TLEF: Two-Layer Evolutionary Framework for t-Closeness Anonymization.
WISE(2023)
摘要
Data anonymization is a fundamental and practical privacy-preserving data publication (PPDP) method, while searching for the optimal anonymization scheme using traditional methods has been proven to be NP-hard. Some recent studies have introduced genetic algorithms (GA) into data anonymization to address this issue, revealing potential solutions. However, the discussions are restricted to a few privacy protection models and evolutionary algorithms (EAs). This paper extends this field by introducing differential evolution (DE) for the first time to optimize data anonymization schemes under the constraints of the t -closeness privacy model. To further enhance the algorithm’s performance, this paper designs a two-layer evolutionary framework (TLEF) that effectively explores optimal solutions by leveraging the unique properties of both GA and DE in a balanced manner. Experimental evaluations conducted on 16 test datasets highlight the advantages of DE in addressing optimal t -closeness anonymization and validate the effectiveness of the TLEF.
更多查看译文
关键词
two-layer,t-closeness
AI 理解论文
溯源树
样例
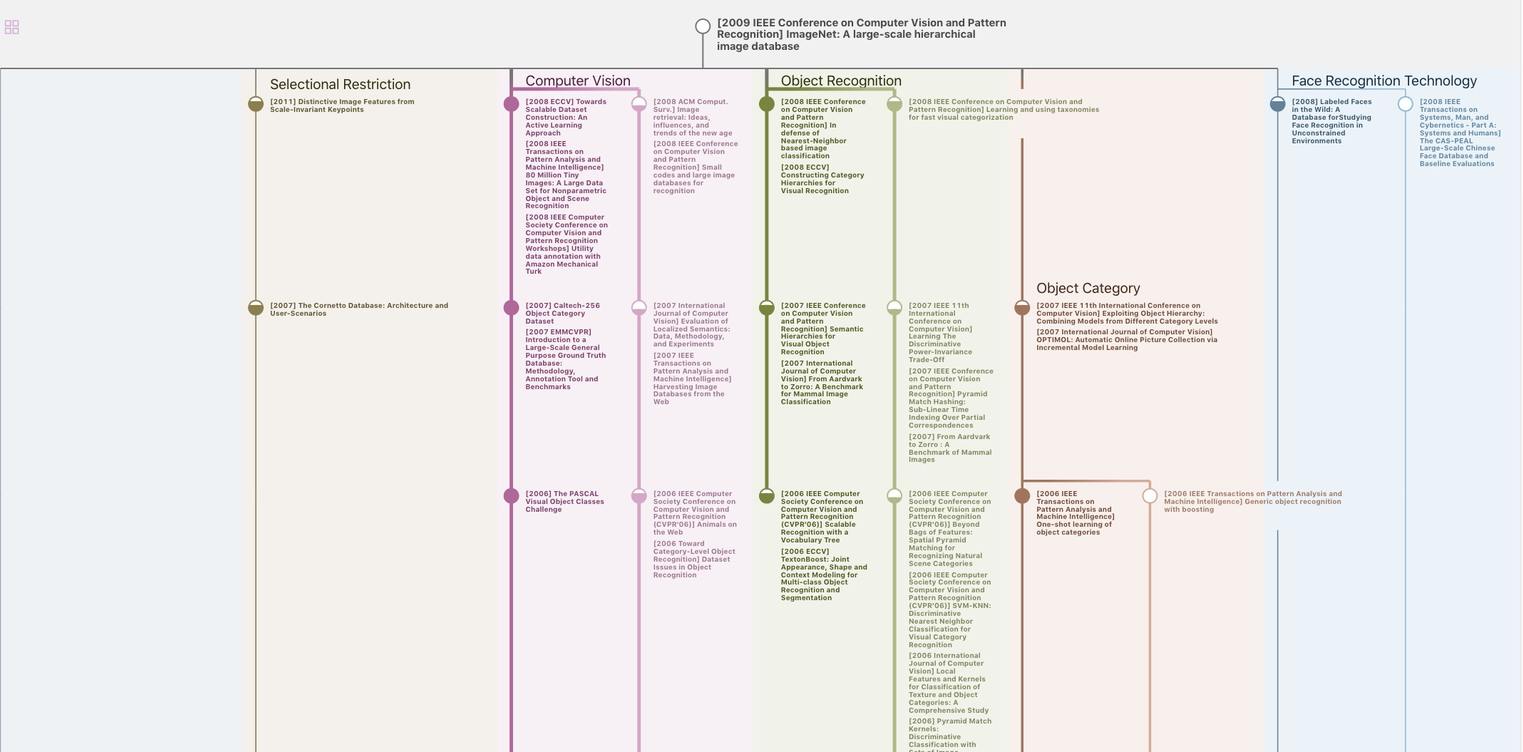
生成溯源树,研究论文发展脉络
Chat Paper
正在生成论文摘要