
关键词
spinal segmentation,modified bisenet
AI 理解论文
溯源树
样例
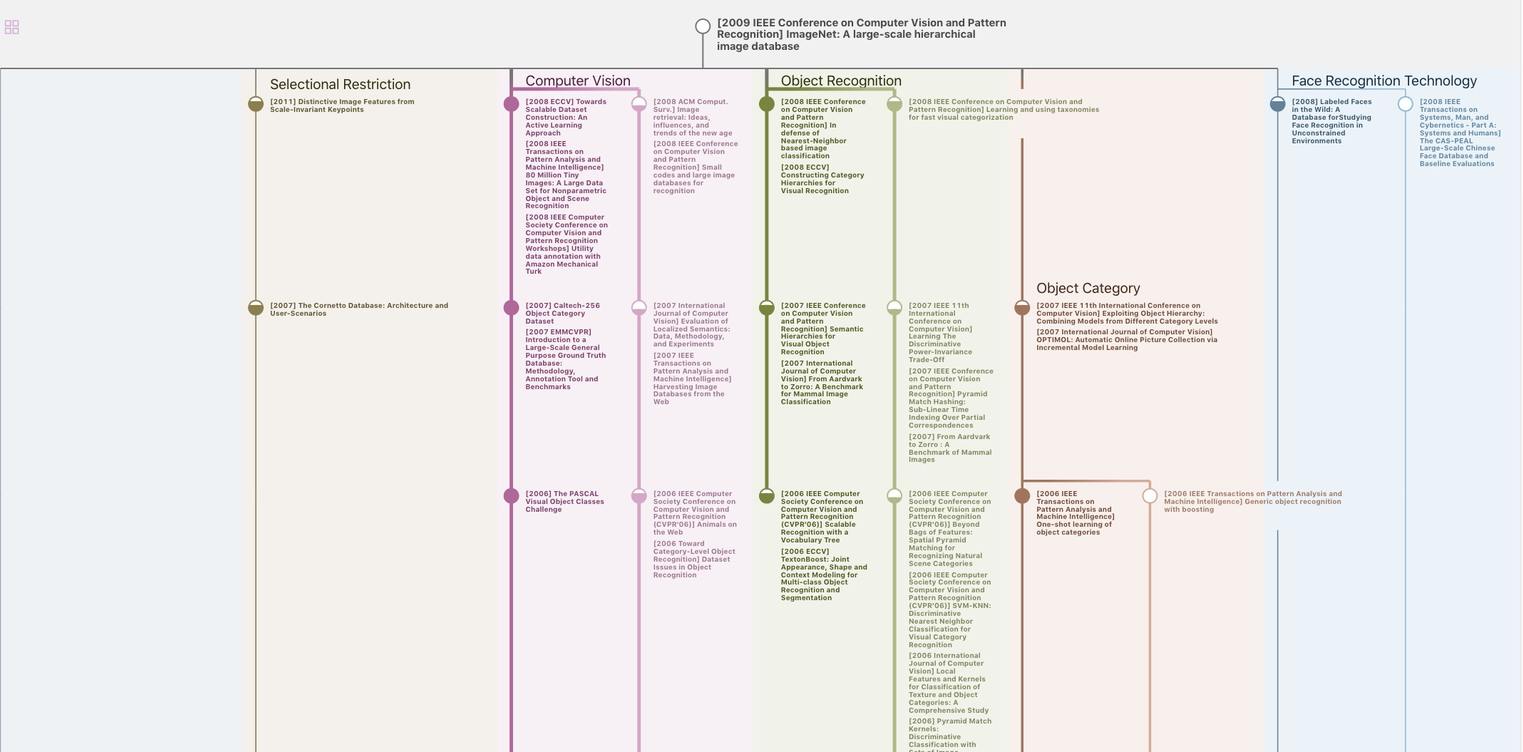
生成溯源树,研究论文发展脉络
Chat Paper
正在生成论文摘要