rT5: A Retrieval-Augmented Pre-trained Model for Ancient Chinese Entity Description Generation.
NLPCC (1)(2023)
摘要
Ancient Chinese, the natural language of ancient China, serves as the key to understanding and propagating Chinese rich history and civilization. However, to facilitate comprehension and education, human experts previously need to write modern language descriptions for special entities, such as persons and locations, out of ancient Chinese texts. This process requires specialized knowledge and can be time-consuming. To address these challenges, we propose a new task called Ancient Chinese Entity Description Generation (ACEDG), which aims to automatically generate modern language descriptions for ancient entities. To address ACEDG, we propose two expert-annotated datasets, XunZi and MengZi, each containing ancient Chinese texts, and some of them have been annotated with entities and their descriptions by human experts. To leverage both labeled and unlabeled texts, we propose a retrieval-augmented pre-trained model called rT5. Specifically, a pseudo-parallel corpus is constructed using retrieval techniques to augment the pre-training stage. Subsequently, the pre-trained model is fine-tuned on our high-quality human-annotated entity-description corpus. Our experimental results, evaluated using various metrics, demonstrate the effectiveness of our method. By combining retrieval techniques and pre-training, our approach significantly advances the state-of-the-art performance in the ACEDG task compared with strong pre-trained models.
更多查看译文
关键词
chinese,retrieval-augmented,pre-trained
AI 理解论文
溯源树
样例
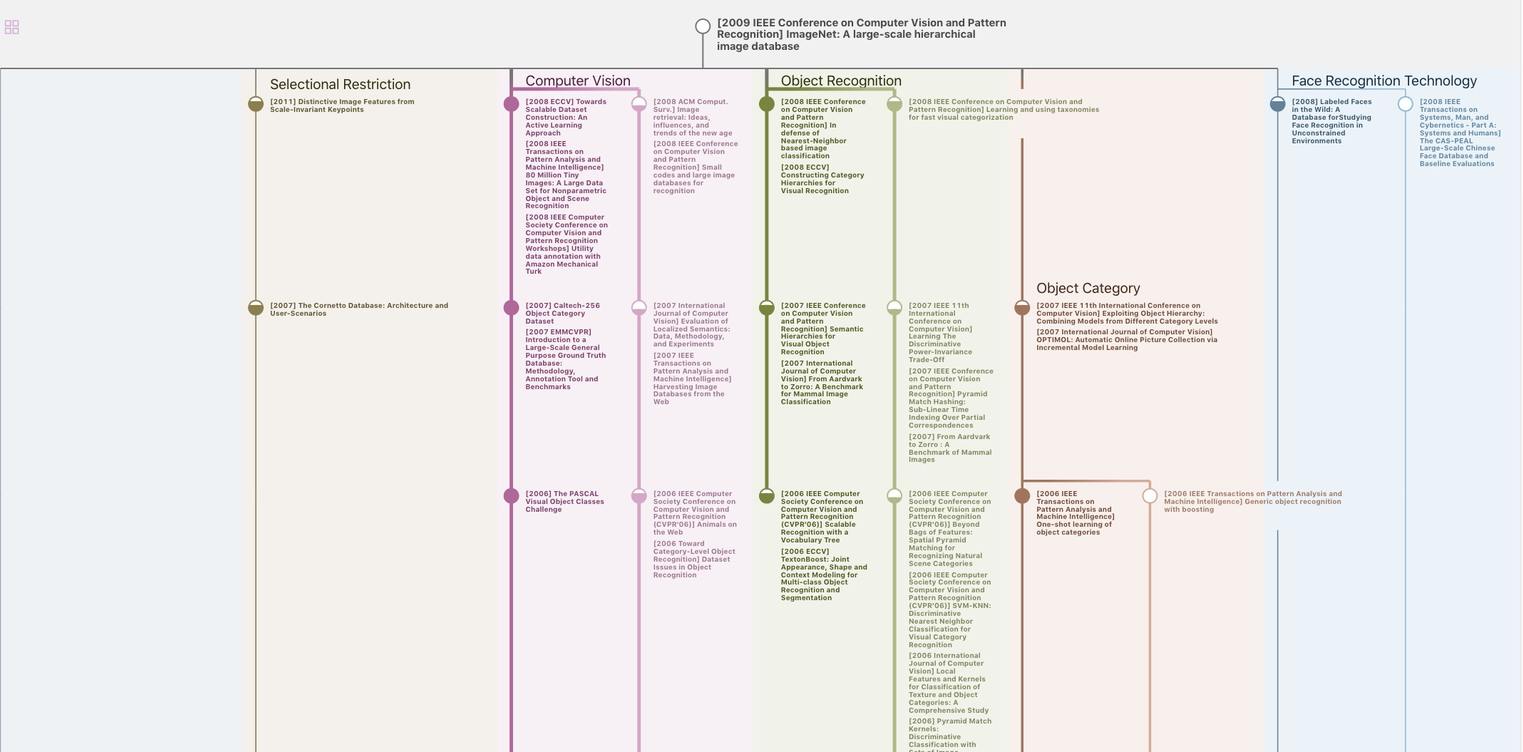
生成溯源树,研究论文发展脉络
Chat Paper
正在生成论文摘要